An End-to-End EEG Channel Selection Method with Residual Gumbel Softmax for Brain-Assisted Speech Enhancement.
IEEE International Conference on Acoustics, Speech, and Signal Processing(2024)
Abstract
Brain-assisted speech enhancement (SE) has gained an increasing attention recently, as electroencephalogram (EEG) measurements somehow reflect auditory attention clues. The design of an EEG cap with sparse channel distributions can save the hardware cost, setup time as well as algorithmic complexity, which can be done by EEG channel selection, as it was shown that the multichannel EEG signals are highly correlated and redundant. In this paper, we thus propose an end-to-end EEG channel selection method based on a weighted residual structure, called Residual Gumbel Selection (ResGS), for the neuro-steered SE task. The use of residual connections can lead to a more efficient and stable training procedure. The proposed ResGS consists of the weighted residual training and fine-tuning steps. Experimental results on a public dataset validate the efficacy of the proposed method in channel selection and show that a small subset of channels is enough to achieve a near-optimal performance.
MoreTranslated text
Key words
EEG channel selection,neuro-steered speech enhancement,Gumbel softmax function,multi-talker conditions
AI Read Science
Must-Reading Tree
Example
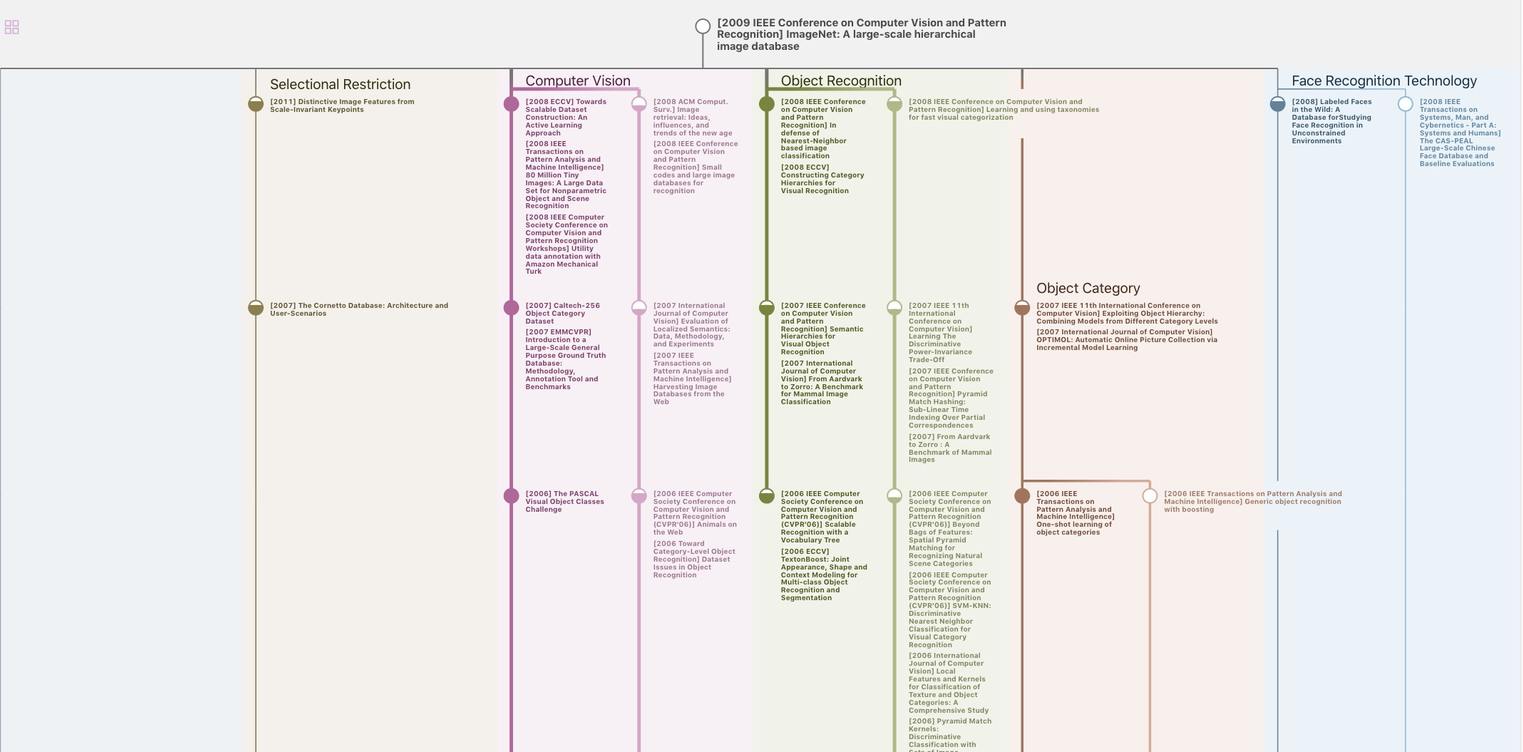
Generate MRT to find the research sequence of this paper
Chat Paper
Summary is being generated by the instructions you defined