AdaMER-CTC: Connectionist Temporal Classification with Adaptive Maximum Entropy Regularization for Automatic Speech Recognition
ICASSP 2024 - 2024 IEEE International Conference on Acoustics, Speech and Signal Processing (ICASSP)(2024)
摘要
In Automatic Speech Recognition (ASR) systems, a recurring obstacle is the
generation of narrowly focused output distributions. This phenomenon emerges as
a side effect of Connectionist Temporal Classification (CTC), a robust sequence
learning tool that utilizes dynamic programming for sequence mapping. While
earlier efforts have tried to combine the CTC loss with an entropy maximization
regularization term to mitigate this issue, they employed a constant weighting
term on the regularization during the training, which we find may not be
optimal. In this work, we introduce Adaptive Maximum Entropy Regularization
(AdaMER), a technique that can modulate the impact of entropy regularization
throughout the training process. This approach not only refines ASR model
training but ensures that as training proceeds, predictions display the desired
model confidence.
更多查看译文
关键词
Automatic Speech Recognition,Connectionist Temporal Classification,Entropy Maximization
AI 理解论文
溯源树
样例
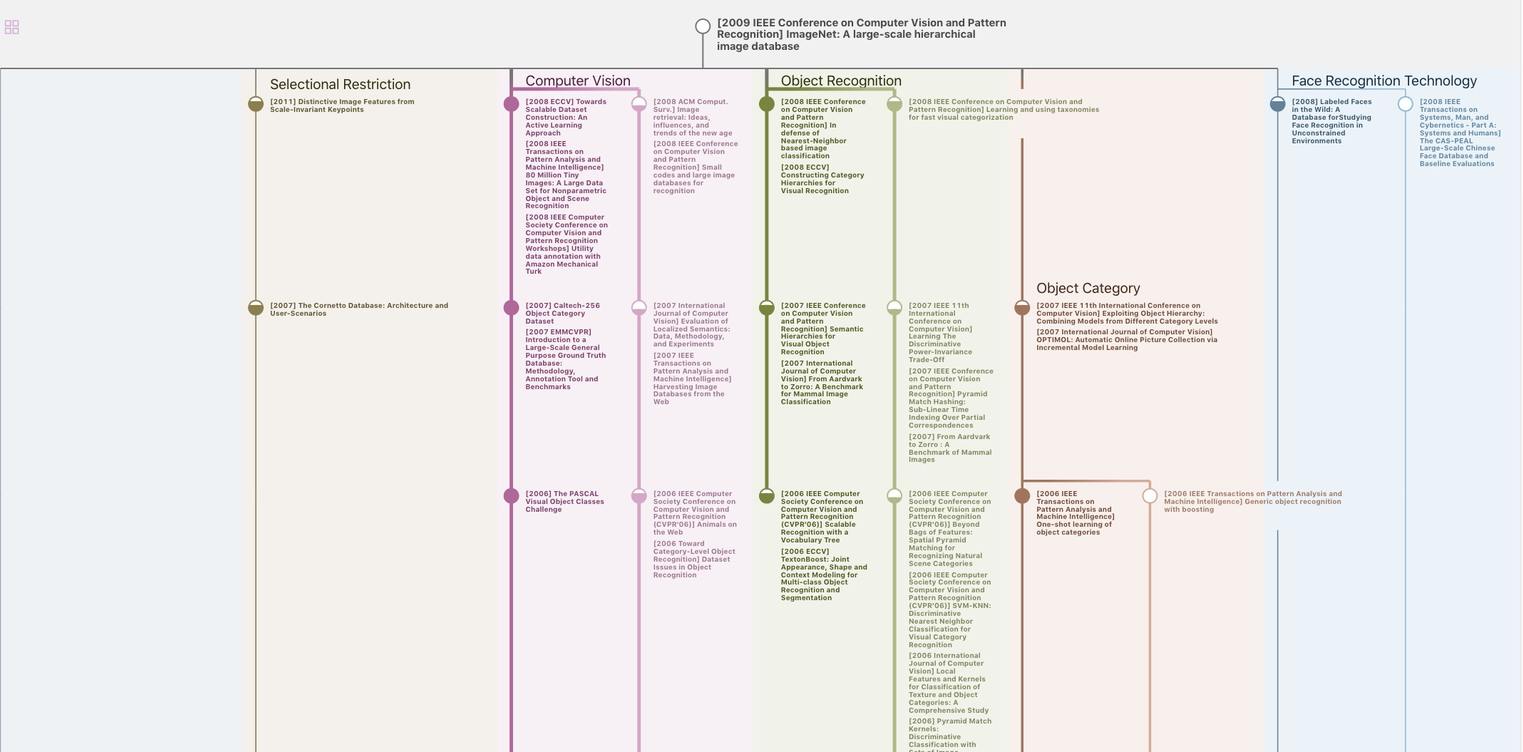
生成溯源树,研究论文发展脉络
Chat Paper
正在生成论文摘要