Implementation of Adaptive Partial Discharge De-noising in Resource-limited Embedded Systems via Efficient Time-frequency Matrix Factorization
IEEE Transactions on Instrumentation and Measurement(2024)
摘要
As low-cost Internet-of-Thing-based PD monitors for medium-voltage apparatuses in distribution power systems increase, developing an effective PD de-noising algorithm is crucial to improve their robustness to on-site noise. Yet, de-noising PD signals in the monitoring devices is challenging primarily due to three critical reasons, i.e., high-level field noises, uncertain PD waveform, and limited computing resources. This work describes an adaptive and efficient PD de-noising algorithm based on the improved spectral decomposition of the noisy PD signal. PD pulses are accurately extracted from the noisy signal by selecting the dominant components via a low-rank singular value decomposition of the time-frequency spectrogram of the signal, thus reducing the size of the involved matrices and the computational complexity. The performance of the proposed de-noising algorithm is first demonstrated on a synthetic PD signal and compared with state-of-the-art alternatives implemented on three embedded systems commonly used for PD monitoring. Finally, the strength and the effectiveness of the proposed approach are further validated on experimental data based on the measurement of Internet-of-Thing-based PD monitors for 35-kV switchgears.
更多查看译文
关键词
Partial discharge de-noising,embedded systems,short-time Fourier transform,randomized singular value decomposition,kurtosis criteria
AI 理解论文
溯源树
样例
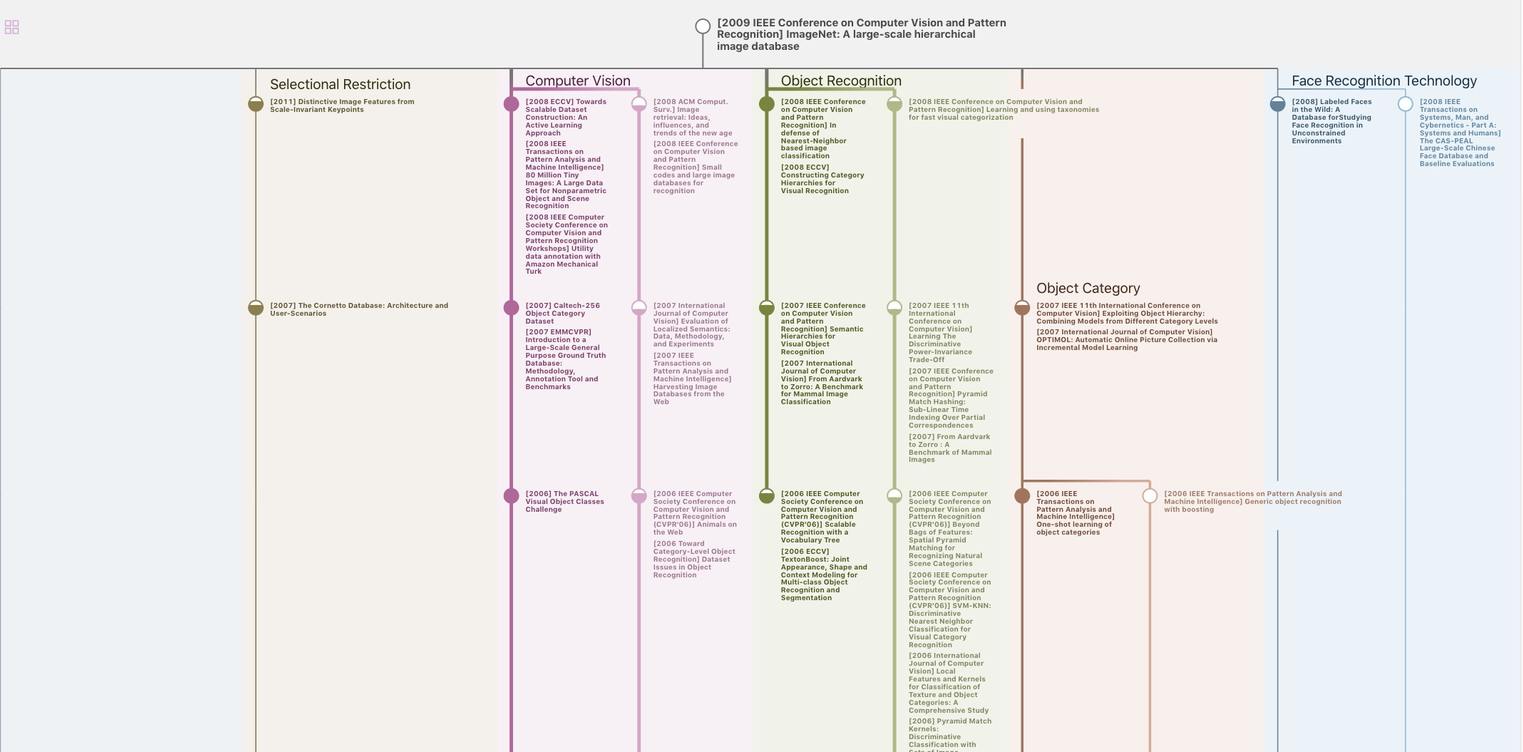
生成溯源树,研究论文发展脉络
Chat Paper
正在生成论文摘要