Tensor Graph Decomposition for Temporal Networks
ICASSP 2024 - 2024 IEEE International Conference on Acoustics, Speech and Signal Processing (ICASSP)(2024)
摘要
Temporal networks arise due to certain dynamics influencing their connections or due to the change in interactions between the nodes themselves, as seen for example in social networks. Such evolution can be algebraically represented by a three-way tensor, which lends itself to using tensor decompositions to study the underpinning factors driving the network evolution. Low rank tensor decompositions have been used for temporal networks but mostly with a focus on downstream tasks and have been seldom used to study the temporal network itself. Here, we use the tensor decomposition to identify a limited number of key mode graphs that can explain the temporal network, and which linear combination can represent its evolution. For this, we put for a novel graph-based tensor decomposition approach where we impose a graph structure on the two modes of the tensor and a smoothness on the temporal dimension. We use these mode graphs to investigate the temporal network and corroborate their usability for network reconstruction and link prediction.
更多查看译文
关键词
Dynamic networks,tensors,adjacency matrix,alternating optimization
AI 理解论文
溯源树
样例
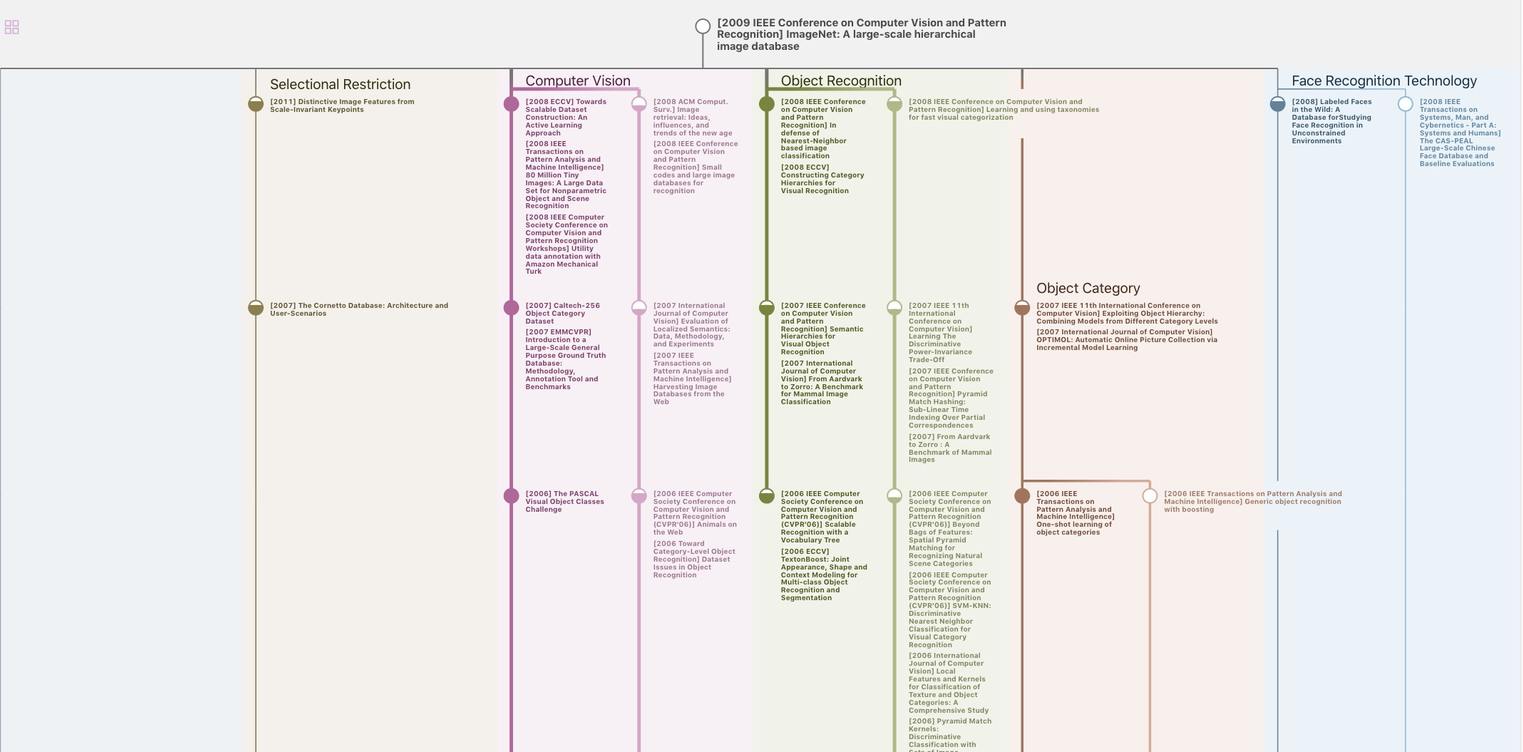
生成溯源树,研究论文发展脉络
Chat Paper
正在生成论文摘要