Machine Learning Predictive Model: Prioritizing Expenditures in Public Transportation Based on Transportation Emissions
CONSTRUCTION RESEARCH CONGRESS 2024: ADVANCED TECHNOLOGIES, AUTOMATION, AND COMPUTER APPLICATIONS IN CONSTRUCTION(2024)
Abstract
The United States (US) is the largest Greenhouse Gas (GHG) emitter globally, with its transportation sector being a major carbon dioxide contributor. This is a matter of significant concern, given the impact of these emissions on climate change. Prioritizing emissions-reducing transportation projects is crucial to address this problem. Currently, such policies are generally discussed at the national level. However, since individual states have varying infrastructure and priorities, this paper proposes studying carbon dioxide emissions at the state level of granularity. To achieve this, ensemble methods such as Random Forest, Extremely Randomized Trees, AdaBoost, and Gradient Boosted Decision Trees, along with a meta-estimator, are used to predict statewide carbon dioxide emissions using variables related to statewide transportation systems. Based on this analysis, we suggest that states should discourage car dependency in a way that is feasible for local characteristics, as well as promote public transit to reduce carbon dioxide emissions.
MoreTranslated text
AI Read Science
Must-Reading Tree
Example
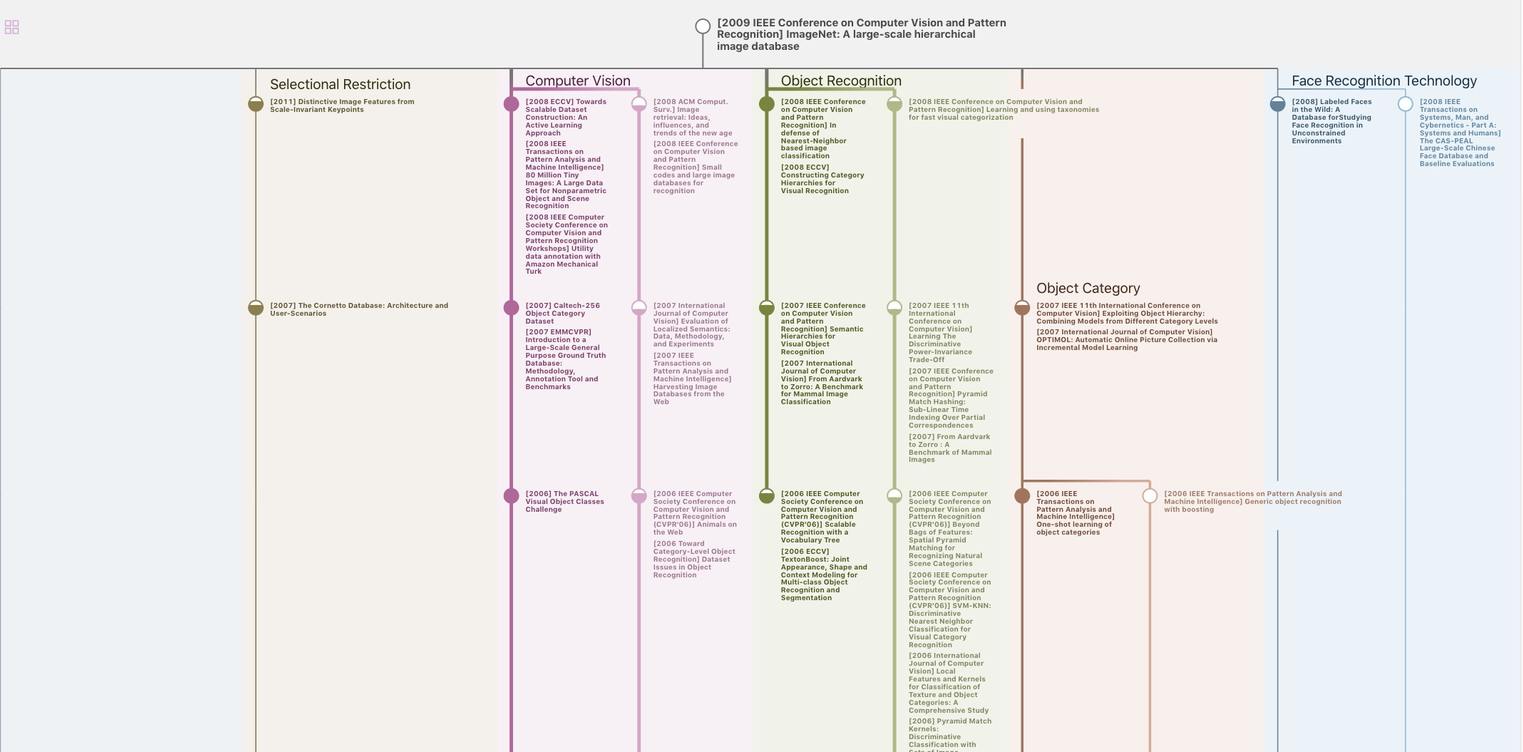
Generate MRT to find the research sequence of this paper
Chat Paper
Summary is being generated by the instructions you defined