DIB-X: Formulating Explainability Principles for a Self-Explainable Model Through Information Theoretic Learning.
IEEE International Conference on Acoustics, Speech, and Signal Processing(2024)
摘要
The recent development of self-explainable deep learning approaches has focused on integrating well-defined explainability principles into learning process, with the goal of achieving these principles through optimization. In this work, we propose DIB-X, a self-explainable deep learning approach for image data, which adheres to the principles of minimal, sufficient, and interactive explanations. The minimality and sufficiency principles are rooted from the trade-off relationship within the information bottleneck framework. Distinctly, DIB-X directly quantifies the minimality principle using the recently proposed matrix-based Rényi’s α-order entropy functional, circumventing the need for variational approximation and distributional assumption. The interactivity principle is realized by incorporating existing domain knowledge as prior explanations, fostering explanations that align with established domain understanding. Empirical results on MNIST and two marine environment monitoring datasets with different modalities reveal that our approach primarily provides improved explainability with the added advantage of enhanced classification performance.
更多查看译文
关键词
Explainability principle,self-explainable deep learning,information bottleneck,Rényi’s α-order entropy functional
AI 理解论文
溯源树
样例
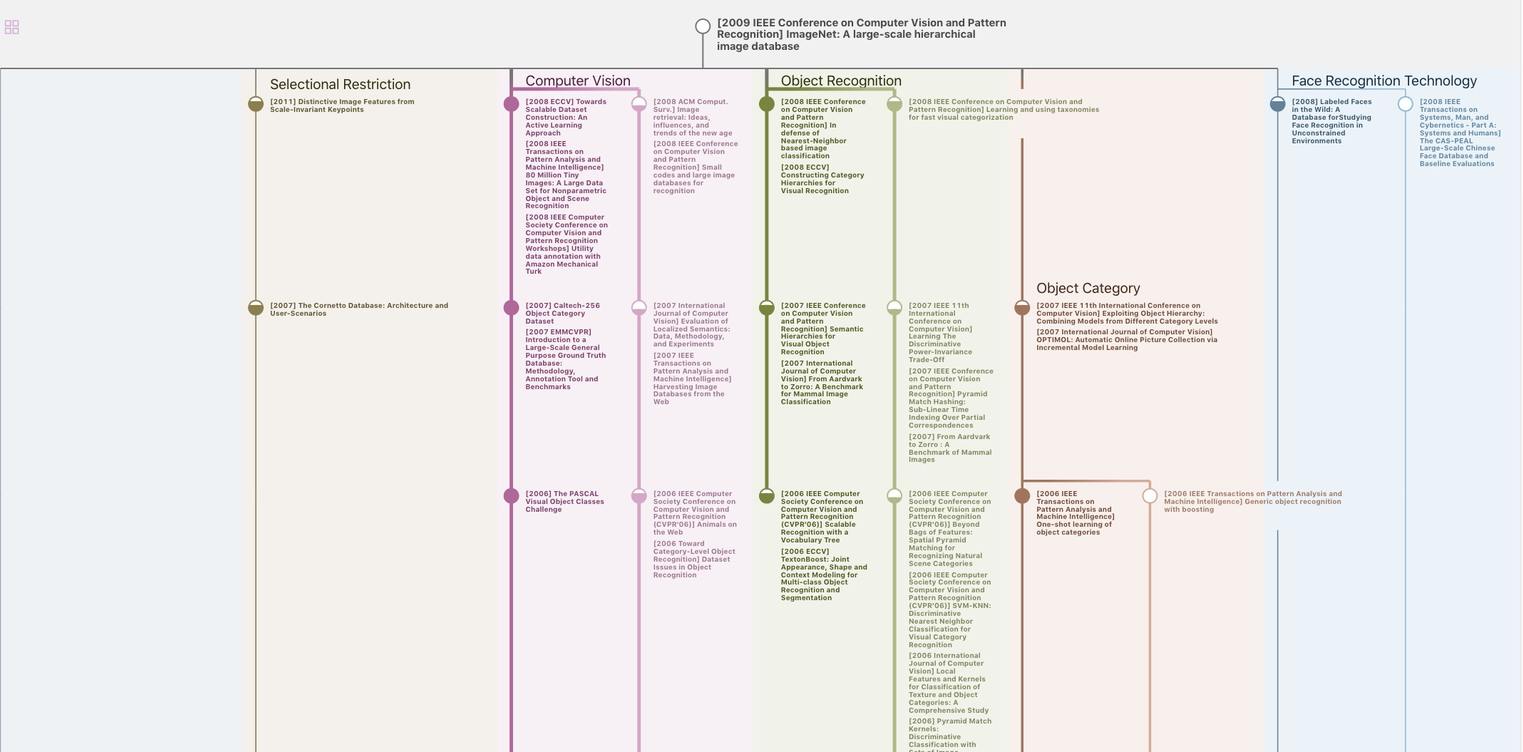
生成溯源树,研究论文发展脉络
Chat Paper
正在生成论文摘要