A Reconstruction-Based Feature Adaptation for Anomaly Detection with Self-Supervised Multi-Scale Aggregation
ICASSP 2024 - 2024 IEEE International Conference on Acoustics, Speech and Signal Processing (ICASSP)(2024)
摘要
Most previous embedding-based methods for anomaly detection directly utilize the visual features extracted from pretrained CNN network. However, there usually exists a gap of domain between pretrained data and target data in anomaly detection. To alleviate this discrepancy, we introduce ReconFA in this paper, a self-supervised domain adaptation method for anomaly detection: firstly, we design a self-supervised multi-scale features fusion method with multi-scale aggregation module (MSAM) to enhance interaction of multi-level outputs of pretrained CNN. Secondly, we train an encoder to adapt the features extracted from pretrained CNN to target domain by a feature-reconstruction task. Meanwhile, we use encoder to reduce the dimension of features to save space complexity. Extensive experiments show that our ReconFA method outperforms previous methods on MVTec AD datasets and on more challenging and sophisticated datasets MPDD.
更多查看译文
关键词
Anomaly detection,self-supervised learning,visual attention mechanism
AI 理解论文
溯源树
样例
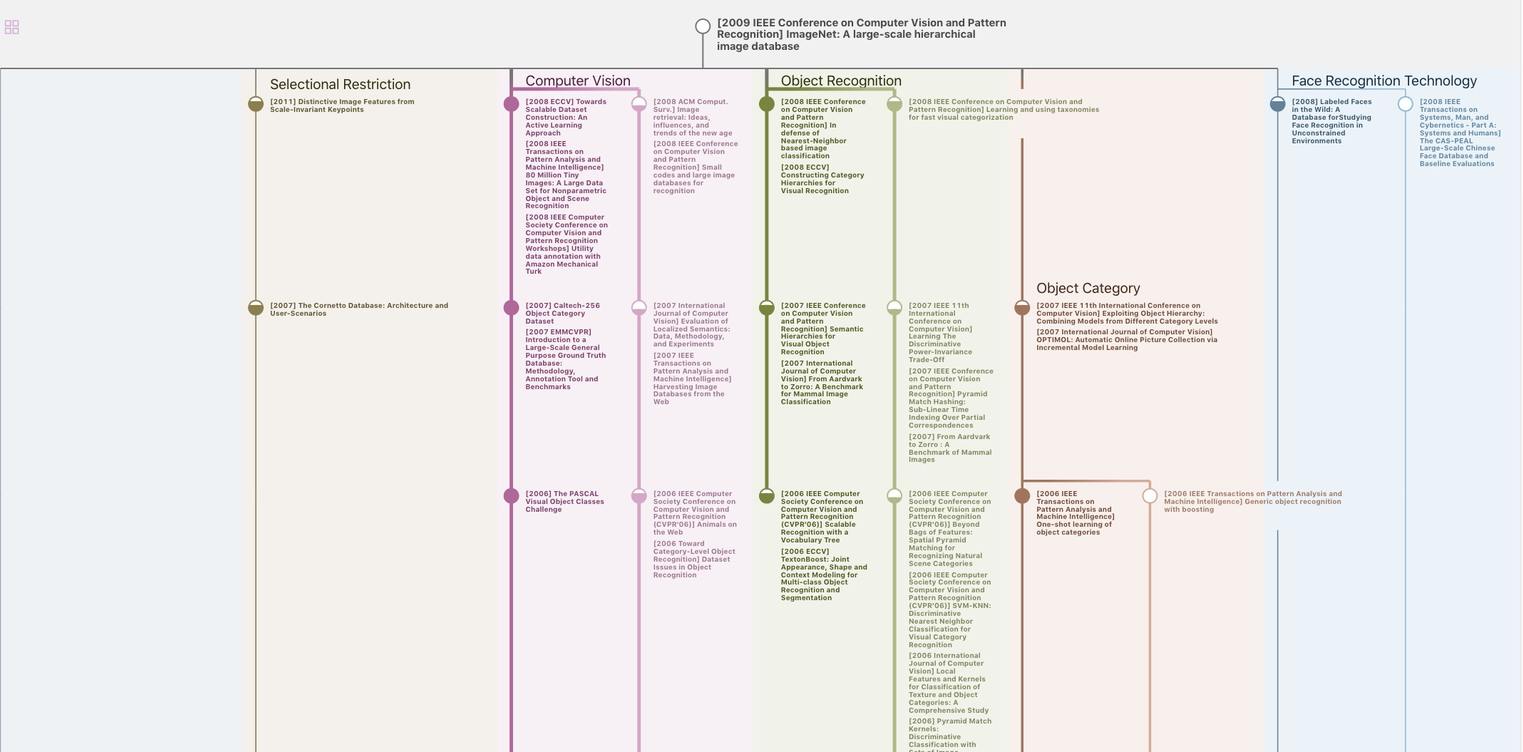
生成溯源树,研究论文发展脉络
Chat Paper
正在生成论文摘要