Improving Continual Learning of Acoustic Scene Classification via Mutual Information Optimization
ICASSP 2024 - 2024 IEEE International Conference on Acoustics, Speech and Signal Processing (ICASSP)(2024)
摘要
Continual learning, which aims to incrementally accumulate knowledge, has been an increasingly significant but challenging research topic for deep models that are prone to catastrophic forgetting. In this paper, we propose a novel replay-based continual learning approach in the context of class-incremental learning in acoustic scene classification, to classify audio recordings into an expanding set of classes that characterize the acoustic scenes. Our approach is improving both the modeling and memory selection mechanism via mutual information optimization in continual learning. By regarding incremental classes of acoustic scenes as different tasks, our model is expected to learn both task-agnostic and task-specific knowledge by replaying representative and informative samples. This optimization also enables the model to utilize past knowledge effectively and learn from new information during continual learning. We demonstrate that our approach has a superior performance compared to existing methods on multiple datasets and continual learning evaluation metrics.
更多查看译文
关键词
Continual learning,acoustic scene classification
AI 理解论文
溯源树
样例
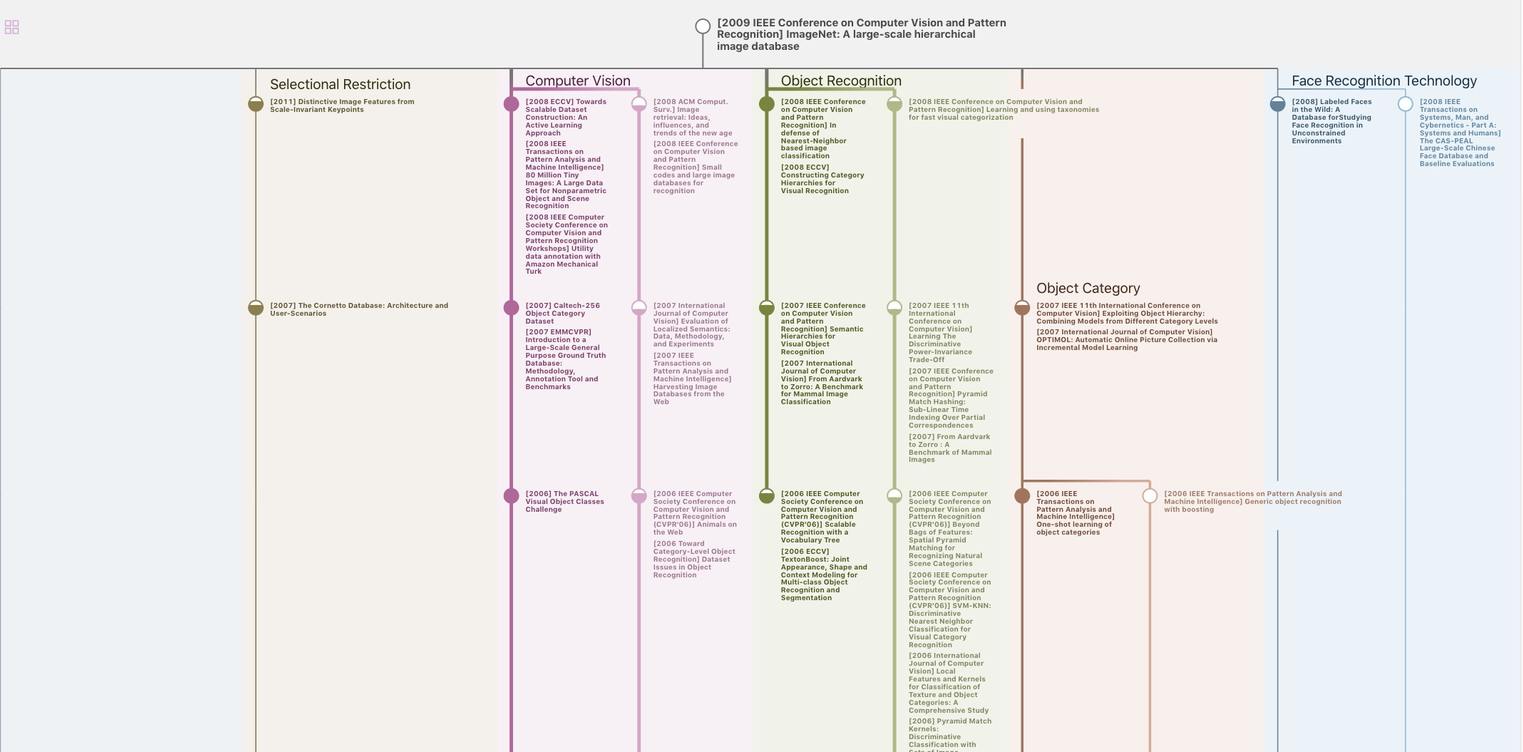
生成溯源树,研究论文发展脉络
Chat Paper
正在生成论文摘要