Maskstr: Guide Scene Text Recognition Models with Masking
ICASSP 2024 - 2024 IEEE International Conference on Acoustics, Speech and Signal Processing (ICASSP)(2024)
摘要
Text recognition in information loss scenarios like blurriness, occlusion, and perspective distortion is challenging in real-world applications. To enhance robustness, some studies use extra unlabeled data for encoder pretraining. Others focus on improving decoder context reasoning. However, pretraining methods require abundant unlabeled data and high computing resources, while decoder-based approaches risk over-correction. In this paper, we propose MaskSTR, a dual-branch training framework for STR models, using patch masking to simulate information loss. MaskSTR guides visual representation learning, improving robustness to information loss conditions without extra data or training stages. Furthermore, we introduce Block Masking, a novel and straightforward mask generation method, for further performance enhancement. Experiments demonstrate MaskSTR’s effectiveness across CTC, attention, and Transformer decoding methods, achieving significant performance gains and setting new state-of-the-art results.
更多查看译文
关键词
Scene text recognition,OCR,computer vision
AI 理解论文
溯源树
样例
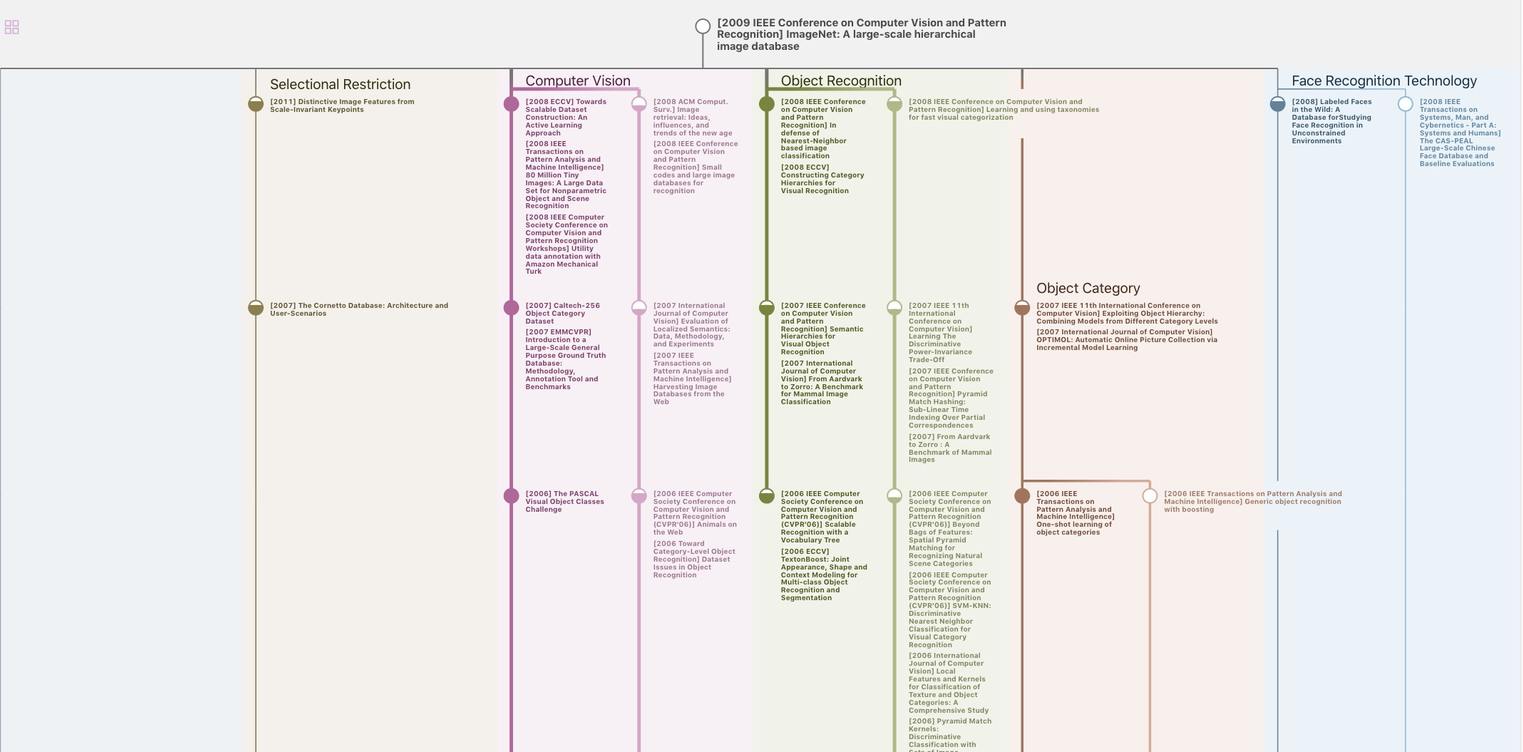
生成溯源树,研究论文发展脉络
Chat Paper
正在生成论文摘要