End-to-End Speech Translation with Mutual Knowledge Distillation
ICASSP 2024 - 2024 IEEE International Conference on Acoustics, Speech and Signal Processing (ICASSP)(2024)
摘要
Multi-task learning (MTL) is widely used to improve end-to-end speech translation (ST), which implicitly transfer knowledge from auxiliary automatic speech recognition (ASR) and/or machine translation (MT) to ST through shared modules. In this study, we find that triple-task MTL (ST+MT+ASR) suffers from a knowledge transfer limitation that leads to performance stagnation compared with dual-task MTL (ST+MT or ST+ASR). To address this issue, we propose a simple yet effective method, ST-MKD (Speech Translation with Mutual Knowledge Distillation). In ST-MKD, we employ a mutual knowledge distillation framework to mutually enhance dual-task MTL models with different knowledge bases, and explore regularization to maintain the consistency of the task representations. Experiments on the ST benchmark dataset MuST-C show that ST-MKD significantly outperforms strong MTL baseline and achieves state-of-the-art performance under three speech pre-training settings. Further analyses confirm that our approach effectively overcomes the knowledge transfer limitation of triple-task MTL.
更多查看译文
关键词
Speech translation,Mutual knowledge distillation,Multi-task learning
AI 理解论文
溯源树
样例
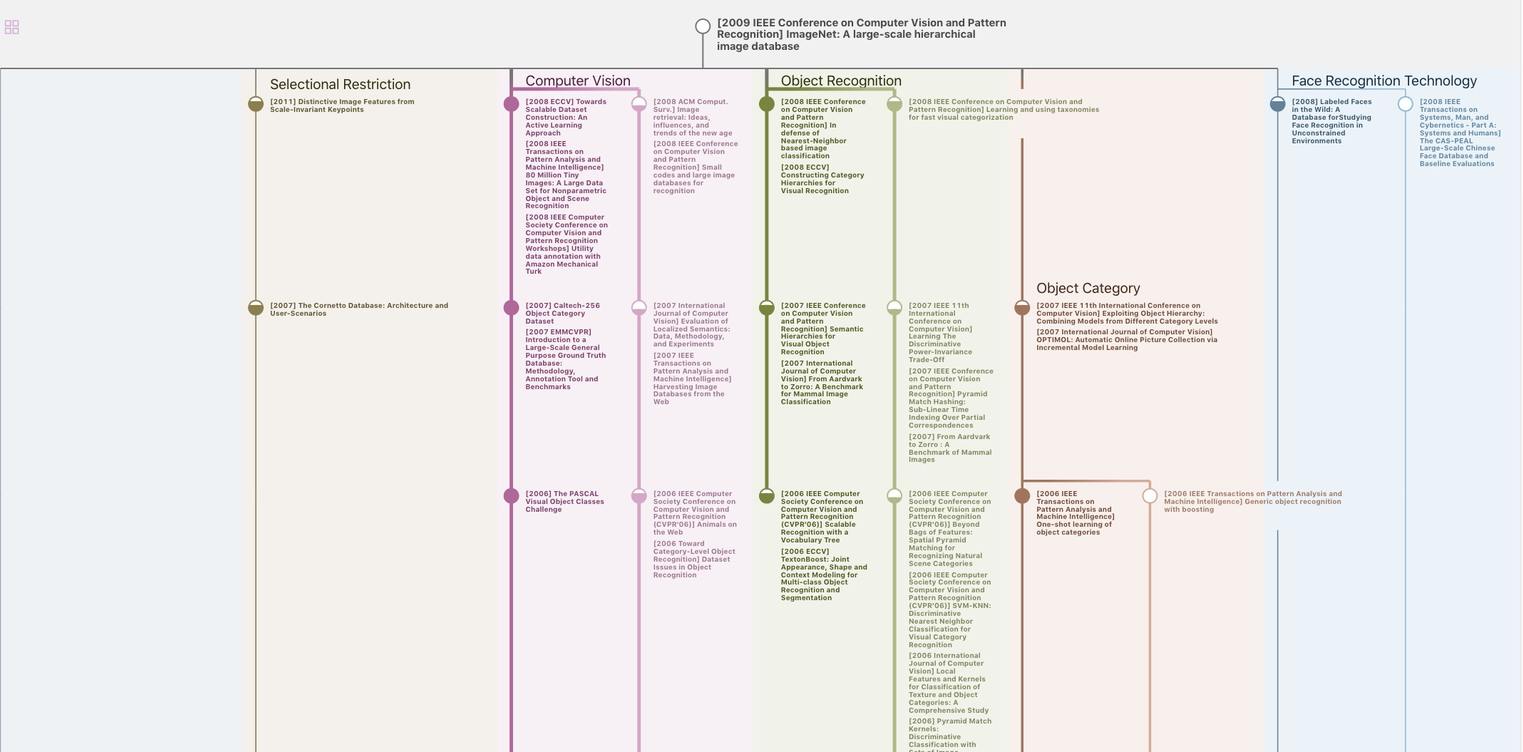
生成溯源树,研究论文发展脉络
Chat Paper
正在生成论文摘要