CoQ:AN Empirical Framework for Multi-hop Question Answering Empowered by Large Language Models
ICASSP 2024 - 2024 IEEE International Conference on Acoustics, Speech and Signal Processing (ICASSP)(2024)
摘要
Prompt-based Large Language Models(LLMs) are surprisingly powerful in generating natural language reasoning steps or Chains of Thoughts(CoT) for multi-hop question answering(QA). However, LLMs struggle when they lack access to necessary knowledge or when the knowledge within their parameters is outdated. Additionally, LLMs that rely solely on CoT tend to generate hallucinations during the reasoning process. To address these dilemmas, we propose the Chain of Question (CoQ) framework, a novel multi-hop QA approach. This approach decomposes a complex original question into multiple sub-questions according to a CoT to retrieve knowledge from an external knowledge base. It then answers the question process based on the retrieved knowledge in accordance with a CoT. We design that each point of thought generated during the reasoning process be supported by the knowledge retrieved in the external knowledge base. Experiments show that CoQ is effective in reducing model hallucinations, leading to higher factual accuracy than CoT. On average, it reduces factual errors by 31% over CoT, and even by 38% on the two most commonly used models today.
更多查看译文
关键词
Large Language Models,Question Answering,CoT,Knowledge Bases
AI 理解论文
溯源树
样例
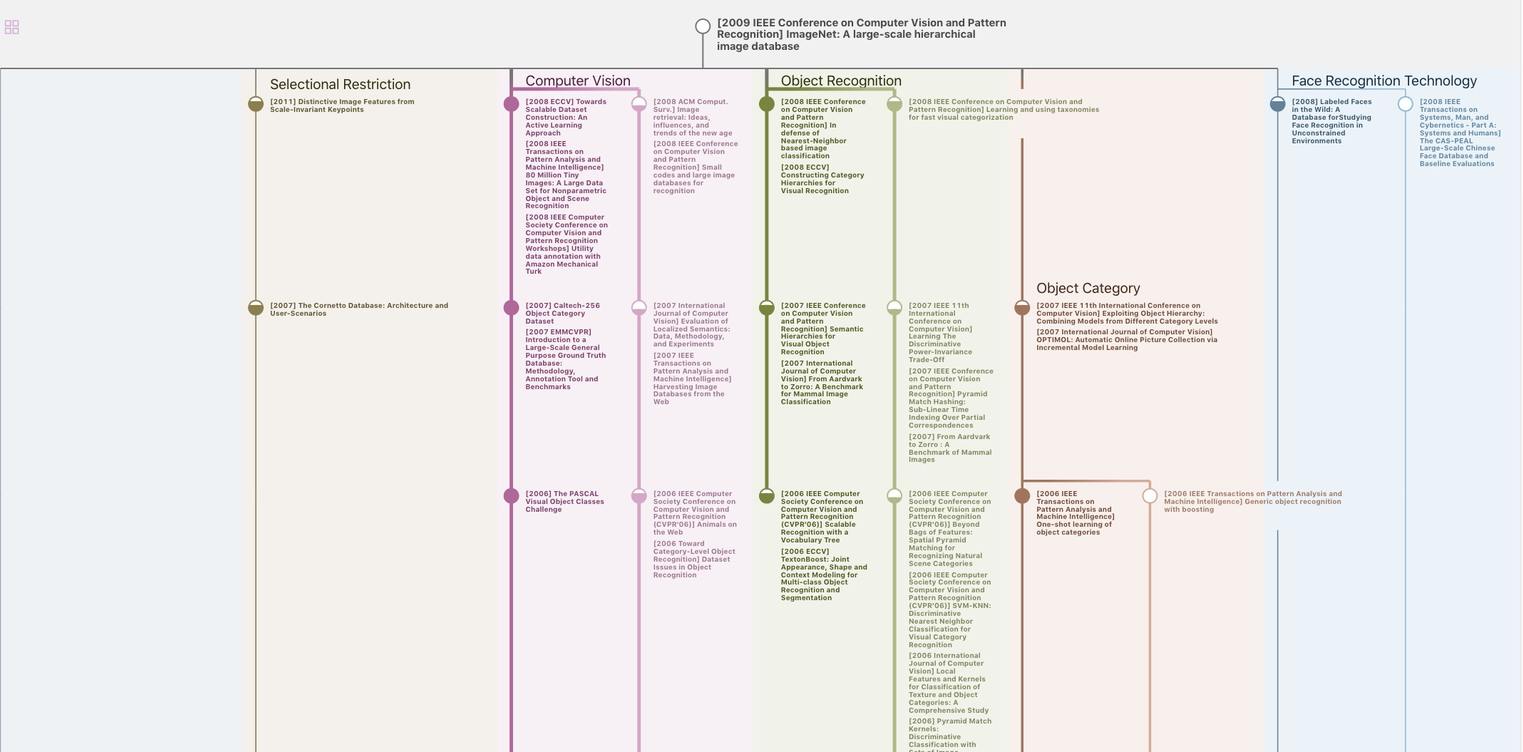
生成溯源树,研究论文发展脉络
Chat Paper
正在生成论文摘要