Digital Pathology Image Deblurring Via Local Focus Quality Assessment
ICASSP 2024 - 2024 IEEE International Conference on Acoustics, Speech and Signal Processing (ICASSP)(2024)
摘要
Defocus blurring is a prevalent problem in digital pathology imaging, which degrades the quality and accuracy of biomedical research and clinical diagnosis. Existing deblurring methods are mostly based on one-to-one image translation models, which require multiple models to handle different defocus focal planes. This limits their practical applicability and scalability. To address this challenge, we propose a novel method that uses local focus quality assessment (LFQA) maps as additional inputs to train a single deblurring model from multiple defocus focal planes. Our method consists of two stages: (1) generating LFQA maps by training a CNN to estimate the focal plane of small patches and applying it with sliding windows; (2) deblurring the image with our proposed Progressive Multi-scale Fusion Network (PMSF-Net) that takes the defocused image, its LFQA map, and the target LFQA map as inputs and outputs the deblurred image. The PMSF-Net is designed to fuse multi-scale features with gated attention modules in a progressive manner. Extensive experiments are conducted to demonstrate the superiority of our method compared with current deblurring state-of-the-art models.
更多查看译文
关键词
Digital pathology,Deblurring,Deep learning,Out-of-focus,Focus quality assessment
AI 理解论文
溯源树
样例
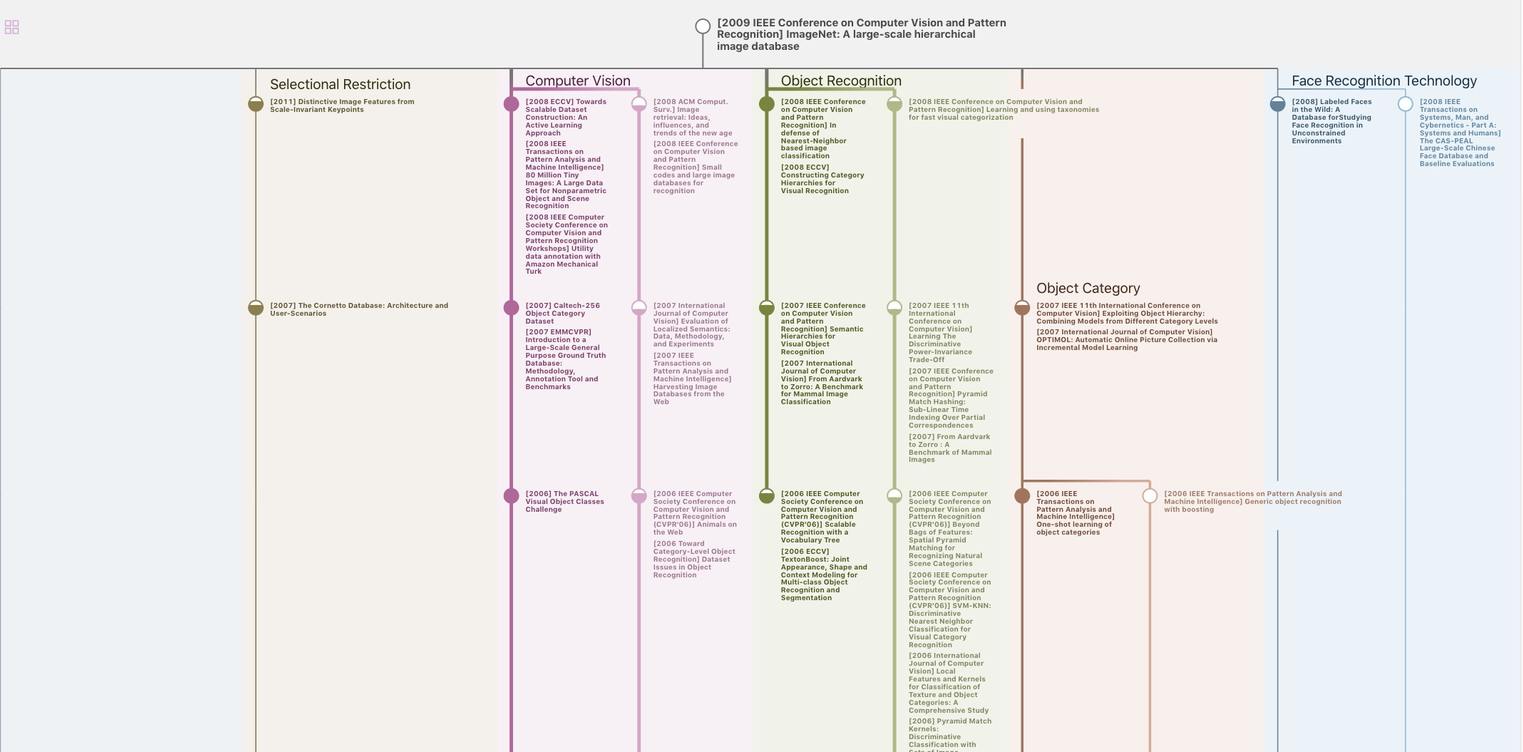
生成溯源树,研究论文发展脉络
Chat Paper
正在生成论文摘要