Bayesian Learning-Based Kalman Smoothing For Linear Dynamical Systems With Unknown Sparse Inputs
ICASSP 2024 - 2024 IEEE International Conference on Acoustics, Speech and Signal Processing (ICASSP)(2024)
摘要
We consider the problem of jointly estimating the states and sparse inputs of a linear dynamical system using noisy low-dimensional observations. We exploit the underlying sparsity in the inputs using fictitious sparsity-promoting Gaussian priors with unknown variances (as hyperparameters). We develop two Bayesian learning-based techniques to estimate states and inputs: sparse Bayesian learning and variational Bayesian inference. Through numerical simulations, we illustrate that our algorithms outperform the conventional Kalman filtering based algorithm and other state-of-the-art sparsity-driven algorithms, especially in the low-dimensional measurement regime.
更多查看译文
关键词
Kalman smoothing,robust filtering,sparse Bayesian learning,variational Bayesian inference,Gaussian prior
AI 理解论文
溯源树
样例
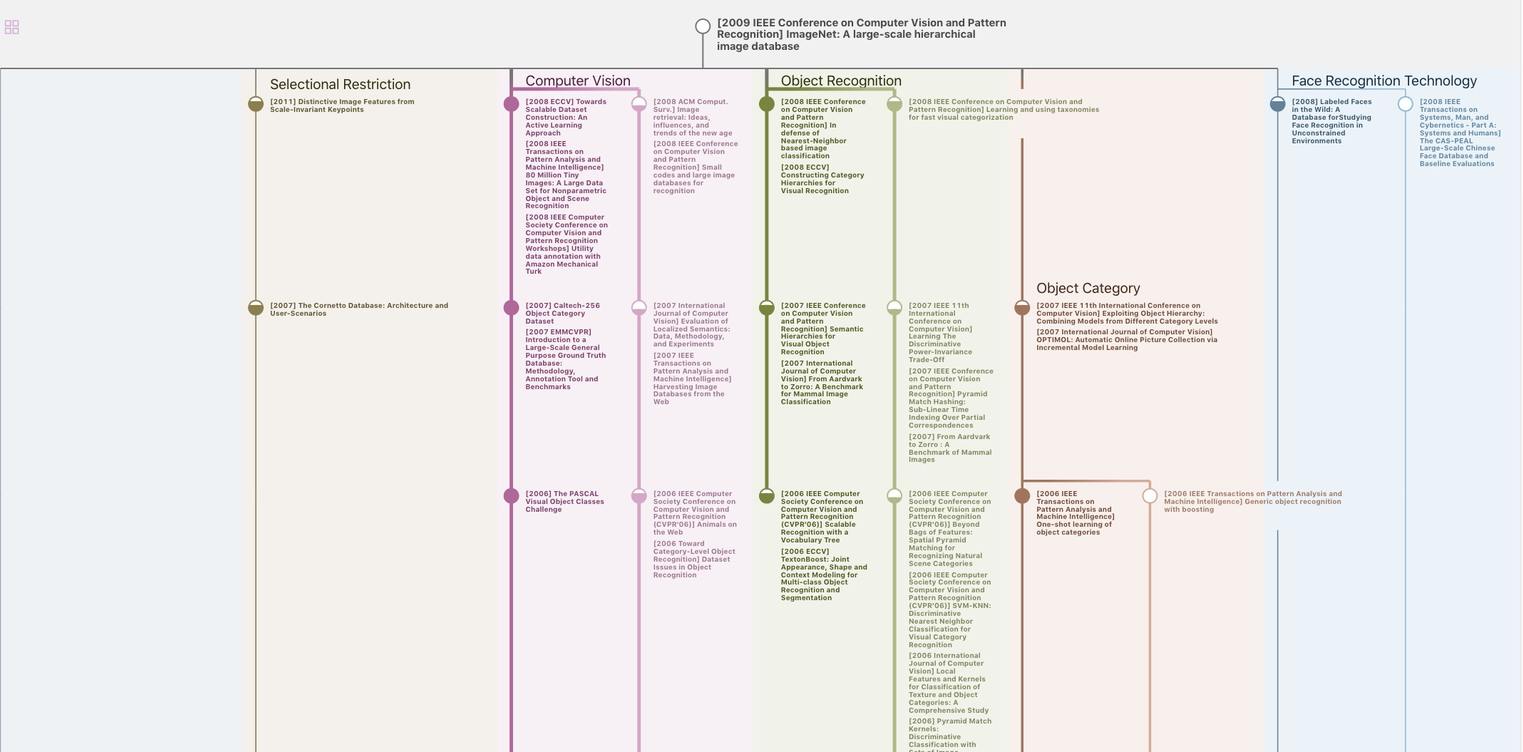
生成溯源树,研究论文发展脉络
Chat Paper
正在生成论文摘要