Hot-Fixing Wake Word Recognition for End-to-End ASR Via Neural Model Reprogramming
ICASSP 2024 - 2024 IEEE International Conference on Acoustics, Speech and Signal Processing (ICASSP)(2024)
摘要
This paper proposes two novel variants of neural reprogramming to enhance wake word recognition in streaming end-to-end ASR models without updating model weights. The first, "trigger-frame reprogramming", prepends the input speech feature sequence with the learned trigger-frames of the target wake word to adjust ASR model’s hidden states for improved wake word recognition. The second, "predictor-state initialization", trains only the initial state vectors (cell and hidden states) of the LSTMs in the prediction network. When applying to a baseline LibriSpeech Emformer RNN-T model with a 98% wake word verification false rejection rate (FRR) on unseen wake words, the proposed approaches achieve 76% and 97% relative FRR reductions with no increase on false acceptance rate. In-depth characteristic analyses of the proposed approaches are also conducted to provide deeper insights. These approaches offer an effective hot-fixing methods to improve wake word recognition performance in deployed production ASR models without the need for model updates.
更多查看译文
关键词
Neural model reprogramming,Hot-fixing,End-to-end ASR,Wake word verification
AI 理解论文
溯源树
样例
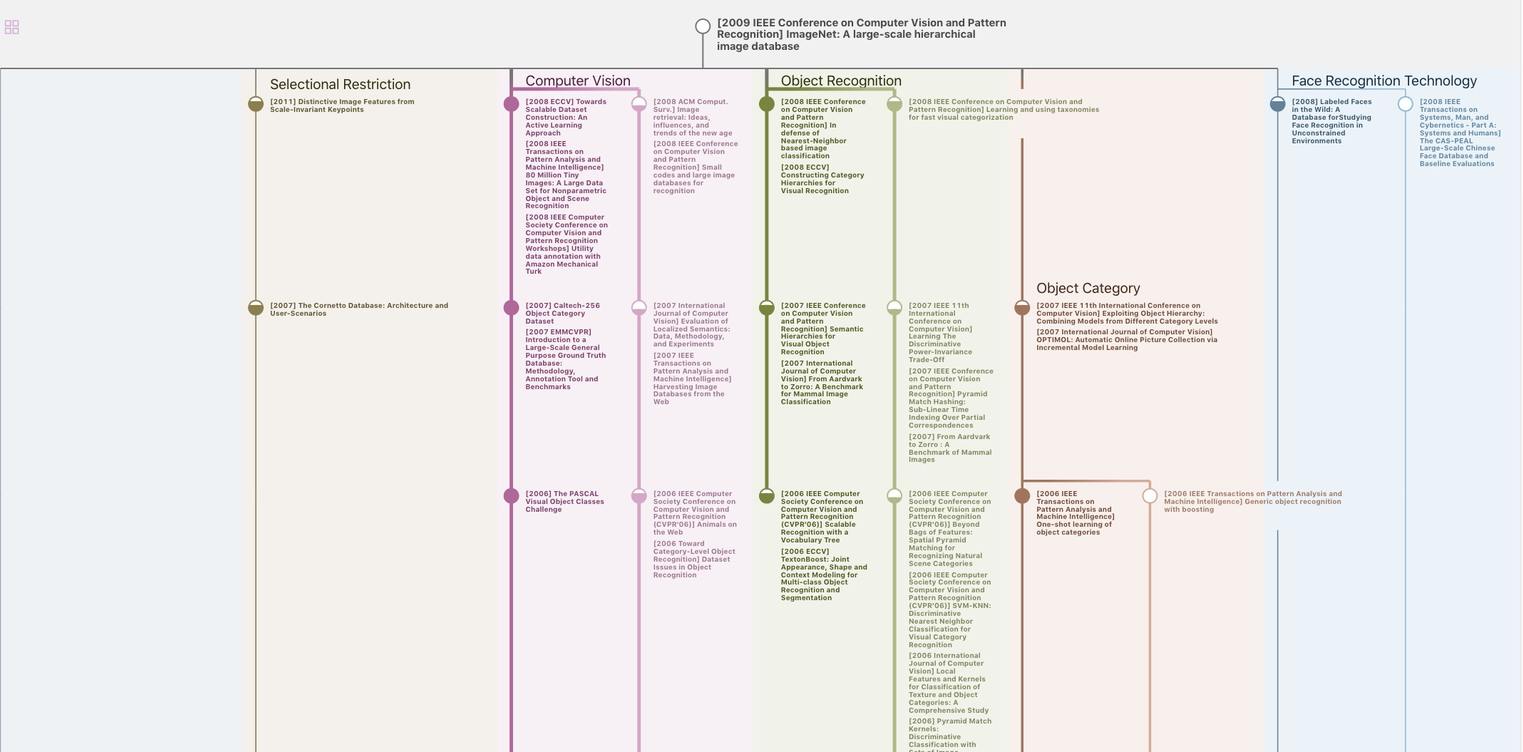
生成溯源树,研究论文发展脉络
Chat Paper
正在生成论文摘要