Sunflower Strategy for Bayesian Relational Data Analysis
ICASSP 2024 - 2024 IEEE International Conference on Acoustics, Speech and Signal Processing (ICASSP)(2024)
摘要
This paper proposes a new inference strategy for Bayesian relational data analysis, inspired by the sunflower lemma in extremal combinatorics. Relational data analysis using rectangular partitioning is a particularly popular analysis tool due to its interpretability and explainability, and has been used in a wide range of signal processing and machine learning applications such as network community detection and cluster structure extraction from cell gene expression data. On the other hand, the combinatorial space of rectangular partitions makes the derivation of its inference algorithms difficult. As a result, many inference algorithms, such as Markov chain Monte Carlo (MCMC) methods, have suffered from local mode and slow mixing problems. Therefore, we propose a method of inference to avoid the difficult combinatorial space by using tractable auxiliary spaces with relaxed constraints, utilizing the knowledge of the sunflower lemma in extremal combinatorics. Specifically, we construct a MCMC method to induce a target combinatorial space of rectangular partitions as the intersection (as the core of a sunflower) of sufficiently and redundantly many relaxed and tractable spaces (as petals of the sunflower). Through experiments, we will show that our method has better prediction performance than previous state-of-the-art methods for network community detection and cluster structure extraction of gene-cell expression data tasks.
更多查看译文
关键词
Sunflower lemma,Relational data analysis,Bayesian methods,Markov chain Monte Carlo
AI 理解论文
溯源树
样例
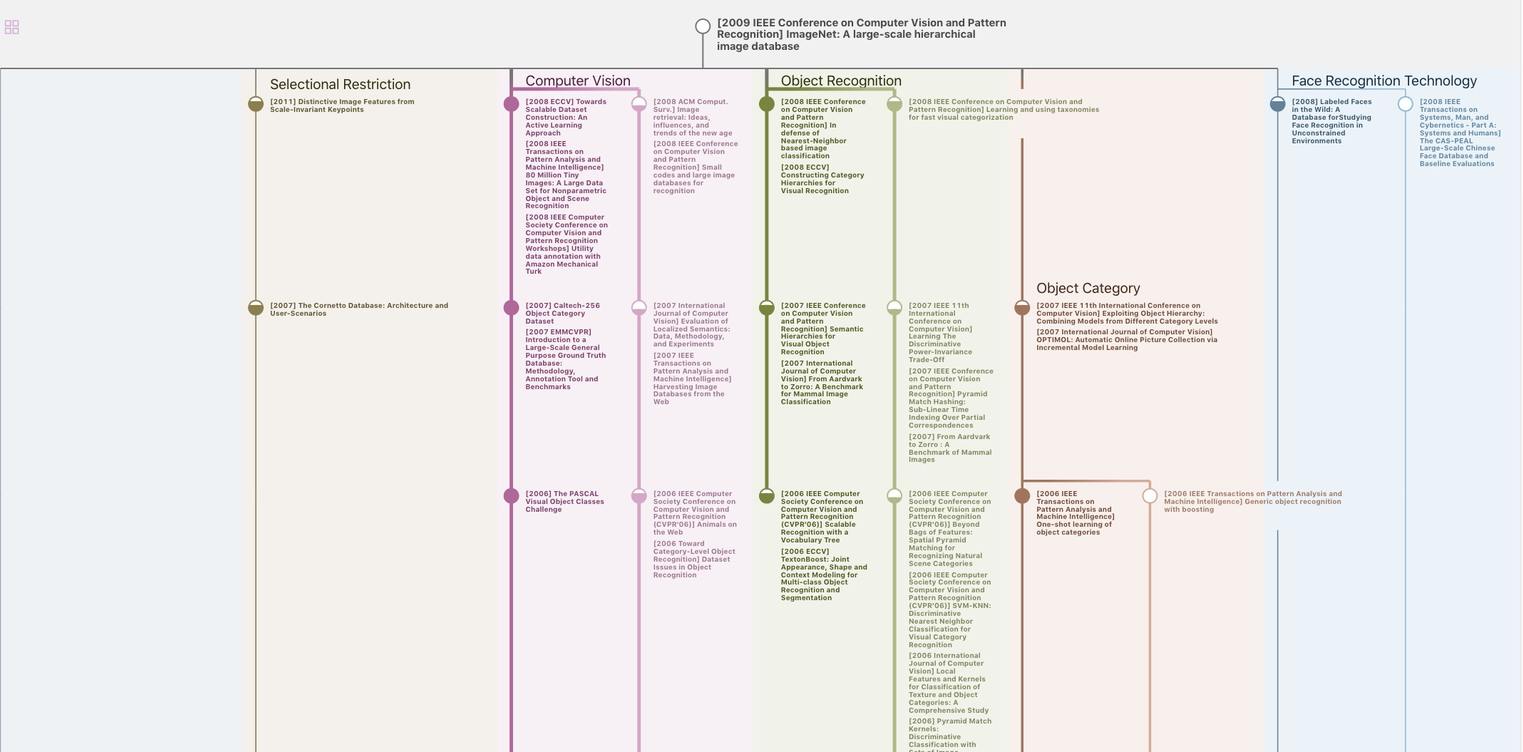
生成溯源树,研究论文发展脉络
Chat Paper
正在生成论文摘要