Spiral Shape Matters: Novel Bio-Inspired Cochlear Cepstrum
ICASSP 2024 - 2024 IEEE International Conference on Acoustics, Speech and Signal Processing (ICASSP)(2024)
摘要
While machines struggle to cope with acoustical variability and noise, humans show remarkable robustness to recognize speech content under different conditions of environmental noise. The tonotopic organization of the spiral human cochlea has motivated the signal processing community for its superb frequency tuning capabilities. In this work, we design and evaluate a novel spiral cochlear cepstrum space, as a novel, directional feature engineering framework, using a cochlear transform approach, that results in tonotopically organized, orthogonal cochlear modes. Such cochlear modes are then transformed to the spiral cochlear cepstral space, yielding cochlear filterbank cepstral coefficients (CFCCs). As opposed to previous works that define the bio-inspired cepstral features based on Mel-, Equivalent Rectangular Bandwidth (ERB) or linear scales, we define the scaling based on the cochlear spiral geometry that spans from θ = 0° at the base to θ = 990° at the apex. We then compute the log function and the discrete cosine transform of the cochlear modes energy yielding spatially supported cepstral features along the spiral cochlear space, spaced by θ = 45°. We assess the impact of noise on the CFCCs and compare the performance to that of Mel-Frequency Cepstral Coefficients (MFCCs) and Gammatone Filterbank Cepstral Coefficients (GFCCs) using the NOIZEUS dataset. We report, for the first time, that the superiority of the CFCCs noise-robustness stems from the geometrical organization of the cochlea (i.e., its tonotopic map) when evaluated on speech signals contaminated with different noise conditions at different SNRs. The proposed CFCCs constitute a platform for a new class, bio-inspired and noise-robust feature extraction for many applications such as speaker recognition.
更多查看译文
关键词
Ear Tonotopic Cespstrum,Cepstral Analysis,Cochlear Transform,MFCCs,Bio-inspired Feature Extraction,CFCCs
AI 理解论文
溯源树
样例
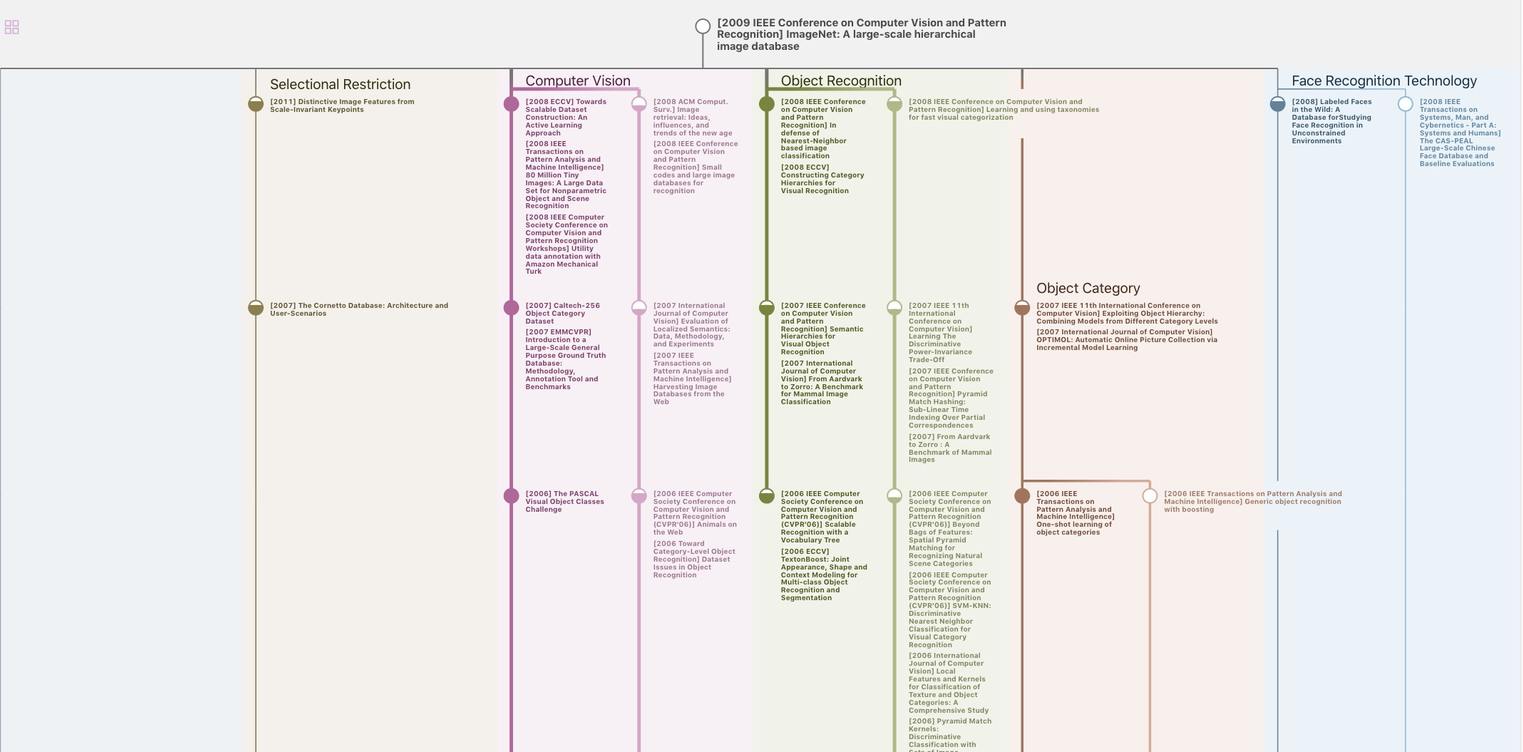
生成溯源树,研究论文发展脉络
Chat Paper
正在生成论文摘要