Refining Text Input For Augmentative and Alternative Communication (AAC) Devices: Analysing Language Model Layers For Optimisation
ICASSP 2024 - 2024 IEEE International Conference on Acoustics, Speech and Signal Processing (ICASSP)(2024)
摘要
Communication impairments are prevalent among a significant proportion of individuals. Methods of Augmentative and Alternative Communication (AAC) can support people with speech disorders (PwSD) to some extent, but AAC users encounter substantial difficulties when engaging in open-domain social interactions, especially involving multiple participants. This is mainly due to the significant communication rate gap between typical speakers and AAC users. Large Language Models (LLM) offer a solution by providing predictions of the next words or sentences. This work analyses refining the prediction capabilities of Masked Language Models (MLM) for AAC users by performing layer-wise analysis specifically for word prediction on an AAC corpus. Experiments show that fine-tuning only specific low-performing LLM layers leads to better results than fine-tuning of the entire model. Fine-tuning of specific layers of a Robust Bidirectional Encoder Representations from Transformers (RoBERTa) model outperforms other tested models; for qualitative evaluation and informal prototype AAC device testing. Fine-tuning the word predictions in an AAC context results in approx. 20% increase in average communication rate (across different communication scenarios) to input speed of approx. 30 words per minute (WPM).
更多查看译文
关键词
Language Modelling,Augmentative and Alternative Communication (AAC),Text-to-Speech (TTS),Communication Rates
AI 理解论文
溯源树
样例
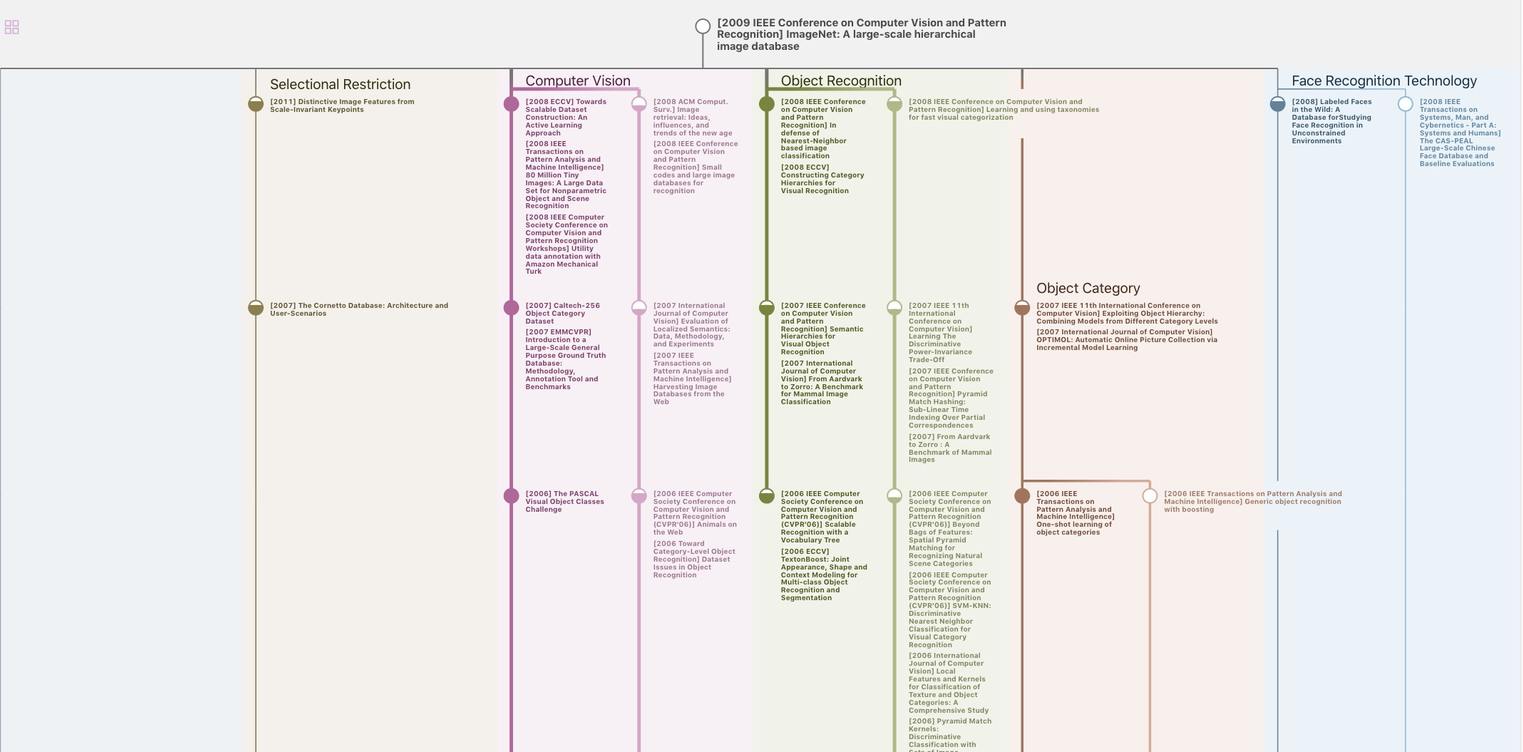
生成溯源树,研究论文发展脉络
Chat Paper
正在生成论文摘要