Reparameterization Head for Efficient Multi-Input Networks
ICASSP 2024 - 2024 IEEE International Conference on Acoustics, Speech and Signal Processing (ICASSP)(2024)
摘要
Reparameterization techniques have demonstrated their efficacy in improving the efficiency of deep neural networks. However, their application has been largely confined to single-input network structures, leaving multi-input ones, commonly encountered in real-world applications, largely unexplored. In this paper, we formulate reparameterization head (RepHead), the first framework designed to introduce reparameterization into multi-input neural networks. RepHead compresses multiple inputs into a single input and employs reconstruction operations to recover them, thereby transforming multi-input networks into single-input, multibranch architectures, thereby enabling the application of reparameterization. We demonstrate the usage of RepHead in both image and point cloud domains. Extensive experimental results validate that the integration of RepHead substantially reduces computational overhead and memory requirements while maintaining minimal performance loss.
更多查看译文
关键词
multi-input networks,deep neural network,reparameterization,efficient
AI 理解论文
溯源树
样例
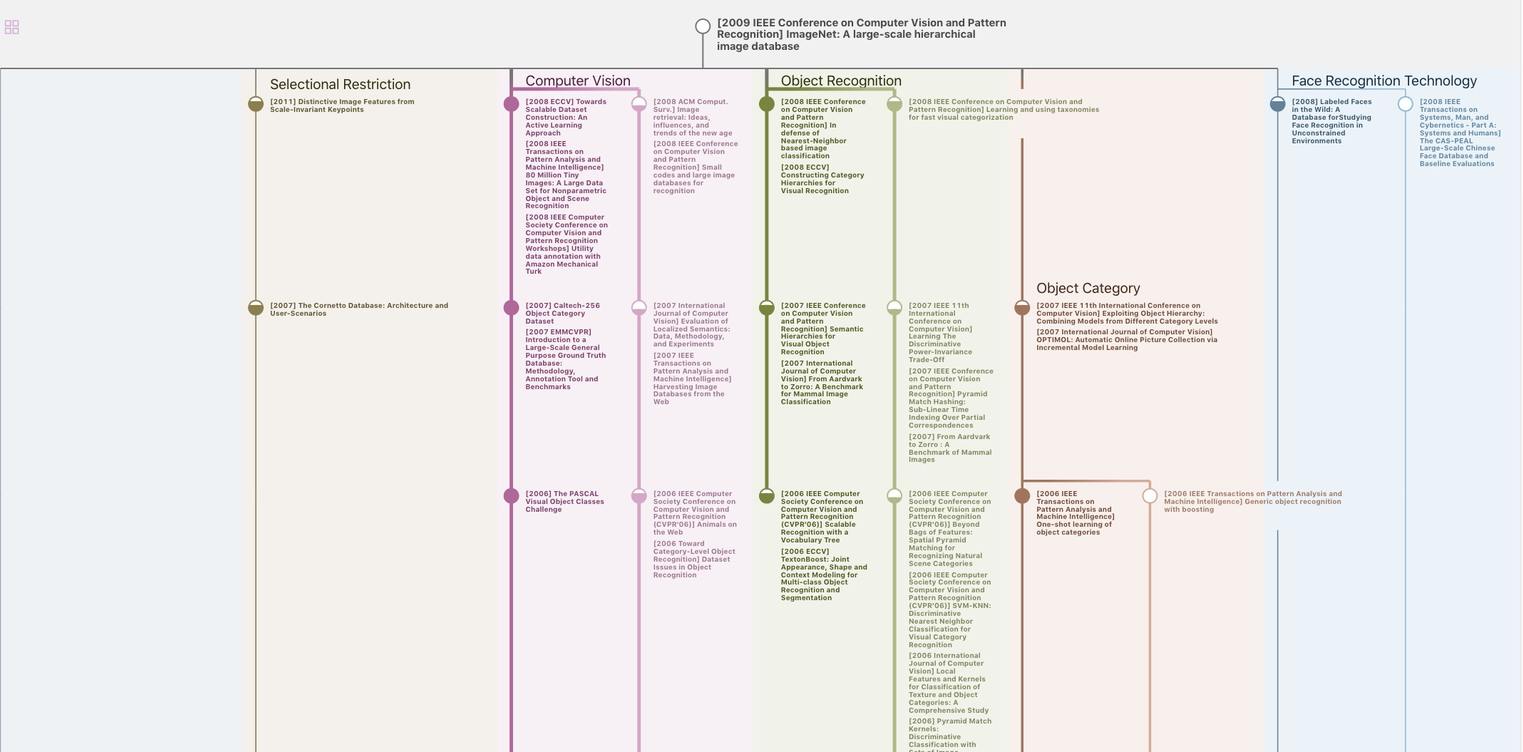
生成溯源树,研究论文发展脉络
Chat Paper
正在生成论文摘要