Robust Self-Supervised Learning with Contrast Samples for Natural Language Understanding
ICASSP 2024 - 2024 IEEE International Conference on Acoustics, Speech and Signal Processing (ICASSP)(2024)
摘要
To improve the robustness of pre-trained language models (PLMs), previous studies have focused more on how to efficiently obtain adversarial samples with similar semantics, but less attention has been paid to the perturbed samples that change the gold label. Therefore, to fully perceive the effects of these different types of small perturbations on robustness, we propose a RObust Self-supervised leArning (ROSA) method, which incorporates different types of perturbed samples and the robustness improvements into a unified framework. Subsequently, to implement ROSA, a perturbed sample generation strategy supported by the large language models (LLMs) is proposed, which adaptively controls the generation process based on the fine-grained similarity information among the training samples. The experimental results demonstrate the remarkable performance of our ROSA.
更多查看译文
关键词
Natural Language Processing,Large Language Models,Robustness,Contrastive Learning
AI 理解论文
溯源树
样例
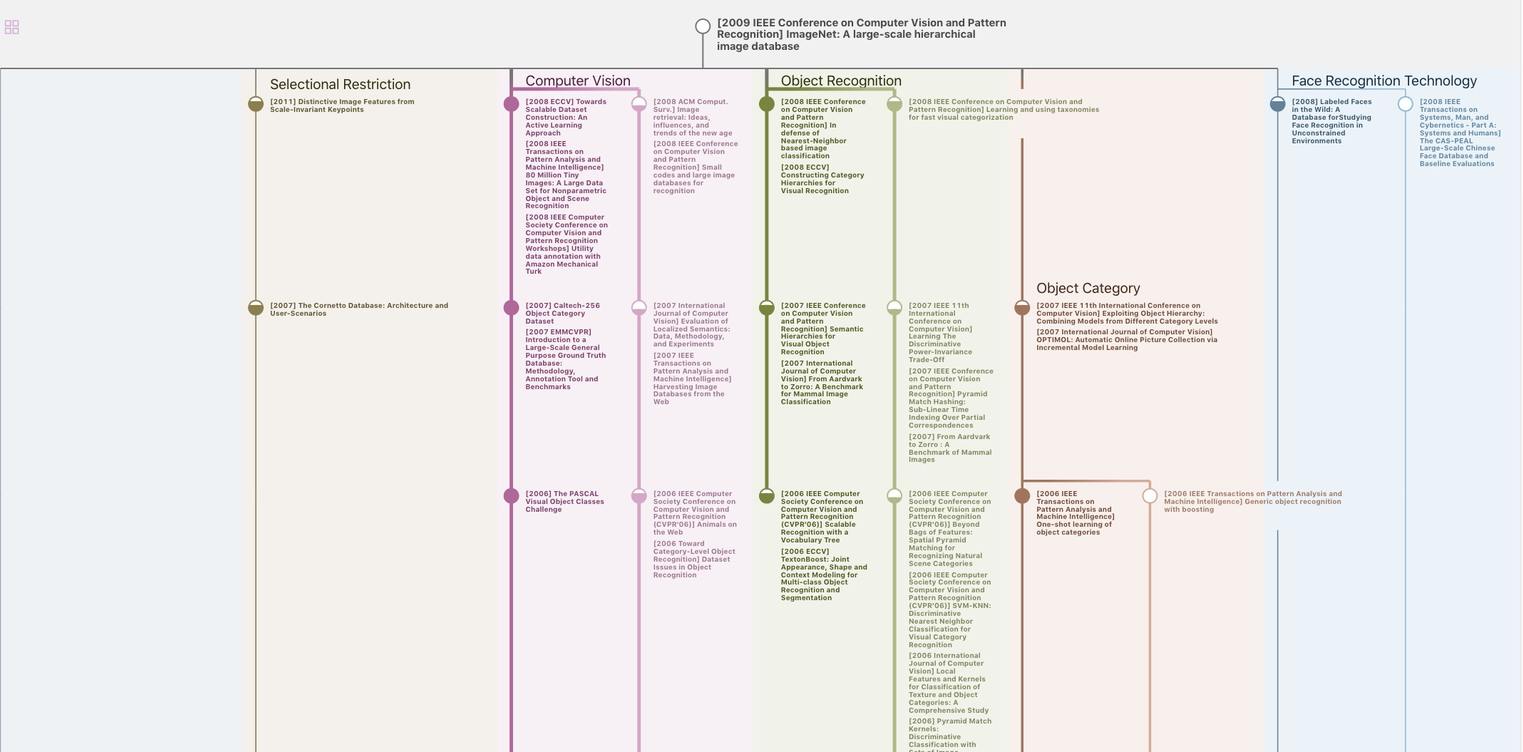
生成溯源树,研究论文发展脉络
Chat Paper
正在生成论文摘要