Deep Convolution Network Based Super Resolution DOA Estimation with Toeplitz and Sparse Prior
ICASSP 2024 - 2024 IEEE International Conference on Acoustics, Speech and Signal Processing (ICASSP)(2024)
摘要
In this paper, a deep learning (DL) based approach is investigated for direction-of-arrival (DOA) estimation, where large-scale uniform linear arrays (ULAs) and small number of samples are considered. Different from existing DL based DOA estimators, the proposed solution first exploits the Toeplitz prior of array covariance matrix and the linear shrinkage technique to obtain an enhanced sample covariance matrix (SCM), which is then formulated as a sparse linear representation (SLR) problem. Finally, a suitable deep convolution network (DCN) that learns such a SLR characteristic from large training dataset is designed. With aid of Toeplitz and sparse prior, the proposed solution can provide an increased resolution and estimation accuracy under the considered scenario, as verified by simulations.
更多查看译文
关键词
Direction of arrival estimation,deep convolution network,Toeplitz prior,sparse prior
AI 理解论文
溯源树
样例
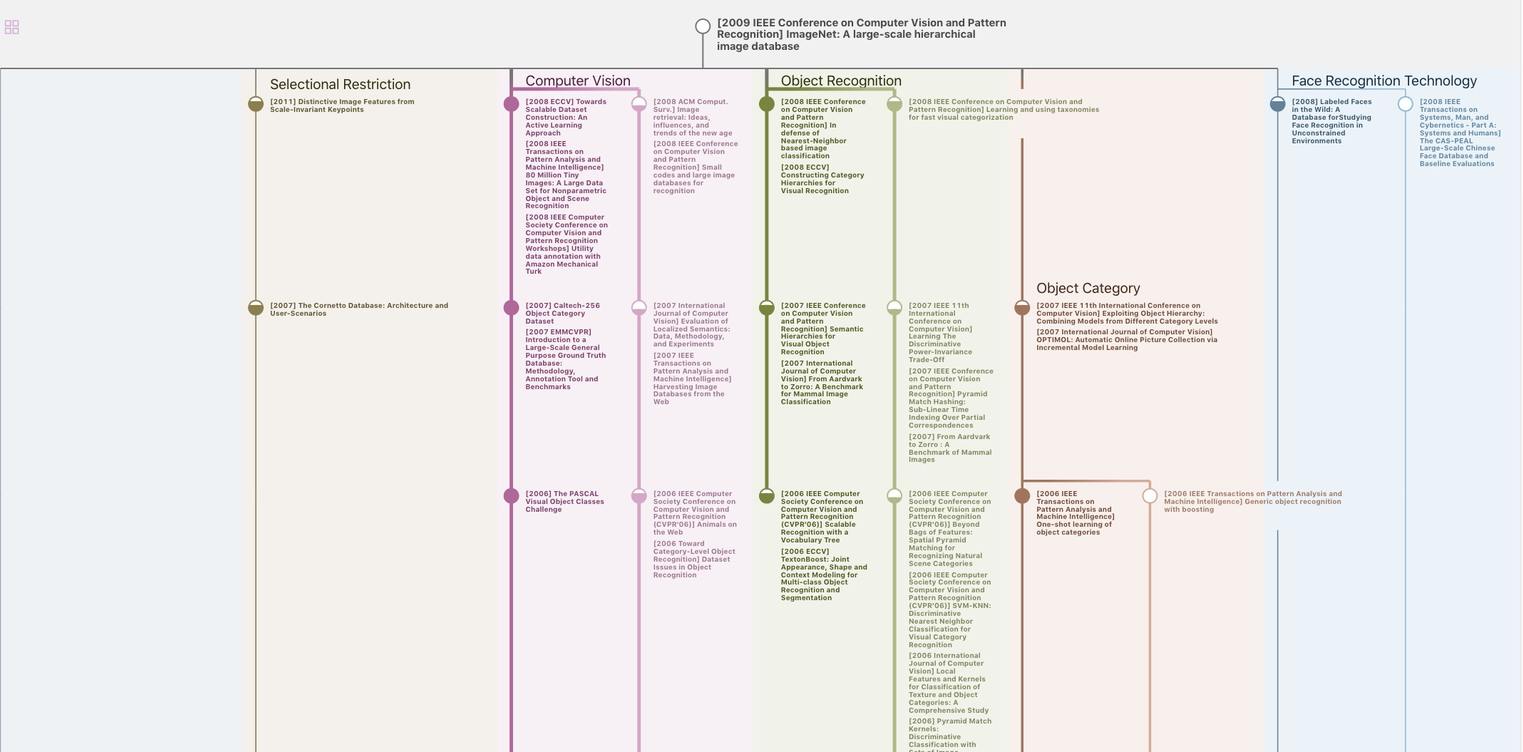
生成溯源树,研究论文发展脉络
Chat Paper
正在生成论文摘要