Adaptive Image-Enhanced Knowledge Graph Completion
ICASSP 2024 - 2024 IEEE International Conference on Acoustics, Speech and Signal Processing (ICASSP)(2024)
摘要
Image-enhanced knowledge graph completion aims to predict potential linkings in KGs by utilizing auxiliary visual information from entity’s images. Ideally, the image selection process should be dynamic, considering the context of inferred relations. However, current methods often assess image importance in a static way, based on how well they represent each entity. To solve above issues, we propose an Adaptive Image-enhanced Knowledge Graph Completion model (AI-KGC). The core idea behind this model is to switch image selection strategies adaptively according to the entity’s role. Specially, for a query entity, we employ a relation-guided dynamic image selection mechanism to select the image that is most appropriate for the given relation context. The chosen image may offer insightful clues about the ground-truth entity. For a candidate entity, we use a name-guided static image selection mechanism to capture its most representative visual features. By exploiting a similarity-based scoring function, the ranking of entities exhibiting similar visual features therefore will be enhanced. This adaptive selection strategy can extract valuable insights from images that previous methods may have overlooked. Extensive experiments conducted on link prediction tasks show that AI-KGC outperforms the state-of-the-art KGC methods remarkably.
更多查看译文
关键词
Multimodal Knowledge Graph,Knowledge Graph Completion,Multi-modal Learning
AI 理解论文
溯源树
样例
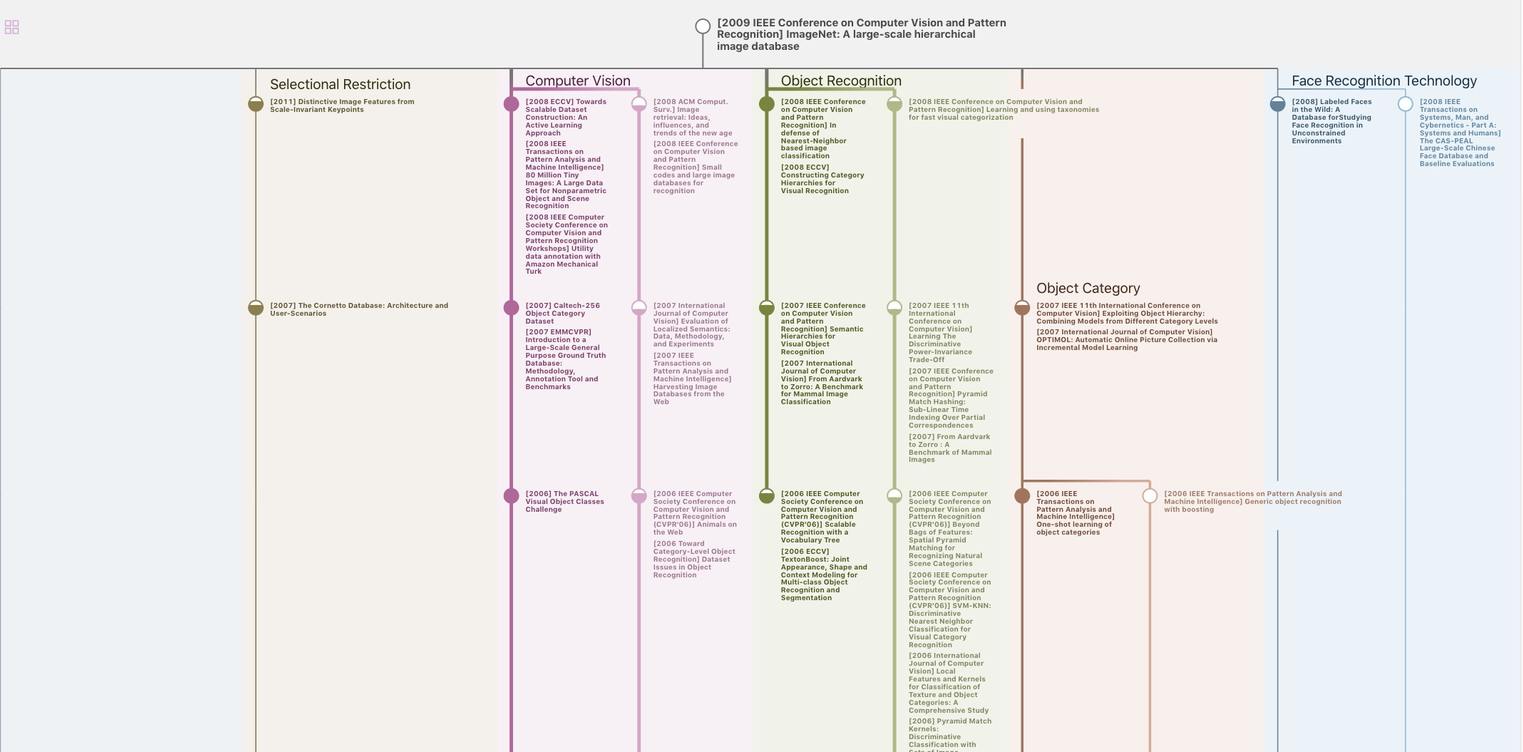
生成溯源树,研究论文发展脉络
Chat Paper
正在生成论文摘要