Understanding Rejection Mechanisms of Trace Organic Contaminants by Polyamide Membranes via Data-Knowledge Codriven Machine Learning
ENVIRONMENTAL SCIENCE & TECHNOLOGY(2024)
摘要
Data-driven machine learning (ML) provides a promising approach to understanding and predicting the rejection of trace organic contaminants (TrOCs) by polyamide (PA). However, various confounding variables, coupled with data scarcity, restrict the direct application of data-driven ML. In this study, we developed a data-knowledge codriven ML model via domain-knowledge embedding and explored its application in comprehending TrOC rejection by PA membranes. Domain-knowledge embedding enhanced both the predictive performance and the interpretability of the ML model. The contribution of key mechanisms, including size exclusion, charge effect, hydrophobic interaction, etc., that dominate the rejections of the three TrOC categories (neutral hydrophilic, neutral hydrophobic, and charged TrOCs) was quantified. Log D and molecular charge emerge as key factors contributing to the discernible variations in the rejection among the three TrOC categories. Furthermore, we quantitatively compared the TrOC rejection mechanisms between nanofiltration (NF) and reverse osmosis (RO) PA membranes. The charge effect and hydrophobic interactions possessed higher weights for NF to reject TrOCs, while the size exclusion in RO played a more important role. This study demonstrated the effectiveness of the data-knowledge codriven ML method in understanding TrOC rejection by PA membranes, providing a methodology to formulate a strategy for targeted TrOC removal.
更多查看译文
关键词
machine learning,data scarcity,domain-knowledgeembedding,trace organic contaminants,polyamidemembranes
AI 理解论文
溯源树
样例
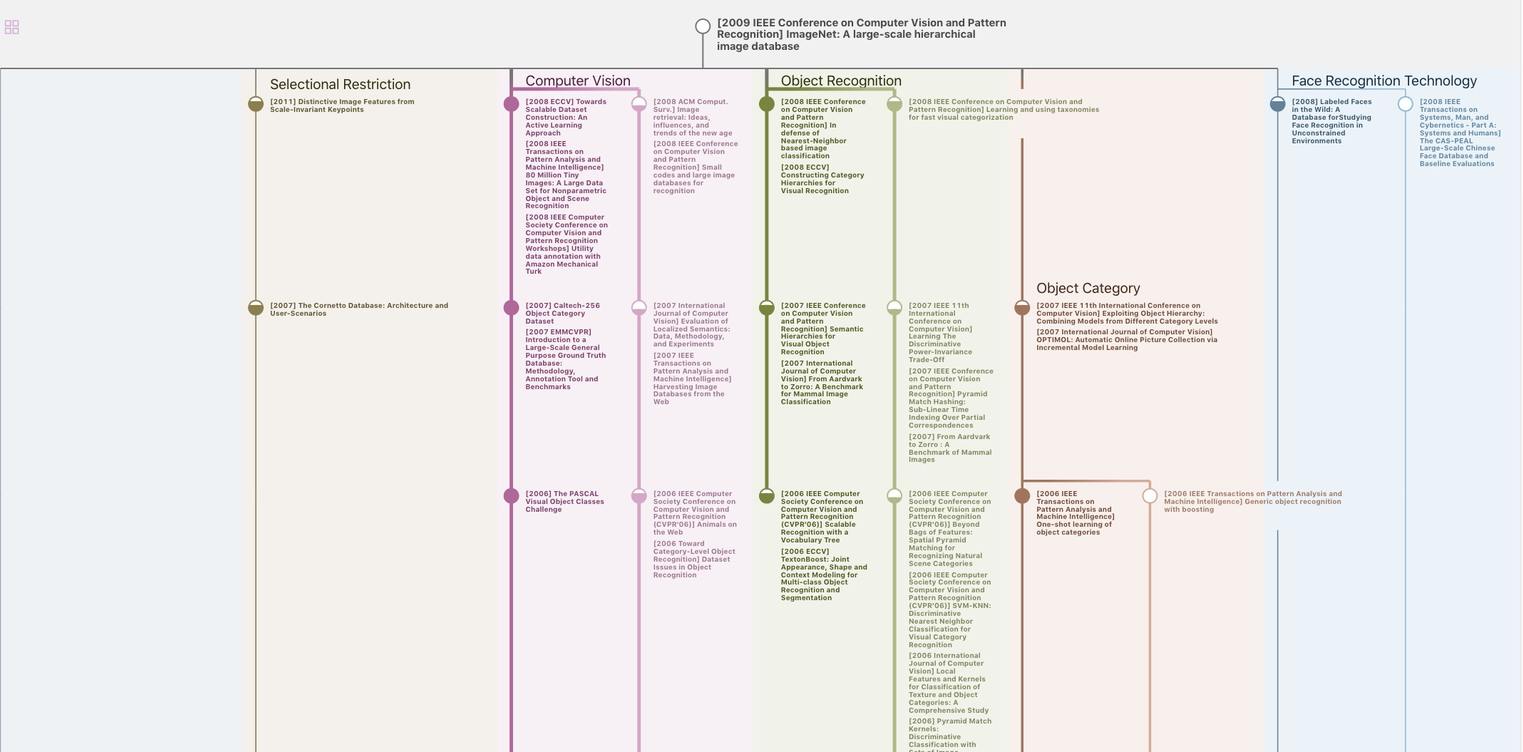
生成溯源树,研究论文发展脉络
Chat Paper
正在生成论文摘要