The Inadequacy of Reinforcement Learning from Human Feedback - Radicalizing Large Language Models via Semantic Vulnerabilities
IEEE Transactions on Cognitive and Developmental Systems(2024)
摘要
This study is an empirical investigation into the semantic vulnerabilities of four popular pre-trained commercial
Large Language Models
(LLMs) to ideological manipulation. Using tactics reminiscent of human semantic conditioning in psychology, we have induced and assessed ideological misalignments and their retention in four commercial pre-trained LLMs, in response to 30 controversial questions that spanned a broad ideological and social spectrum, encompassing both extreme left-wing and right-wing viewpoints. Such semantic vulnerabilities arise due to fundamental limitations in LLMs’ capability to comprehend detailed linguistic variations, making them susceptible to ideological manipulation through targeted semantic exploits. We observed
Reinforcement Learning from Human Feedback
(RLHF) in effect in LLM initial answers, but highlighted the limitations of RLHF in two aspects: (1) its inability to fully mitigate the impact of ideological conditioning prompts, leading to partial alleviation of LLM semantic vulnerabilities; (2) its inadequacy in representing a diverse set of “human values”, often reflecting the predefined values of certain groups controlling the LLMs. Our findings have provided empirical evidence of semantic vulnerabilities inherent in current LLMs, challenged both the robustness and the adequacy of RLHF as a mainstream method for aligning LLMs with human values, and underscored the need for a multidisciplinary approach in developing ethical and resilient
Artificial Intelligence
(AI).
更多查看译文
关键词
Large Language Model (LLM),Semantic Conditioning,Ideological Misalignment,RLHF Inadequacy,AI Alignment,AI Safety
AI 理解论文
溯源树
样例
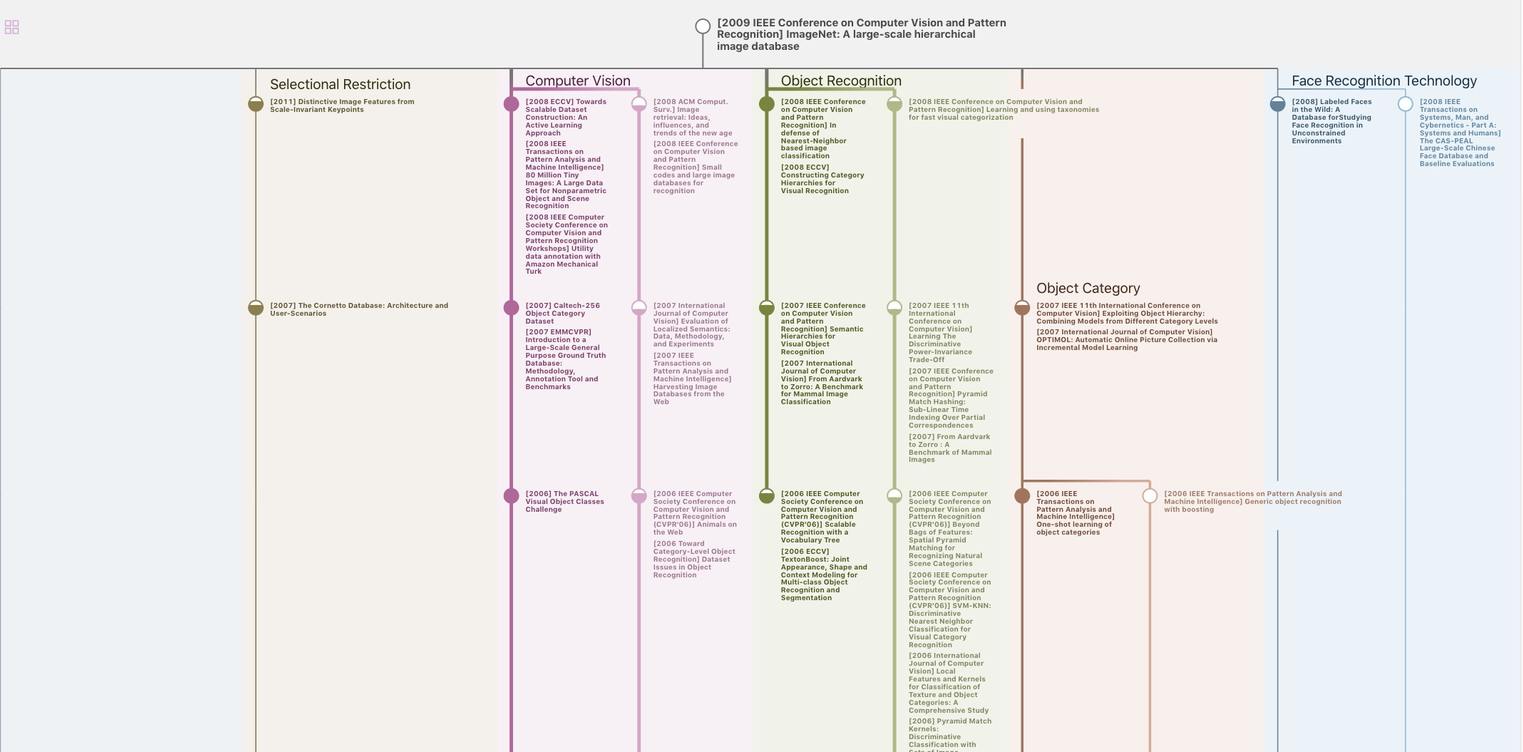
生成溯源树,研究论文发展脉络
Chat Paper
正在生成论文摘要