Towards Efficient and Interpretative Rolling Bearing Fault Diagnosis via Quadratic Neural Network With Bi-LSTM
IEEE Internet of Things Journal(2024)
摘要
With the widespread application of deep learning in Internet of Things (IoT), remarkable achievements have been made especially in rolling bearing fault diagnosis in rotating machinery. However, such complex models commonly have high demand for a large number of parameters and computational resources, and with insufficient interpretability, which restrict their extensive application in real-world industrial applications. To improve efficiency and interpretability, this study innovatively fuses a quadratic neural network (QNN) with a bidirectional long and short-term memory network (Bi-LSTM) to develop a novel hybrid model for quick and accurate diagnosis of rolling bearing faults. The results show that the model fully utilizes the multilayer feature extraction of QNN and the sensitivity of Bi-LSTM to the dynamic evolution of signals to significantly improve the accuracy and speed of fault diagnosis. By visualizing the convolutional kernel response map, the Qttention mapping of QNN, and the hidden states of Bi-LSTM, this study makes progress in interpretability and successfully demonstrates the model’s attention to different features of the bearing signals, which provides users with a more reasonable understanding of the interpretation of the model results.
更多查看译文
关键词
Deep Learning,Internet of Things (IoT),Quadratic Neural Network (QNN),Bidirectional Long and Short-Term Memory Network (Bi-LSTM),Interpretability
AI 理解论文
溯源树
样例
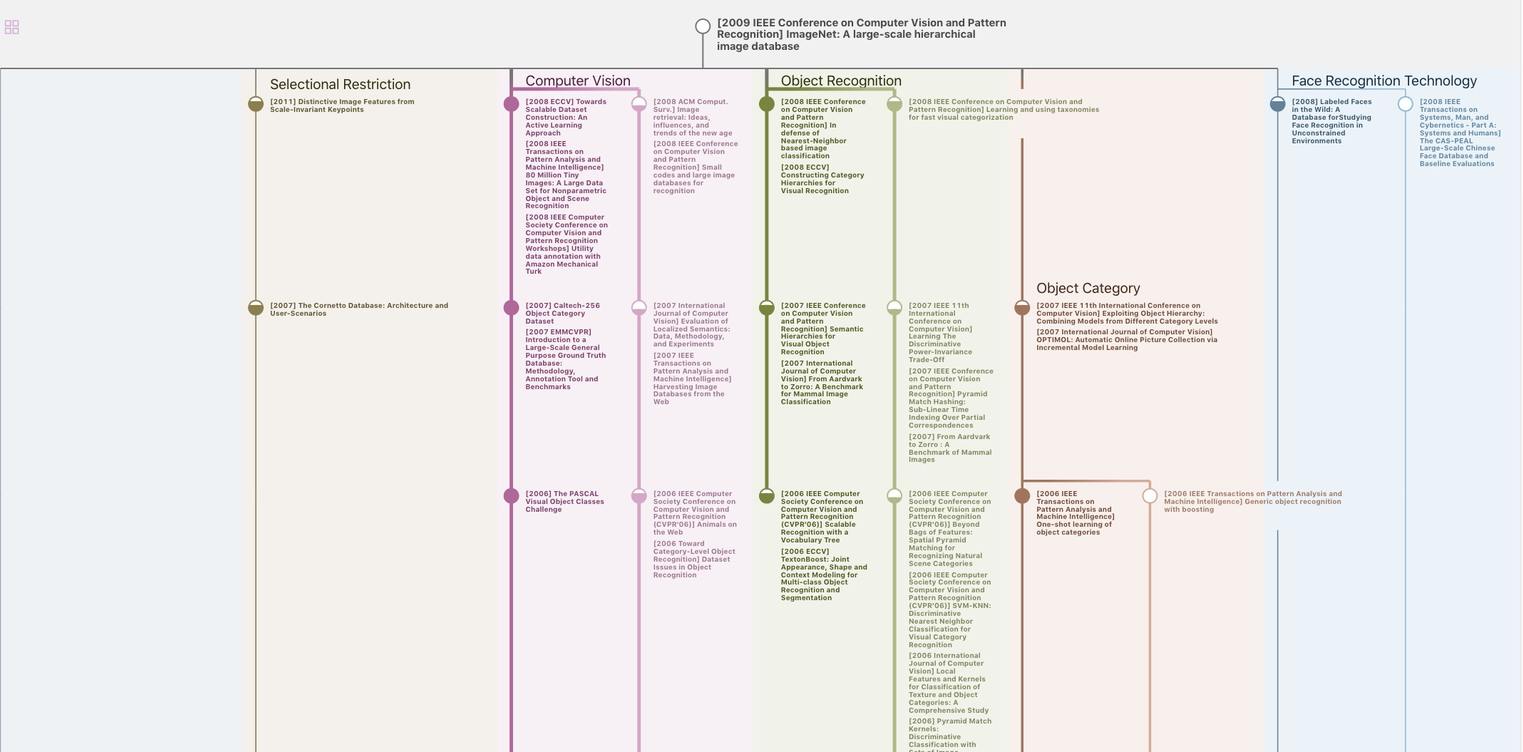
生成溯源树,研究论文发展脉络
Chat Paper
正在生成论文摘要