MMHSV: A Multimodal Handwritten Signature Verification Fusing Dynamic and Static Feature
ICASSP 2024 - 2024 IEEE International Conference on Acoustics, Speech and Signal Processing (ICASSP)(2024)
摘要
In recent years, significant progress has been made in the field of handwritten signature verification through methods based on deep learning. However, due to the high intra-class variability and high inter-class similarity of signature samples, achieving high accuracy and security in handwritten signature verification systems remains challenging. In this paper, we propose a deep learning-based multimodal handwritten signature verification framework, MMHSV, to fuse dynamic and static features by utilizing the complementary signal components of signature images and pen stroke sounds, and explore the feasibility of multimodal signature verification. MMHSV consists of an innovative joint-embedded feature representation method based on multi-task learning and a dual-path network model for signature representation extraction. To support our method, we have curated a multimodal signature dataset, serving as a benchmark for the proposed technique. Preliminary findings suggest that our approach offers a pioneering solution in the realm of handwritten signature verification and security metrics that surpass the benchmarks set by the unimodal state-of-the-art methods.
更多查看译文
关键词
Multimodal handwritten signature verification,contrastive learning,joint embedding,deep learning
AI 理解论文
溯源树
样例
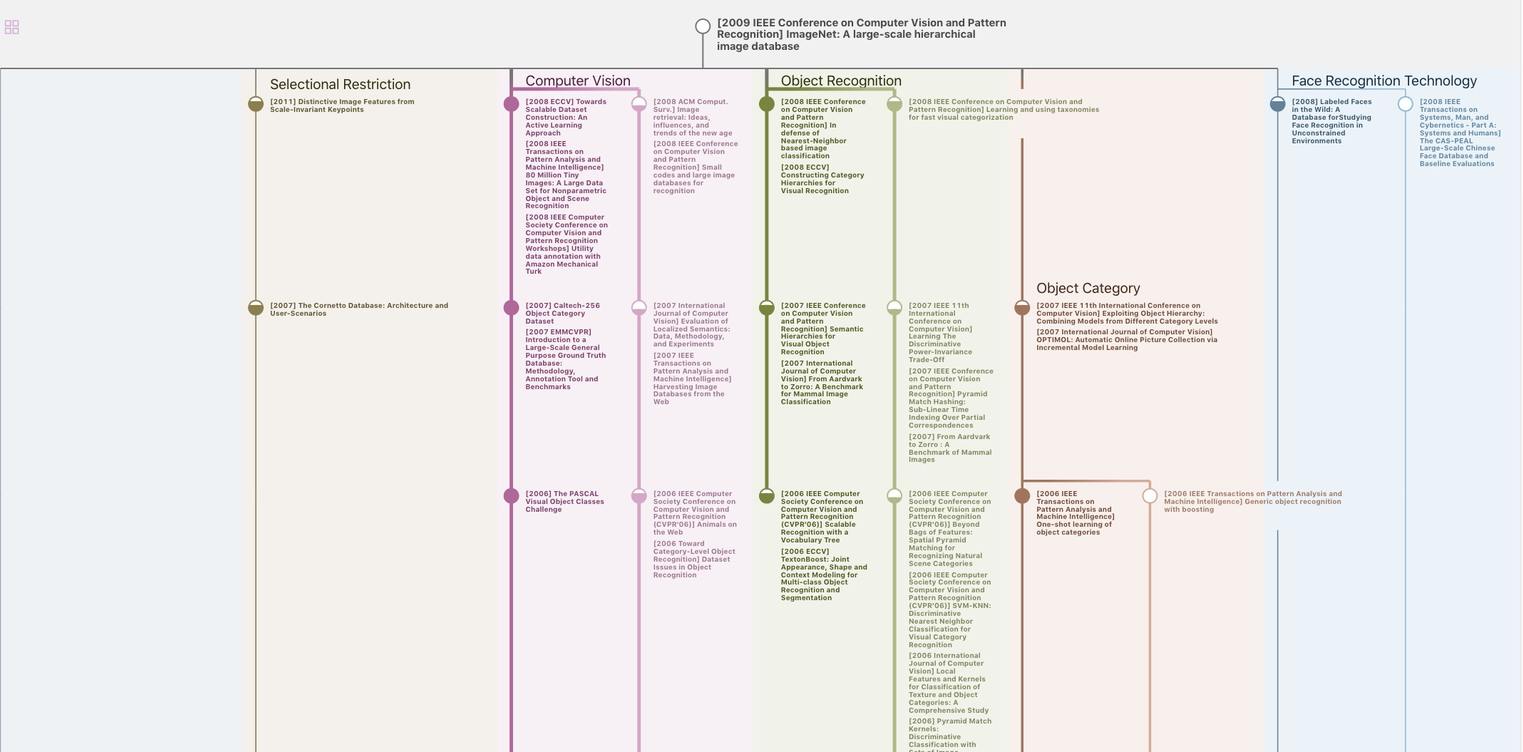
生成溯源树,研究论文发展脉络
Chat Paper
正在生成论文摘要