Error bounds for rank-one DNN reformulation of QAP and DC exact penalty approach
arxiv(2024)
摘要
This paper concerns the quadratic assignment problem (QAP), a class of
challenging combinatorial optimization problems. We provide an equivalent
rank-one doubly nonnegative (DNN) reformulation with fewer equality
constraints, and derive the local error bounds for its feasible set. By
leveraging these error bounds, we prove that the penalty problem induced by the
difference of convexity (DC) reformulation of the rank-one constraint is a
global exact penalty, and so is the penalty problem for its Burer-Monteiro (BM)
factorization. As a byproduct, we verify that the penalty problem for the
rank-one DNN reformulation proposed in is a global exact penalty
without the calmness assumption. Then, we develop a continuous relaxation
approach by seeking approximate stationary points of a finite number of penalty
problems for the BM factorization with an augmented Lagrangian method, whose
asymptotic convergence certificate is also provided under a mild condition.
Numerical comparison with Gurobi for 131 benchmark instances validates
the efficiency of the proposed DC exact penalty approach.
更多查看译文
AI 理解论文
溯源树
样例
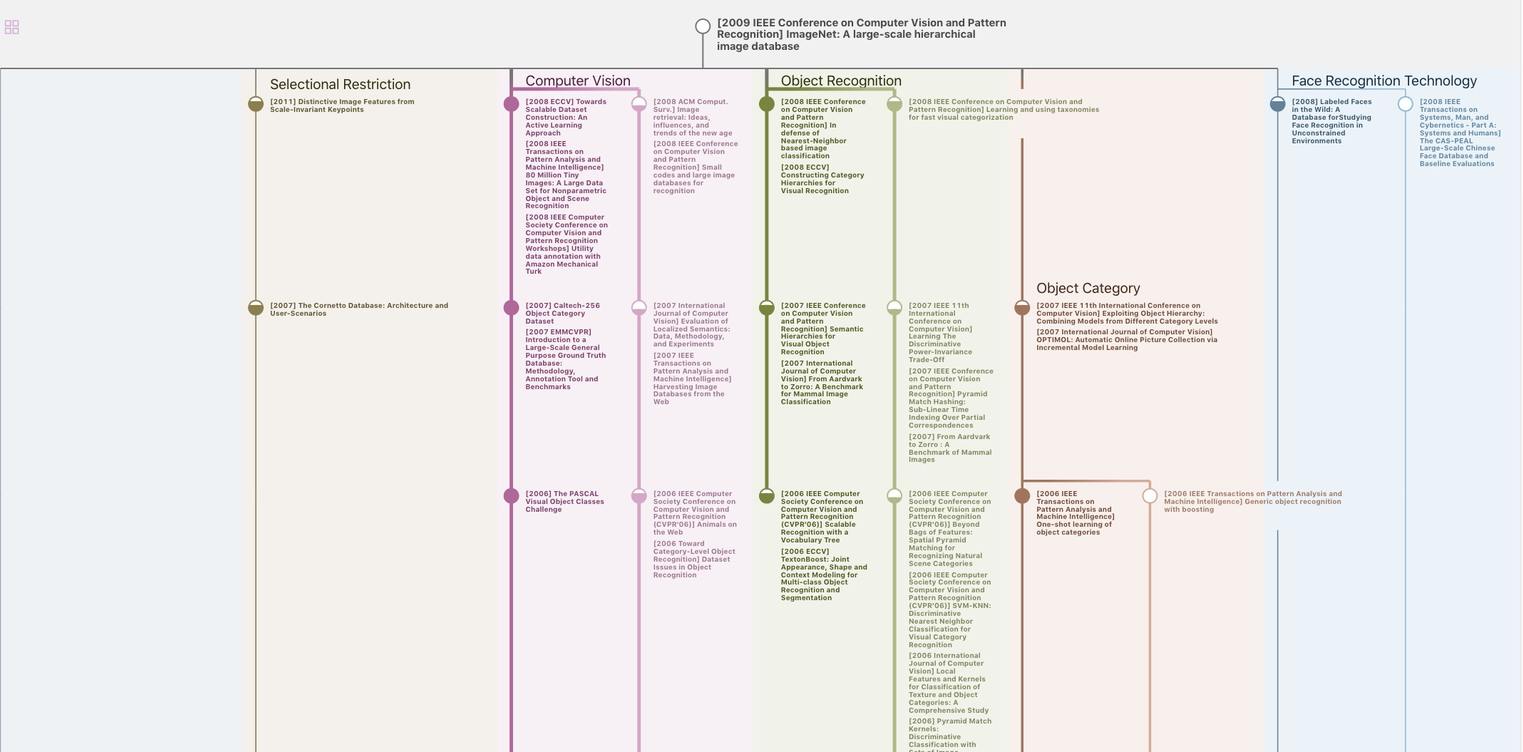
生成溯源树,研究论文发展脉络
Chat Paper
正在生成论文摘要