ECRC: Emotion-Causality Recognition in Korean Conversation for GCN
arxiv(2024)
摘要
In this multi-task learning study on simultaneous analysis of emotions and
their underlying causes in conversational contexts, deep neural network methods
were employed to effectively process and train large labeled datasets. However,
these approaches are typically limited to conducting context analyses across
the entire corpus because they rely on one of the two methods: word- or
sentence-level embedding. The former struggles with polysemy and homonyms,
whereas the latter causes information loss when processing long sentences. In
this study, we overcome the limitations of previous embeddings by utilizing
both word- and sentence-level embeddings. Furthermore, we propose the
emotion-causality recognition in conversation (ECRC) model, which is based on a
novel graph structure, thereby leveraging the strengths of both embedding
methods. This model uniquely integrates the bidirectional long short-term
memory (Bi-LSTM) and graph neural network (GCN) models for Korean conversation
analysis. Compared with models that rely solely on one embedding method, the
proposed model effectively structures abstract concepts, such as language
features and relationships, thereby minimizing information loss. To assess
model performance, we compared the multi-task learning results of three deep
neural network models with varying graph structures. Additionally, we evaluated
the proposed model using Korean and English datasets. The experimental results
show that the proposed model performs better in emotion and causality
multi-task learning (74.62
characteristics are incorporated into the graph structure. Similar results were
recorded for the Korean ECC and Wellness datasets (74.62
respectively) with 71.35
更多查看译文
AI 理解论文
溯源树
样例
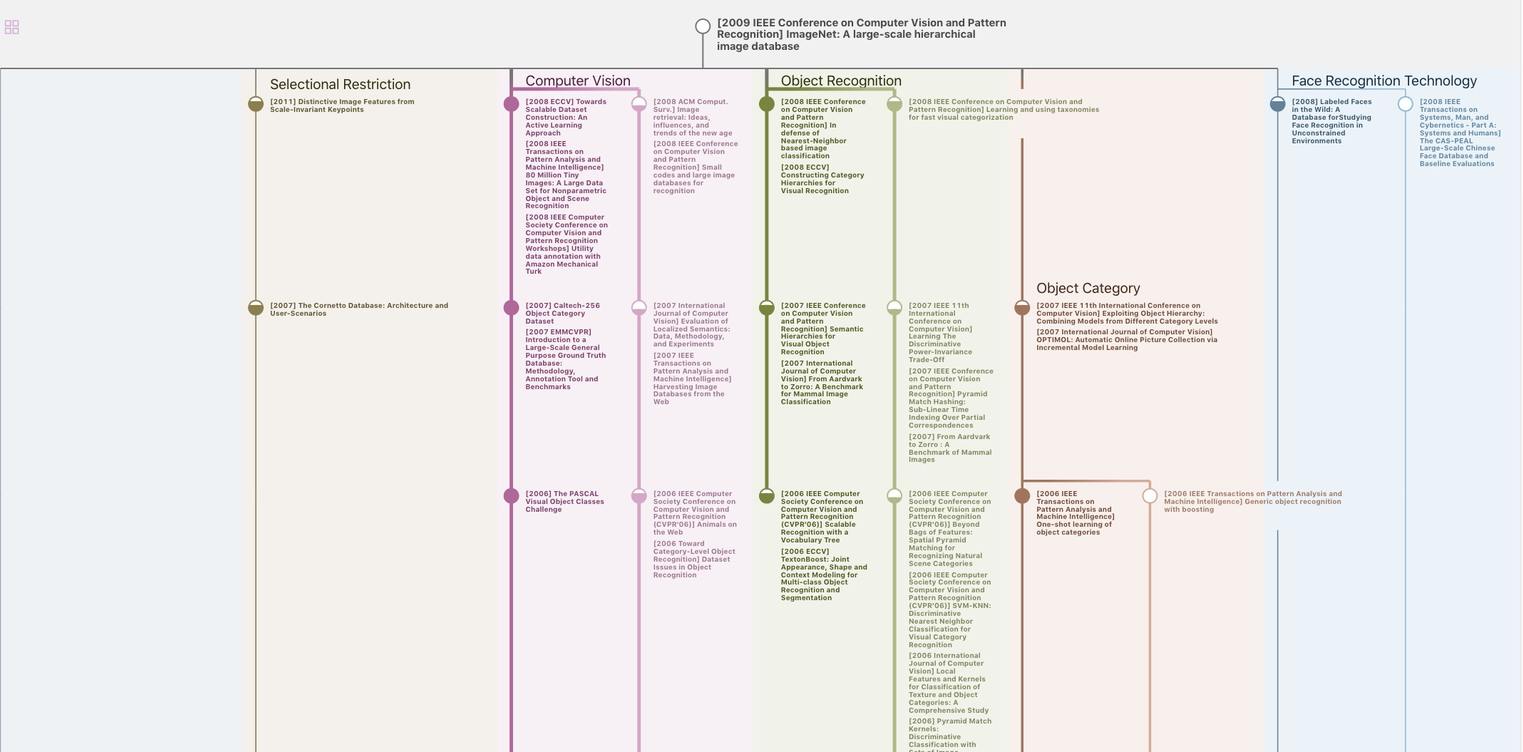
生成溯源树,研究论文发展脉络
Chat Paper
正在生成论文摘要