A Primal-Dual Algorithm for Faster Distributionally Robust Optimization
CoRR(2024)
摘要
We consider the penalized distributionally robust optimization (DRO) problem
with a closed, convex uncertainty set, a setting that encompasses the f-DRO,
Wasserstein-DRO, and spectral/L-risk formulations used in practice. We
present Drago, a stochastic primal-dual algorithm that achieves a
state-of-the-art linear convergence rate on strongly convex-strongly concave
DRO problems. The method combines both randomized and cyclic components with
mini-batching, which effectively handles the unique asymmetric nature of the
primal and dual problems in DRO. We support our theoretical results with
numerical benchmarks in classification and regression.
更多查看译文
AI 理解论文
溯源树
样例
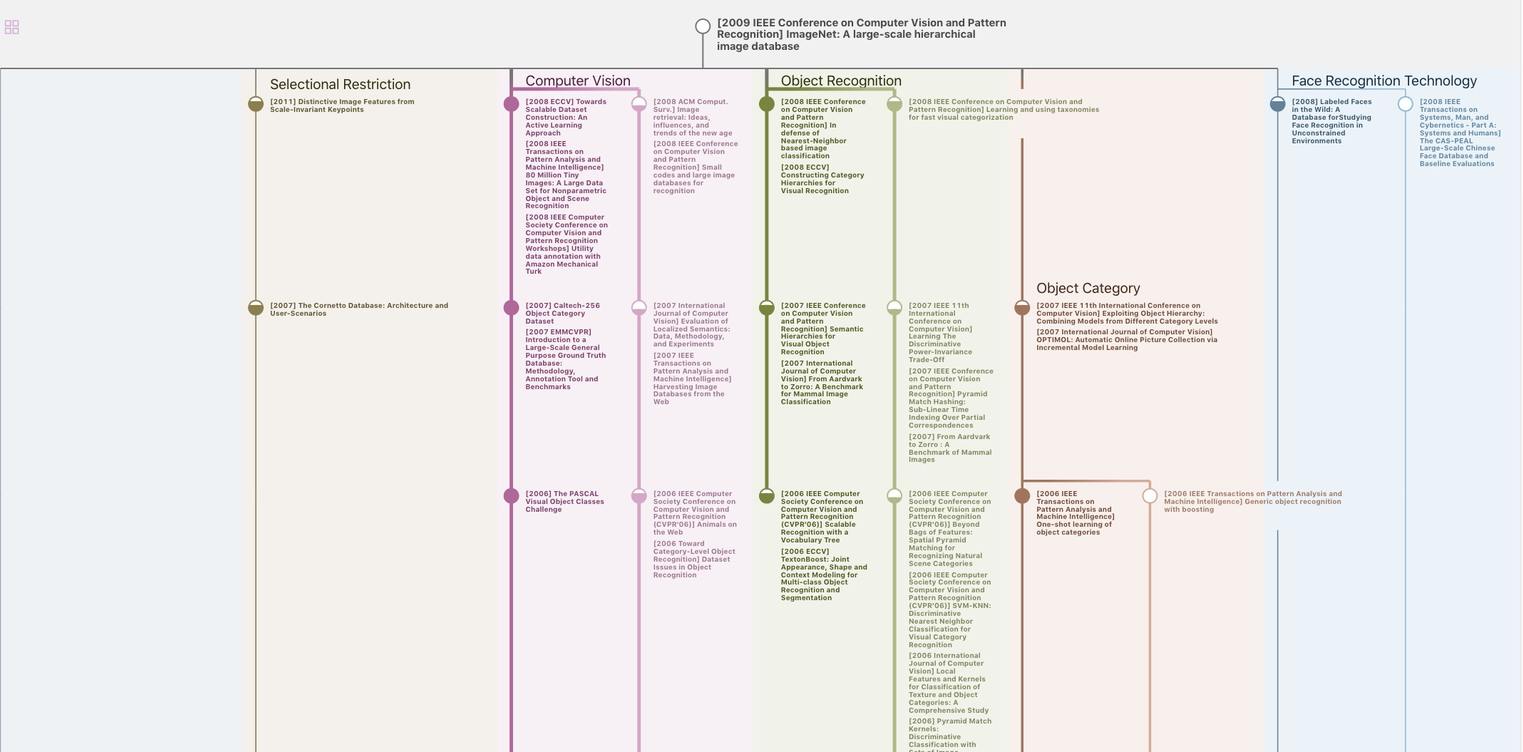
生成溯源树,研究论文发展脉络
Chat Paper
正在生成论文摘要