Learning to project in a criterion space search algorithm: an application to multi-objective binary linear programming
Optimization Letters(2024)
摘要
In this paper, we investigate the possibility of improving the performance of multi-objective optimization solution approaches using machine learning techniques. Specifically, we focus on multi-objective binary linear programs and employ one of the most effective and recently developed criterion space search algorithms, the so-called KSA, during our study. This algorithm computes all nondominated points of a problem with p objectives by searching on a projected criterion space, i.e., a (p-1) -dimensional criterion apace. We present an effective and fast learning approach to identify on which projected space the KSA should work. We also present several generic features/variables that can be used in machine learning techniques for identifying the best projected space. Finally, we present an effective bi-objective optimization-based heuristic for selecting the subset of the features to overcome the issue of overfitting in learning. Through an extensive computational study over 2000 instances of tri-objective knapsack and assignment problems, we demonstrate that an improvement of up to 18
更多查看译文
关键词
Multiple objective programming,Machine learning,Binary linear program,Criterion space search algorithm,Learning to project
AI 理解论文
溯源树
样例
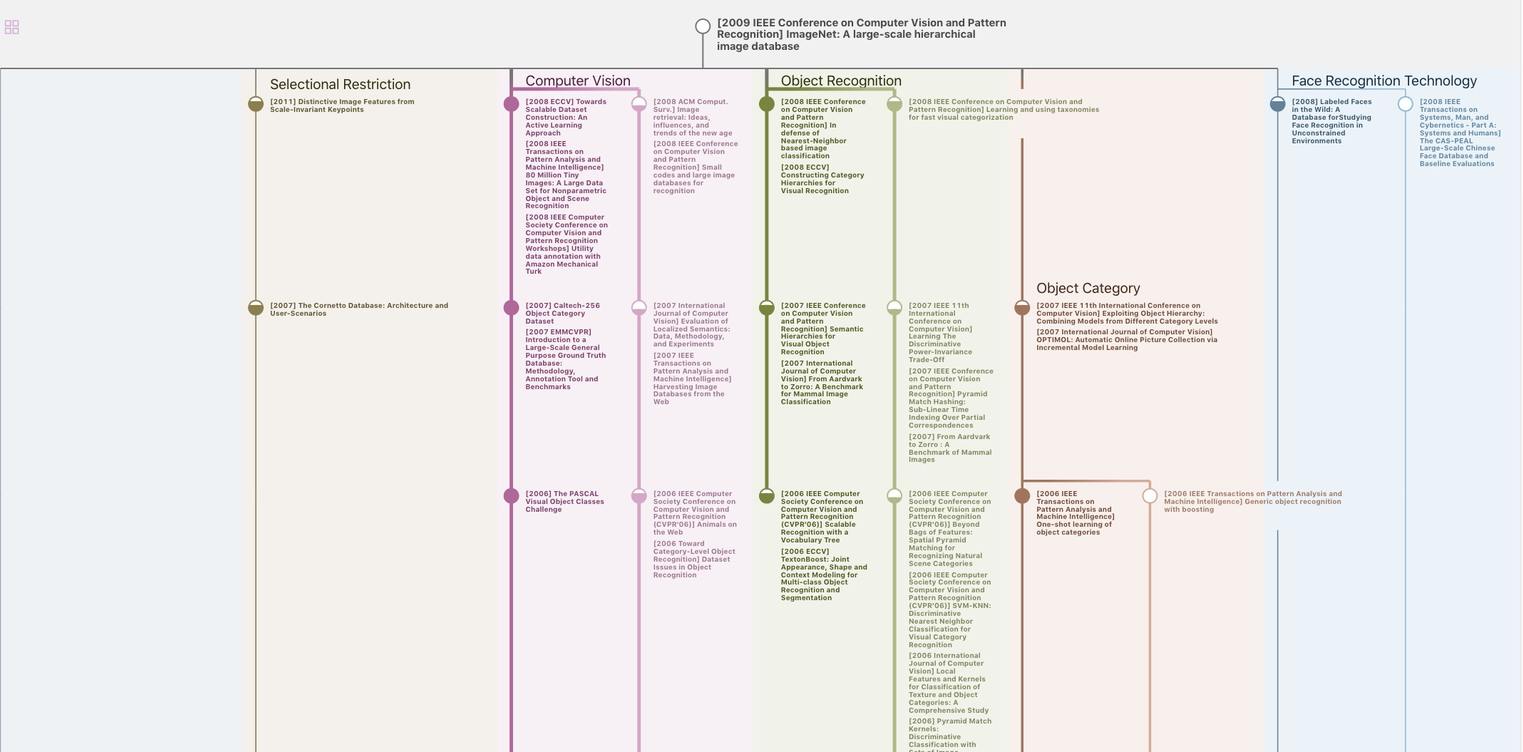
生成溯源树,研究论文发展脉络
Chat Paper
正在生成论文摘要