GFMAE: Self-Supervised GNN-Free Masked Autoencoders
ICASSP 2024 - 2024 IEEE International Conference on Acoustics, Speech and Signal Processing (ICASSP)(2024)
摘要
Generative self-supervised learning, represented by graph autoencoders (GAEs), has begun to exhibit significant potential in addressing graph tasks. However, GAEs often rely on Graph Neural Networks (GNNs) for encoding and decoding, this can pose a computation challenge due to the inherent complexities of the aggregation mechanism in GNNs. Furthermore, the bipartite structure of GAEs introduces additional computational burdens. In contrast, Multi-Layer Perceptrons (MLPs) have no graph dependency and can train much faster than GNNs. Motivated by this, in this work, we introduce a simple yet effective alternative: the GNN-Free Masked AutoEncoder (GFMAE), which employs MLPs rather than GNNs to serve as the backbone model to speed up training. Additionally, we devise comprehensive decoding strategies to compensate for the inability of MLPs in characterizing the graph. Our comprehensive experiments conducted on eight datasets demonstrate that GFMAE achieves performance comparable to GNNs while also enhancing the training efficiency of generative models with GNNs as the backbone.
更多查看译文
关键词
Generative Learning,Autoencoder,Multi-Layer Perceptrons,Self-supervised Learning
AI 理解论文
溯源树
样例
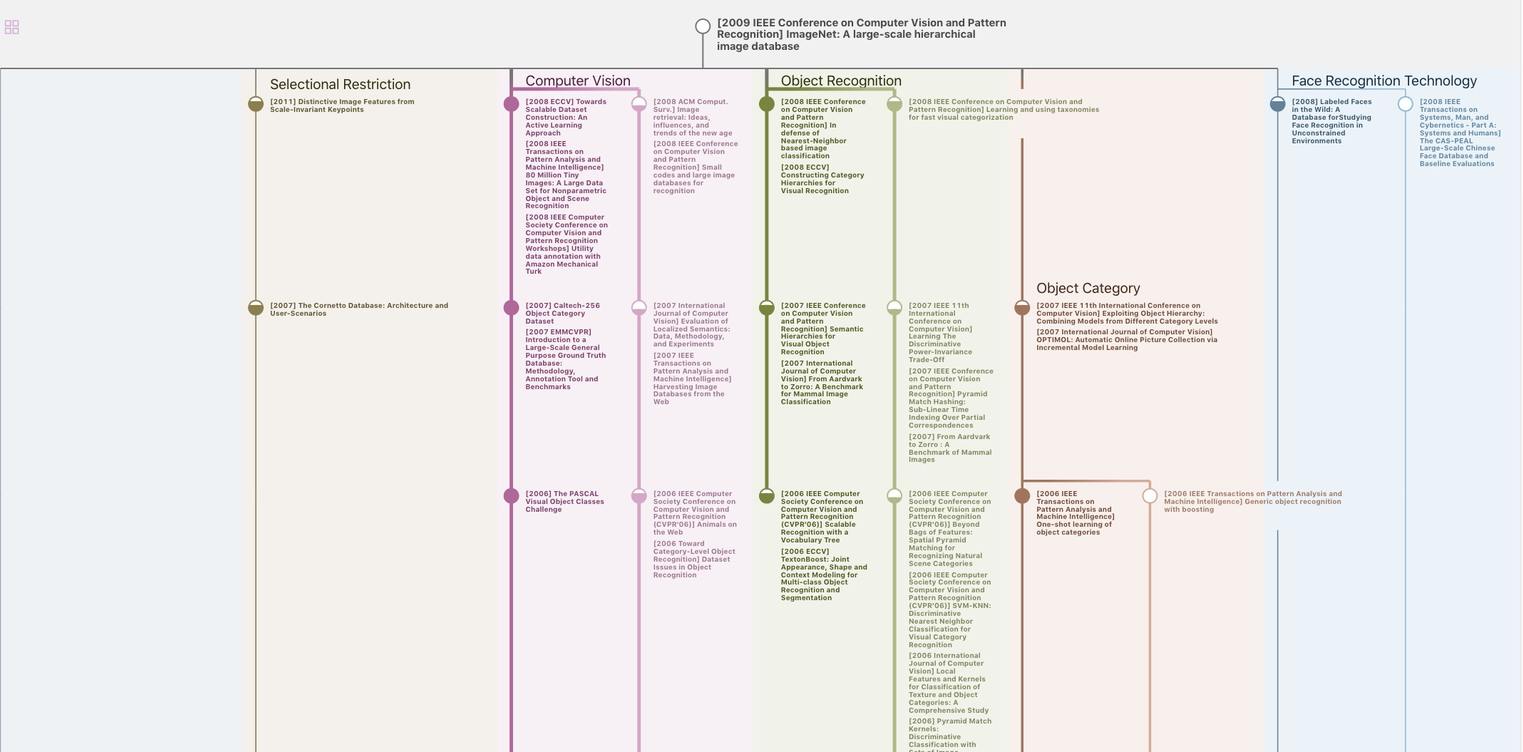
生成溯源树,研究论文发展脉络
Chat Paper
正在生成论文摘要