A Keyless Extraction Framework Targeting at Deep Learning Based Image-Within-Image Models
ICASSP 2024 - 2024 IEEE International Conference on Acoustics, Speech and Signal Processing (ICASSP)(2024)
摘要
Image-within-image technique aims to establish covert communication by concealing a secret image within a cover image. Compared with traditional steganography algorithms, the security of image-within-image technique has not been rigorously evaluated by steganalysis. Existing attack methods just brutally destroy the container image, resulting in the secret image cannot be revealed by the original decryption model (key). This paper introduces a novel keyless extraction framework, carrying out steganalysis on the container image without destroying it. Our approach utilizes collected pairs of container and revealed images to construct a master key, enabling us to extract secret image from container image without relying on the original key. Remarkably, the master key remains effective for multiple image-within-image techniques simultaneously, even when their encryption and decryption models are re-trained. In addition, we propose a patch-based data augmentation technique to adapt to scenarios with limited training samples, and we design a weighted loss function with three components to further enhance the visual quality of the extracted secret image. All the experiments are conducted on datasets derived from ImageNet, COCO and DIV2k. The results demonstrate that our approach can extract secret images with comparable visual quality to the original ones.
更多查看译文
关键词
image-within-image technique,image forensics,keyless extraction framework,a master key
AI 理解论文
溯源树
样例
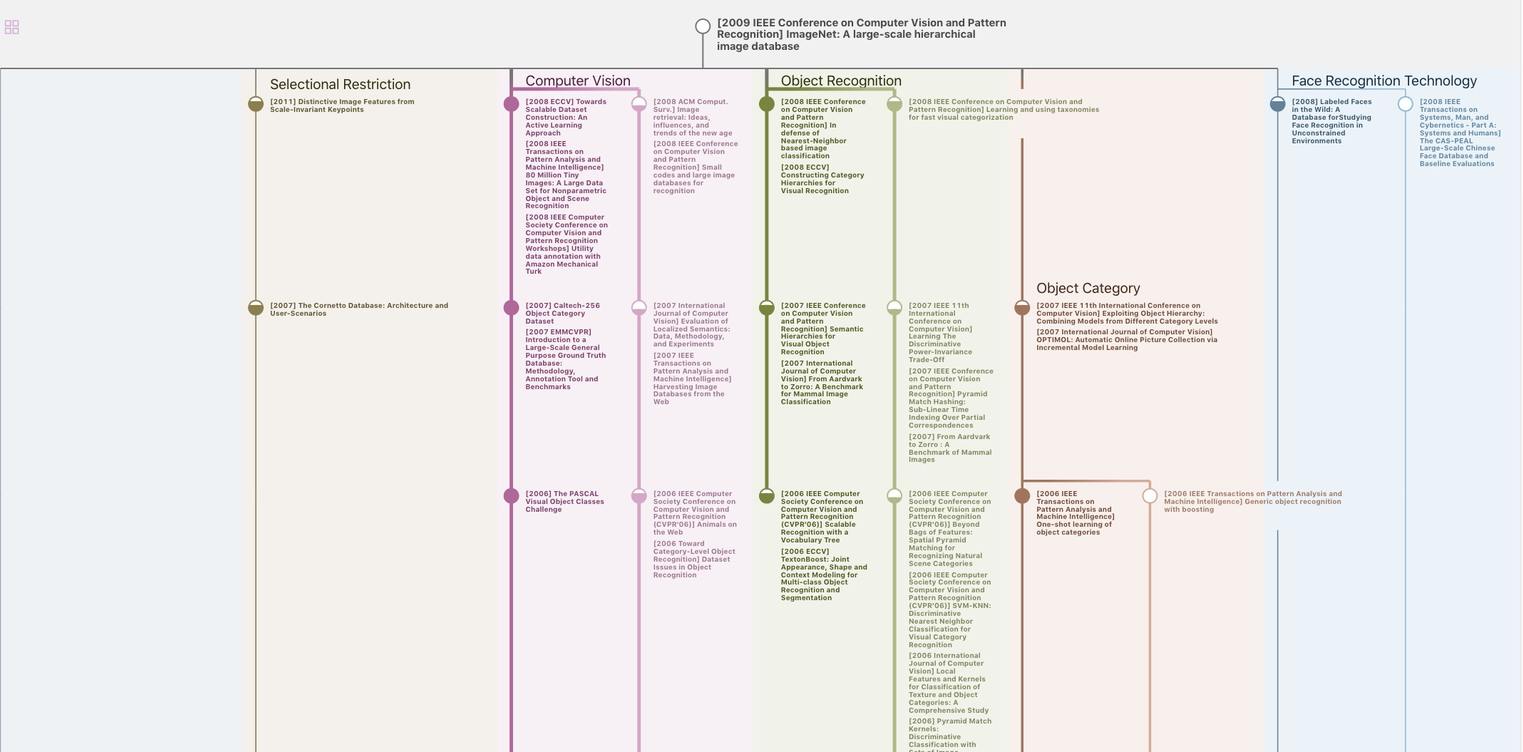
生成溯源树,研究论文发展脉络
Chat Paper
正在生成论文摘要