COLORFLOW: A Conditional Normalizing Flow for Image Colorization
ICASSP 2024 - 2024 IEEE International Conference on Acoustics, Speech and Signal Processing (ICASSP)(2024)
摘要
Image colorization is an ill-posed task, as objects within grayscale images can correspond to multiple colors, motivating researchers to establish a one-to-many relationship between objects and colors. Previous work mostly could only create an insufficient deterministic relationship. Normalizing flow can fully capture the color diversity from natural image manifold. However, classical flow often overlooks the color correlations between different objects, resulting in generating unrealistic color. To solve this issue, we propose a conditional normalizing flow, named ColorFlow, to jointly learn the one-to-many relationships between objects and colors, and the color correlations between different objects within image. To represent these color correlations in flow, we design a color distribution predictor to estimate the global color histogram of grayscale image as global tones, which is utilized as the mean value of flow’s latent variables. Experiments results show that ColorFlow outperforms state-of-the-art methods.
更多查看译文
关键词
Image understanding,Normalizing flow,Colorization
AI 理解论文
溯源树
样例
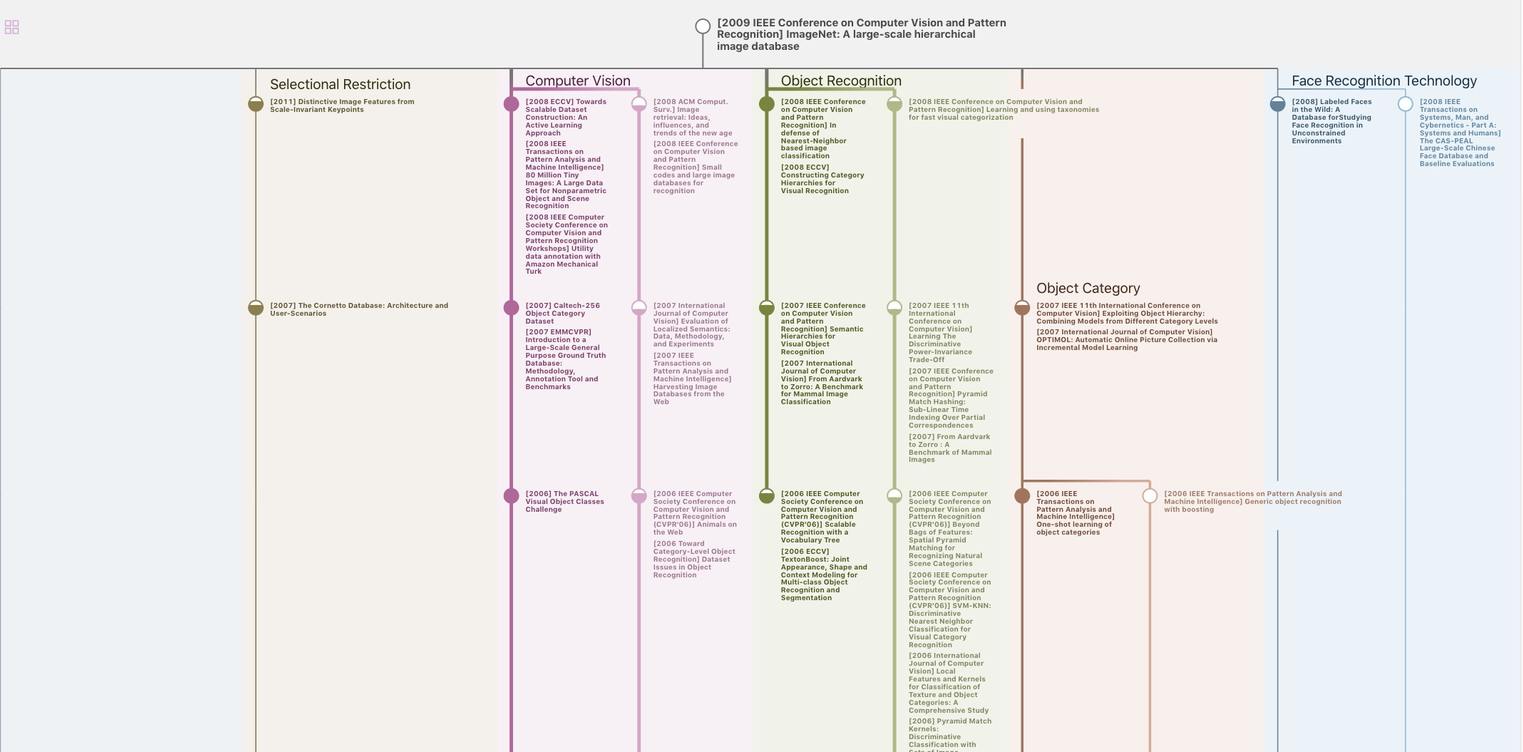
生成溯源树,研究论文发展脉络
Chat Paper
正在生成论文摘要