Inference for New Environmental Contours Using Extreme Value Analysis
Journal of Agricultural Biological and Environmental Statistics(2024)
Key words
Bivariate extremes,Environmental contours,Generalised additive model,Generalised Pareto distribution,Structure function
AI Read Science
Must-Reading Tree
Example
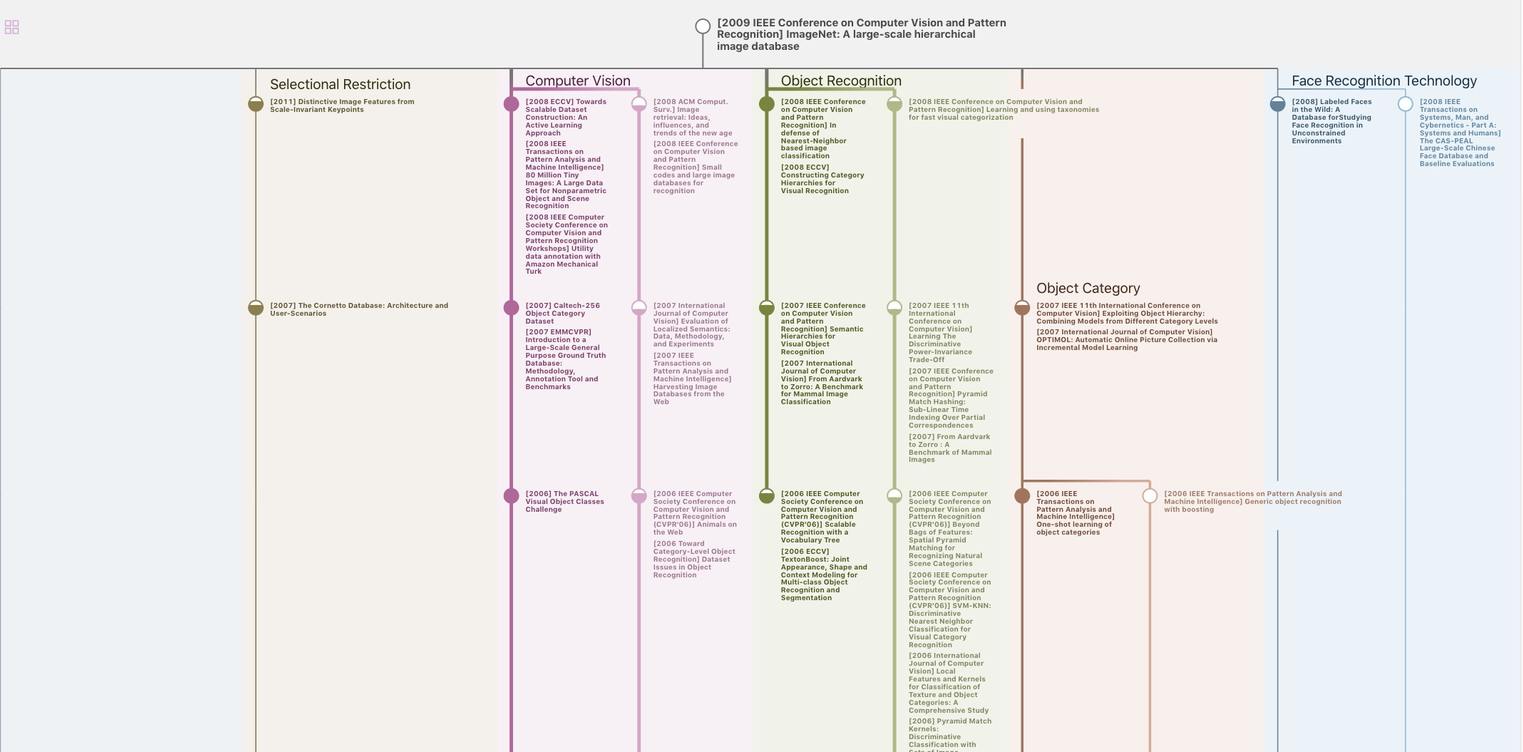
Generate MRT to find the research sequence of this paper
Chat Paper
Summary is being generated by the instructions you defined