Interpretable Face Aging: Enhancing Conditional Adversarial Autoencoders with Lime Explanations
ICASSP 2024 - 2024 IEEE International Conference on Acoustics, Speech and Signal Processing (ICASSP)(2024)
摘要
An innovative approach is proposed that leverages a perturbation explainable system within the Conditional Adversarial Autoencoder (CAAE) framework. The incorporation of the perturbation-based explainable system in the CAAE model harnesses the explanatory power of Local Interpretable Model-Agnostic Explanations (LIME). LIME generates perturbations in the latent space of the CAAE and provides insightful explanations for the discrepancies between fake and real face images. By indicating the areas that contribute most significantly to the aging process, LIME guides the adversarial training process to focus on those aspects, resulting in corrective feedback to the discriminator. The performance of the proposed framework, against state-of-the-art methods, is assessed by objective figures of merit demonstrating superior results in face aging.
更多查看译文
关键词
Face Aging,Explainable Artificial Intelligence,Generative Adversarial Networks
AI 理解论文
溯源树
样例
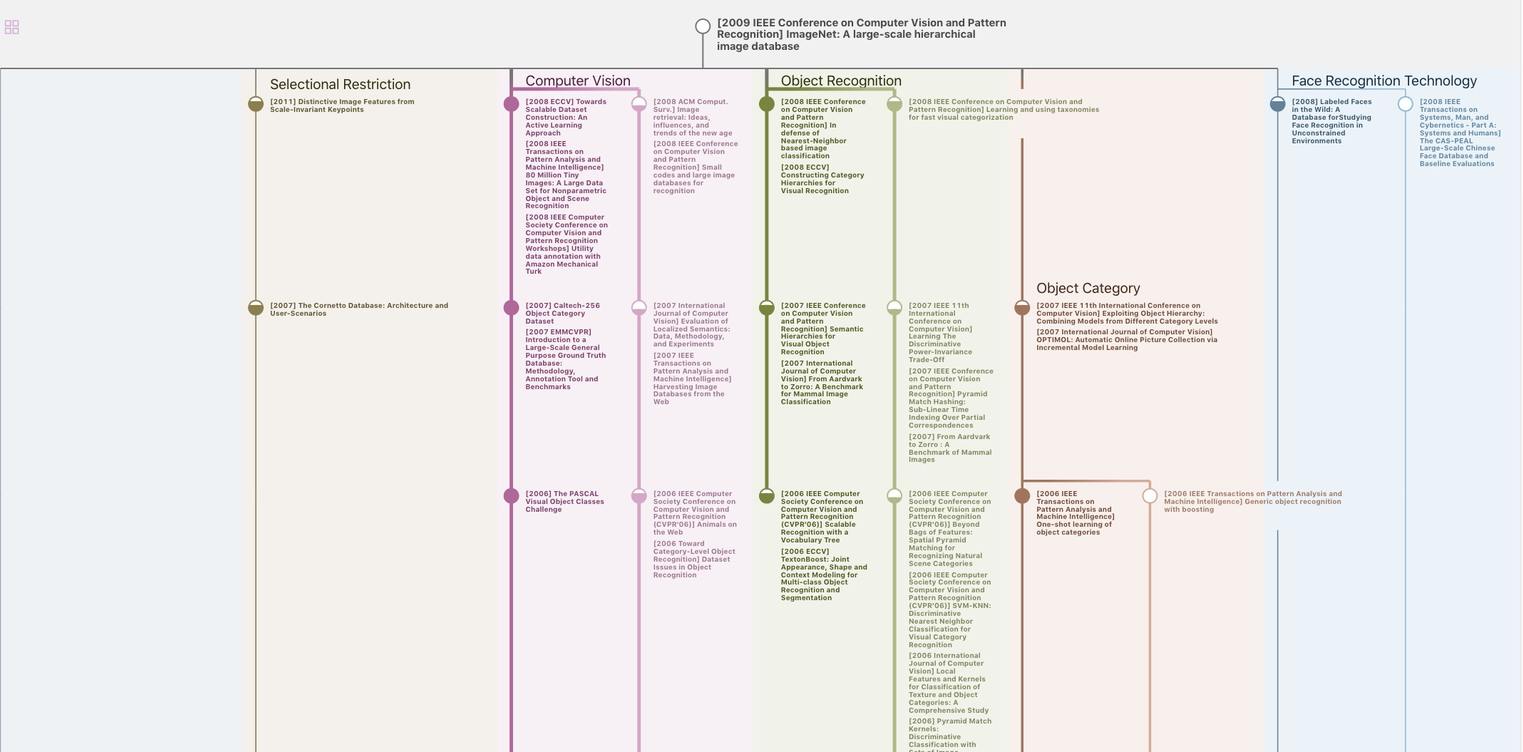
生成溯源树,研究论文发展脉络
Chat Paper
正在生成论文摘要