AEAM3D:Adverse Environment-Adaptive Monocular 3D Object Detection via Feature Extraction Regularization
ICASSP 2024 - 2024 IEEE International Conference on Acoustics, Speech and Signal Processing (ICASSP)(2024)
摘要
3D object detection plays a crucial role in intelligent vision systems. Detection in the open world inevitably encounters various adverse scenes while most of existing methods fail in these scenes. To address this issue, this paper proposes a monocular 3D detection model, termed AEAM3D, which effectively mitigates the degradation of detection performance in various harsh environments. Additionally, we assemble a new adverse 3D object detection dataset encompassing some challenging scenes, including rainy, foggy, and low light weather conditions. Experimental results demonstrate that our proposed method outperforms current state-of-the-art approaches by an average of 3.12% in terms of AP
R40
for car category across adverse environments.
更多查看译文
关键词
3D object detection,monocular vision,image enhancement
AI 理解论文
溯源树
样例
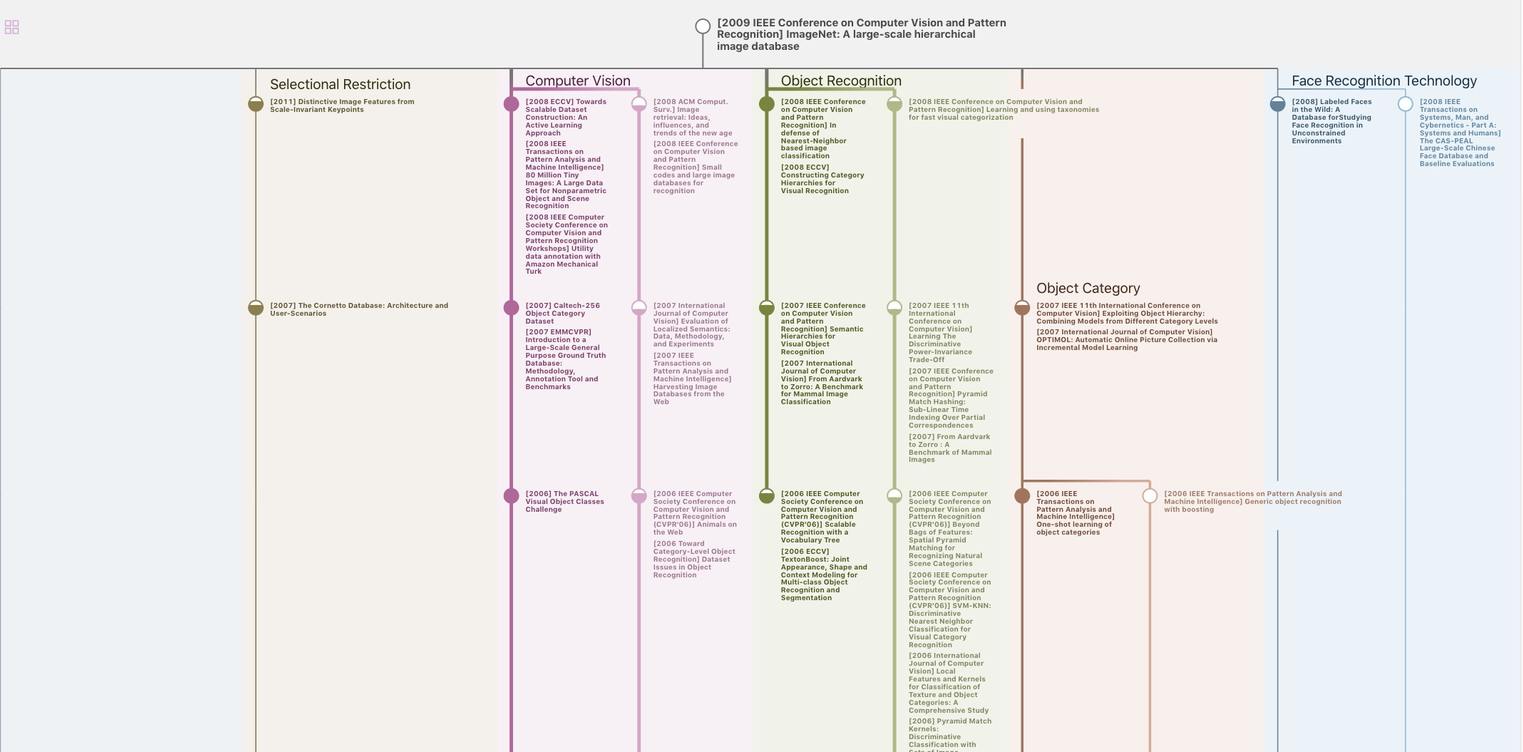
生成溯源树,研究论文发展脉络
Chat Paper
正在生成论文摘要