Prompting Label Efficiency in Federated Graph Learning Via Personalized Semi-Supervision
ICASSP 2024 - 2024 IEEE International Conference on Acoustics, Speech and Signal Processing (ICASSP)(2024)
摘要
Federated graph learning (FGL) enables the collaborative training of graph neural networks (GNNs) in a distributed manner. A critical challenge in FGL is label deficiency, which becomes more intricate due to non-IID decentralized data. Existing methods have focused on extracting knowledge from abundant unlabeled data, leaving few-shot labeled data unexplored. To this end, we propose ConFGL, a novel FGL framework to enhance label efficiency in federated learning with non-IID subgraphs. We formulate a semi-supervised objective to harness both unlabeled and labeled data, where self-supervised learning is achieved via a graph contrastive module. Additionally, a personalized federated learning (FL) strategy is adopted to concurrently train a global model and an individual model, which helps alleviate the representation disparities encoded by local models. Extensive experiments on four node-level datasets under non-IID settings have shown that ConFGL can consistently provide an average of 4.10% accuracy gains over supervised FL personalization methods while maintaining a higher GPU throughput.
更多查看译文
关键词
Personalized Federated Learning,Graph Neural Networks,Label Deficiency,Contrastive Learning
AI 理解论文
溯源树
样例
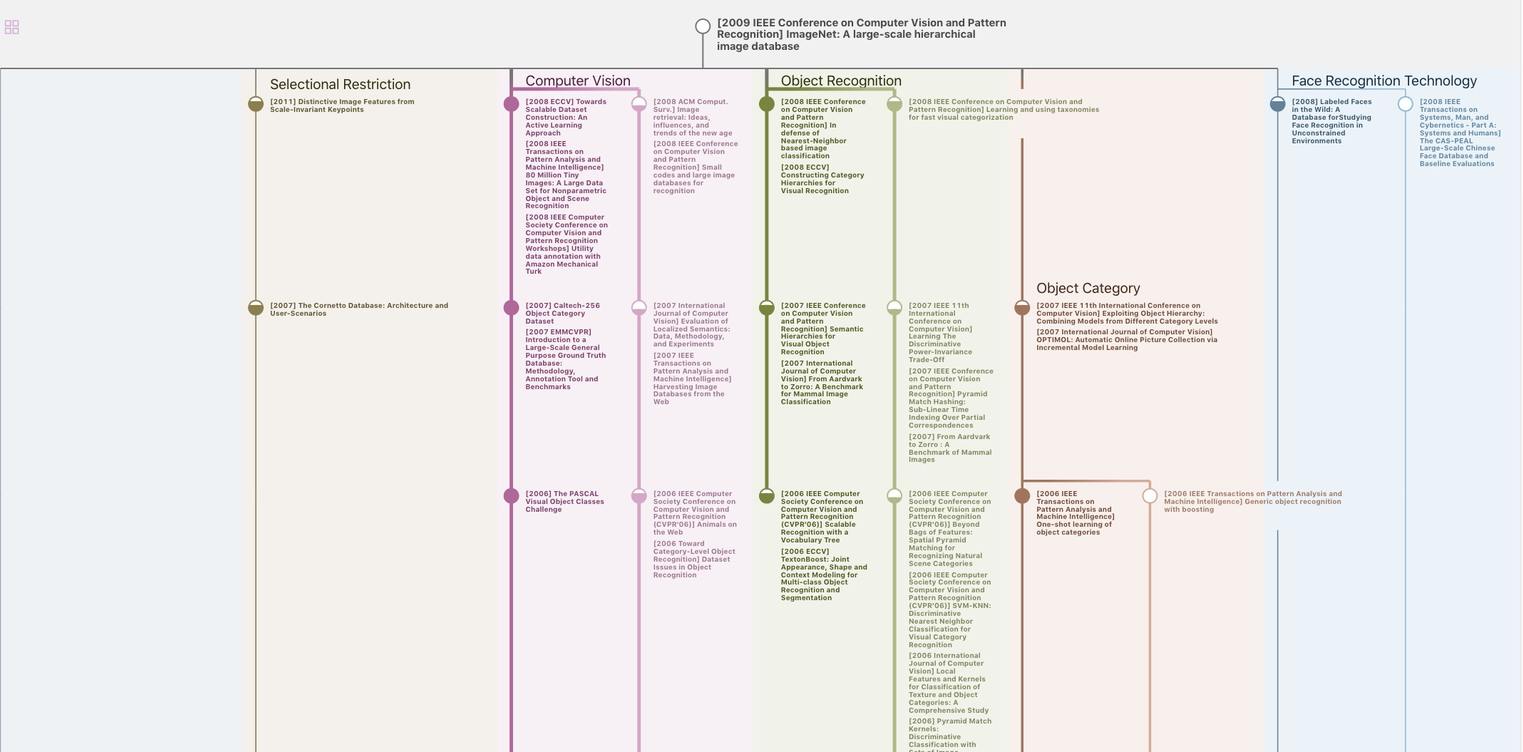
生成溯源树,研究论文发展脉络
Chat Paper
正在生成论文摘要