Whisper-Based Transfer Learning for Alzheimer Disease Classification: Leveraging Speech Segments with Full Transcripts as Prompts
ICASSP 2024 - 2024 IEEE International Conference on Acoustics, Speech and Signal Processing (ICASSP)(2024)
Abstract
Alzheimer’s disease (AD) is a neurodegenerative disorder that can lead to speech impairments. Early diagnosis is crucial for effective treatment, and speech-based diagnosis is currently a hot research topic. In this study, we explore the feasibility of transfer learning for Alzheimer’s disease detection using the state-of-the-art multilingual speech recognition and translation model: Whisper. In order to address the limitation of Whisper’s narrow perspective caused by the restricted audio segment length during fine-tuning, we propose an innovative method to overcome this problem by using the full transcript as a prompt to assist in training speech segments. This approach results in a relative performance improvement of 9%-12% for models with a higher number of parameters. On the ADReSSo test set, the accuracy and F1 score achieved are 84.51% and 84.50% respectively, surpassing both the baseline system and commonly used speech recognition-language model cascade methods, demonstrating its effectiveness.
MoreTranslated text
Key words
Whisper,prompt,transfer learning,Alzheimer’s Disease classification
AI Read Science
Must-Reading Tree
Example
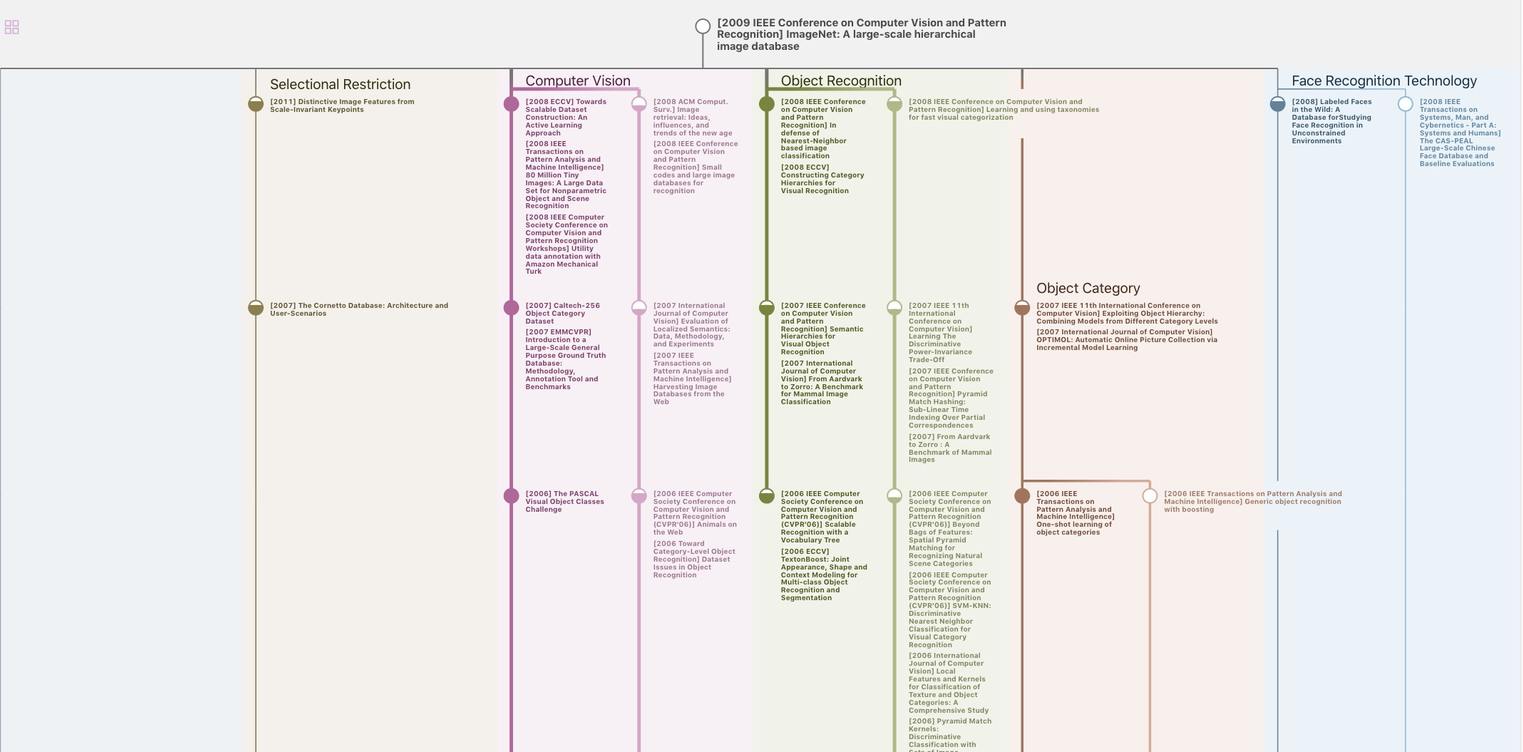
Generate MRT to find the research sequence of this paper
Chat Paper
Summary is being generated by the instructions you defined