Automatic High-Performance Neural Network Construction for Channel Estimation in IRS-Aided Communications
IEEE Transactions on Wireless Communications(2024)
摘要
Accurate channel estimation is an essential prerequisite for achieving significant performance gains in intelligent reflecting surface (IRS)-aided communication systems. Recent studies have shown that deep neural network-based channel estimation holds promise as a competitive alternative to conventional methods. However, existing neural network-based approaches typically involve manual design of network architectures through a trial-and-error process, demanding extensive domain knowledge and human resources. In this paper, we propose an automatic approach to construct a high-performance neural network architecture for channel estimation. Our method, called the channel estimation neural network architecture search (CENAS), utilizes a truncated back-propagation optimization search strategy to explore a neural network tailored for channel estimation. By carefully designing a search space tailored to channel estimation tasks, the automatically constructed network surpasses both conventional and deep learning-based channel estimation algorithms. The convergence of our framework’s network construction process is comprehensively analyzed, providing formal evidence of its convergence properties. Additionally, the proposed framework exhibits good generalization and applicability by allowing flexible adjustment of hyperparameters to generate networks with varying scales. Empirical results show the stability and the improved performance of CENAS framework, validating its effectiveness and desirability.
更多查看译文
关键词
Intelligent reflecting surface,channel estimation,neural network architecture search,deep learning
AI 理解论文
溯源树
样例
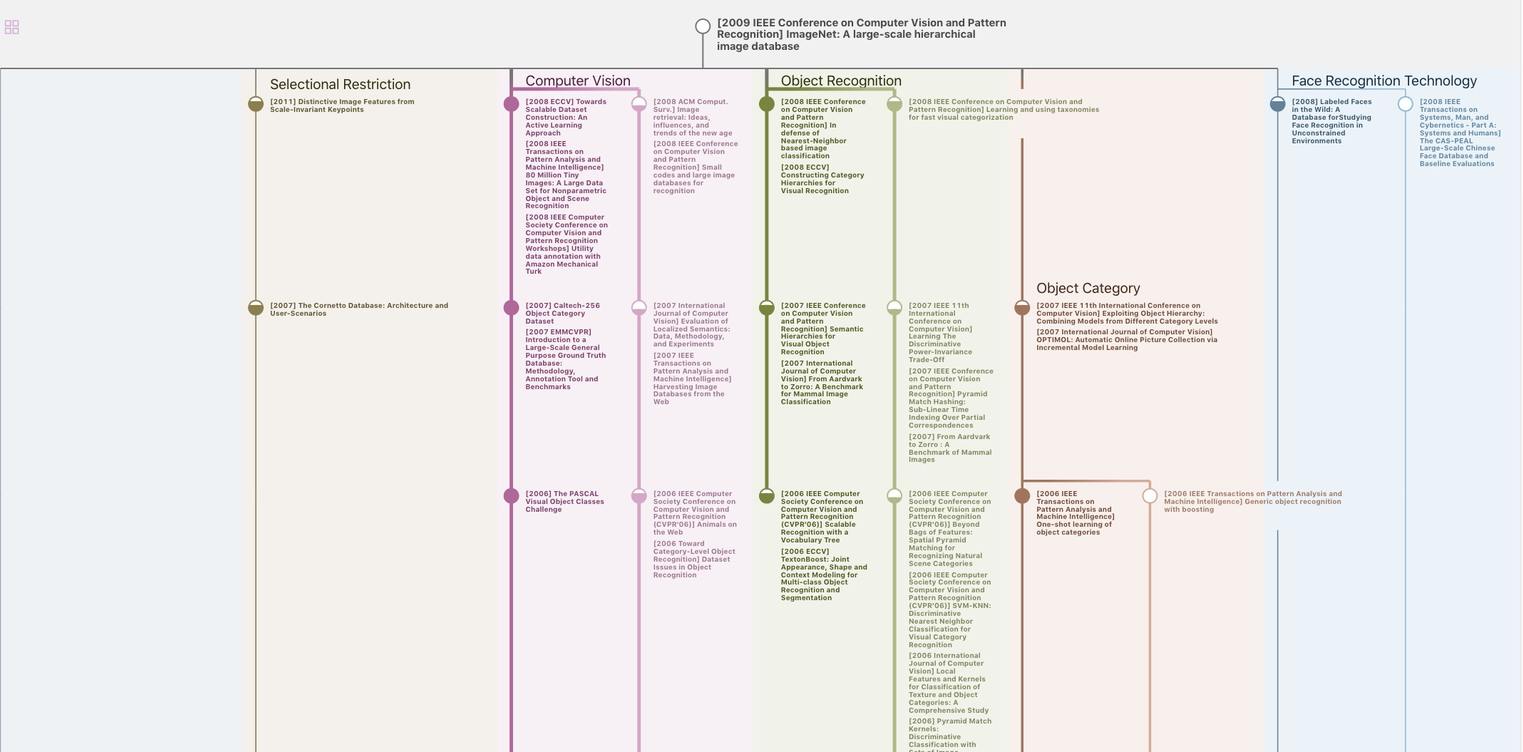
生成溯源树,研究论文发展脉络
Chat Paper
正在生成论文摘要