Motion Transfer-Driven Intra-Class Data Augmentation for Finger Vein Recognition
ICASSP 2024 - 2024 IEEE International Conference on Acoustics, Speech and Signal Processing (ICASSP)(2024)
摘要
Finger vein recognition (FVR) has emerged as a secure biometric technique because of the confidentiality of vascular bio-information. Recently, deep learning-based FVR has gained increased popularity and achieved promising performance. However, the limited size of public vein datasets has caused overfitting issues and greatly limits the recognition performance. Although traditional data augmentation can partially alleviate this data shortage issue, it cannot capture the real finger posture variations due to the rigid label-preserving image transformations, bringing limited performance improvement. To address this issue, we propose a novel motion transfer (MT) model for finger vein image data augmentation via modeling the actual finger posture and rotational movements. The proposed model first utilizes a key point detector to extract the key point and pose map of the source and drive finger vein images. We then utilize a dense motion module to estimate the motion optical flow, which is fed to an image generation module for generating the image with the target pose. Experiments conducted on three public finger vein databases demonstrate that the proposed motion transfer model can generate realistic intra-class augmented samples and effectively improve the recognition accuracy.
更多查看译文
关键词
Vascular biometrics,Motion transfer,Data augmentation,Self-supervised learning
AI 理解论文
溯源树
样例
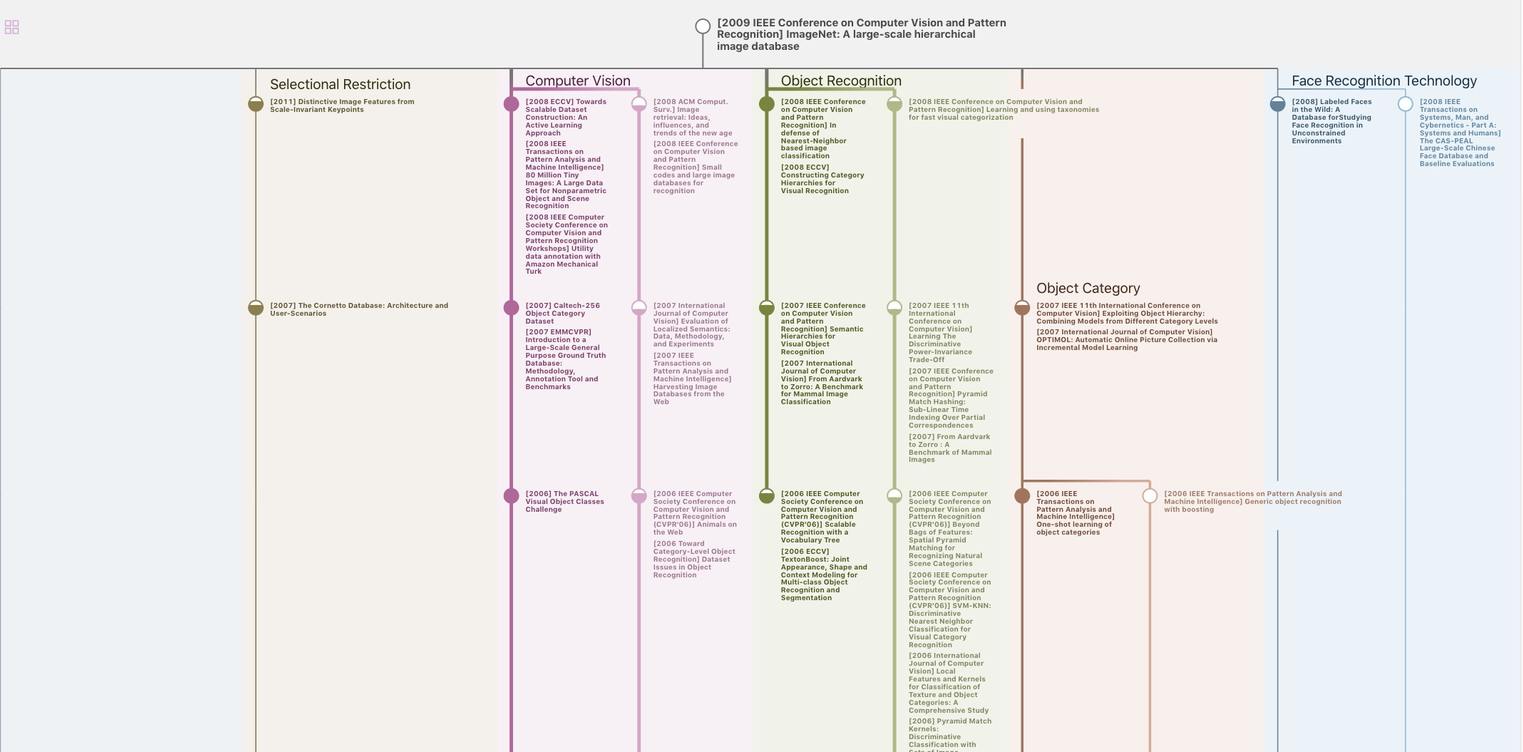
生成溯源树,研究论文发展脉络
Chat Paper
正在生成论文摘要