Causality-Inspired Single-Source Domain Generalization for Face Anti-Spoofing.
IEEE International Conference on Acoustics, Speech, and Signal Processing(2024)
摘要
Most face anti-spoofing methods address the generalization problem by extracting domain-invariant representations from multiple source domains or unlabelled target data. However, their deployment in real-world applications is unfeasible when data is insufficient or unavailable due to the collection costs and privacy concerns. This work investigates a more practical yet challenging scenario: single-source domain generalization based face anti-spoofing, where only one source domain is available during training and evaluated on multiple unseen target domains. To tackle this problem, a Causality-inspired Single-source Domain Generalization method (CSDG) is developed, which focuses on learning causal spoofing representations from the causality perspective. Specifically, a causal diagram is constructed to estimate the fundamental properties of ideal causal spoofing representations: remain invariant to shifts of domain-related confounders and causally sufficient for the detection category. To satisfy the above properties, the Causal Learning Module (CLM) maximizes the correlation of representations before and after intervention and minimizes the correlation with negative distributions. The intervention is achieved by arbitrarily performing spectrum mixup and structure destruction on source data within the Causal Intervention Model (CIM). Extensive experiments on four benchmark datasets validate the effectiveness of the proposed method.
更多查看译文
关键词
Face anti-spoofing,single-source domain generalization,causal learning
AI 理解论文
溯源树
样例
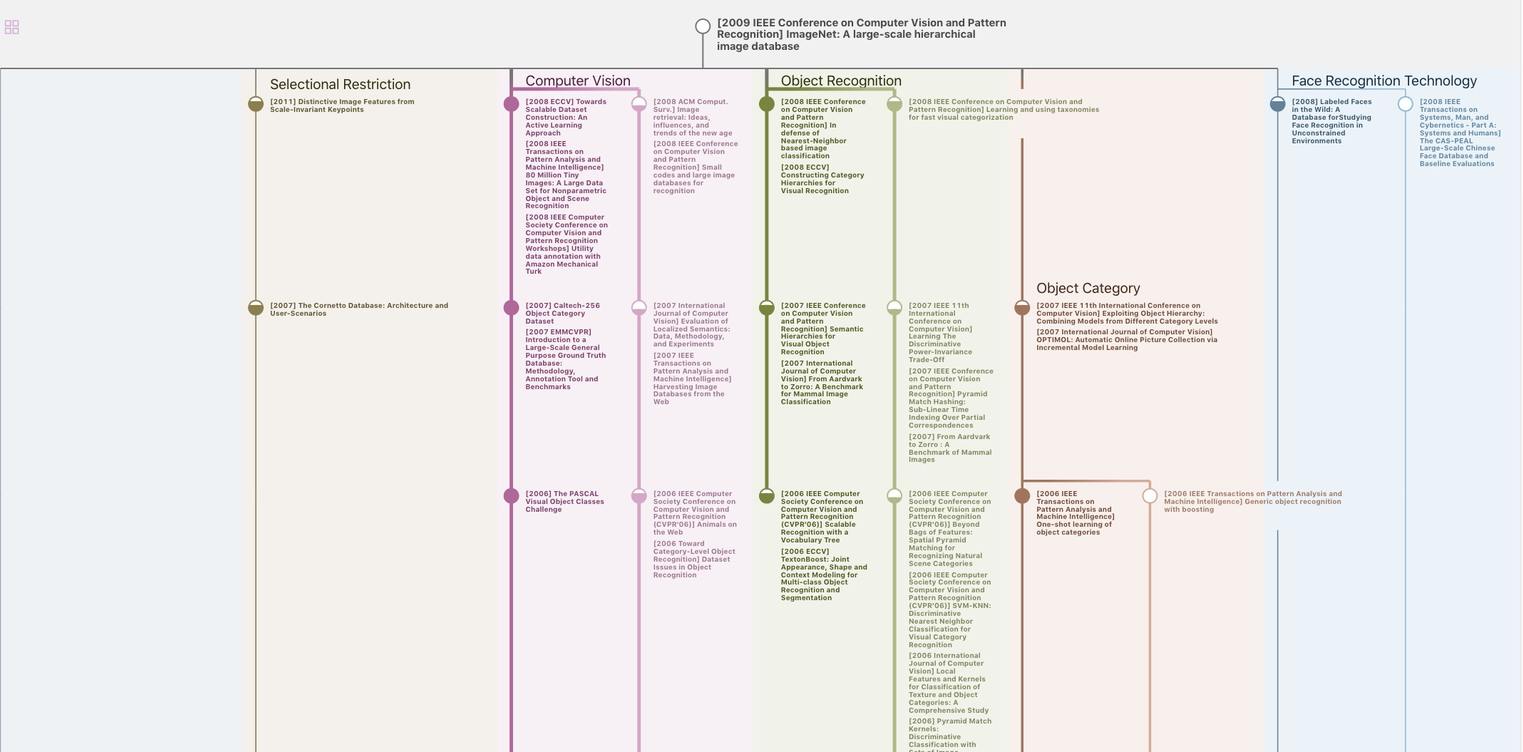
生成溯源树,研究论文发展脉络
Chat Paper
正在生成论文摘要