On the Convergence of Single-Timescale Multi-Sequence Stochastic Approximation Without Fixed Point Smoothness
ICASSP 2024 - 2024 IEEE International Conference on Acoustics, Speech and Signal Processing (ICASSP)(2024)
摘要
Stochastic approximation (SA) that involves multiple coupled sequences has diverse applications, including but not limited to bilevel optimization, meta learning and reinforcement learning. Unfortunately, the existing multi-timescale analysis of multiple-sequence SA (MSSA) implies a slow convergence rate, whereas the single-timescale analysis relies on assuming smoothness of fixed points. In this paper, we present tighter single-timescale analysis for MSSA, without assuming smoothness of fixed points. Our theoretical results demonstrate that, when all involved operators are strongly monotone, MSSA converges at a rate of $\tilde {\mathcal{O}}\left( {{K^{ - 1}}} \right)$, where K is the total number of iterations. Under a weaker assumption that all involved operators are strongly monotone except for$O\left( {{K^{ - \frac{1}{2}}}} \right)$ the main one, MSSA converges at a rate of . These theoretical results align with those established in single-sequence SA (SSSA). Applying these theoretical results to bilevel optimization offers relaxed assumptions and/or simpler algorithms with performance guarantees, as validated by numerical experiments.
更多查看译文
关键词
Stochastic approximation (SA),convergence analysis,bilevel optimization
AI 理解论文
溯源树
样例
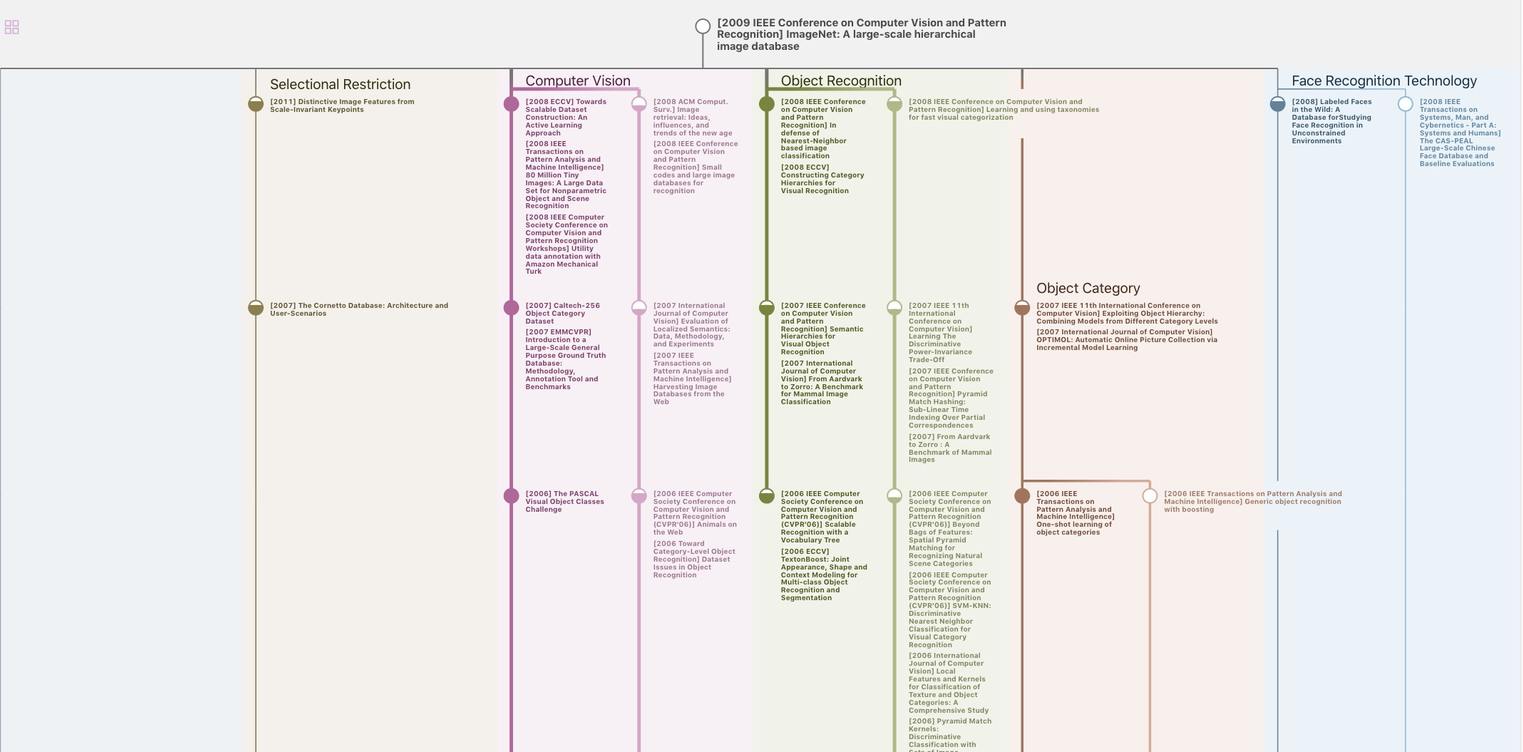
生成溯源树,研究论文发展脉络
Chat Paper
正在生成论文摘要