Dual Parameter-Efficient Fine-Tuning for Speaker Representation Via Speaker Prompt Tuning and Adapters
IEEE International Conference on Acoustics, Speech, and Signal Processing(2024)
Key words
Speaker verification,parameter-efficient tuning,prompt tuning,Transformer adapter,pre-trained Transformer
AI Read Science
Must-Reading Tree
Example
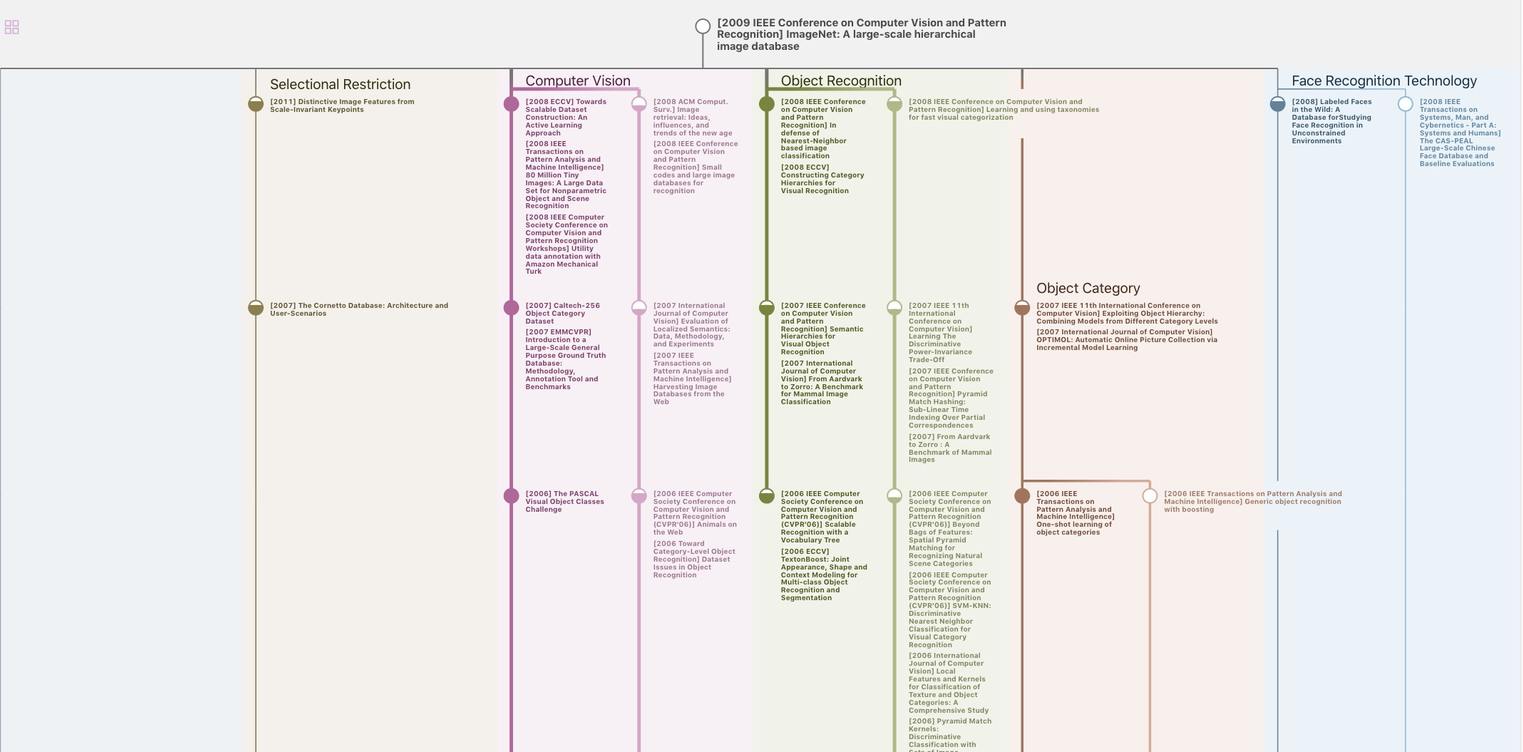
Generate MRT to find the research sequence of this paper
Chat Paper
Summary is being generated by the instructions you defined