KN-SLAM: Keypoints and Neural Implicit Encoding SLAM.
IEEE Trans. Instrum. Meas.(2024)
Abstract
In recent years, the combination of neural implicit representations with Simultaneous Localization and Mapping (SLAM) has shown promising advancements. Nevertheless, the existing methods suffer from drawbacks including poor localization accuracy and the absence of loop closure modules, resulting in suboptimal localization accuracy and issues such as ghosting and blurring in the reconstruction. To overcome these challenges, we propose a novel architecture “Keypoints and Neural implicit encoding SLAM” (KN-SLAM), a combination of feature-based localization and neural implicit representations for mapping, which aims to achieve better reconstruction quality while ensuring high localization accuracy. Moreover, we leverage global and local features to achieve loop closure detection and global optimization, which can further reduce cumulative errors. Comprehensive experiments demonstrate that KN-SLAM can achieve the competitive performance in both map quality and localization accuracy.
MoreTranslated text
Key words
SLAM,Neural Implicit Encoding,Keypoints,Localization,Reconstruction
AI Read Science
Must-Reading Tree
Example
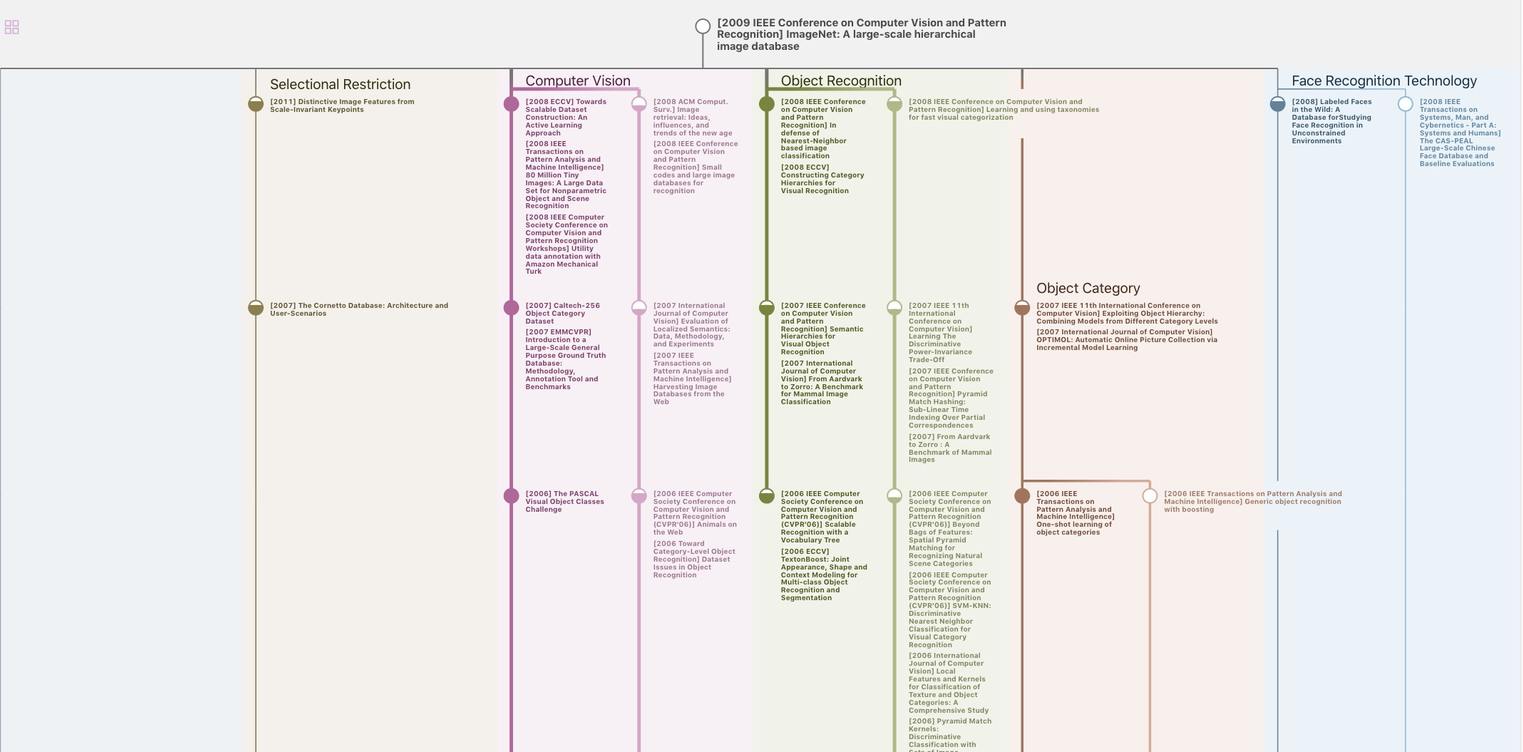
Generate MRT to find the research sequence of this paper
Chat Paper
Summary is being generated by the instructions you defined