Multiway-Adapter: Adapting Multimodal Large Language Models for Scalable Image-Text Retrieval
ICASSP 2024 - 2024 IEEE International Conference on Acoustics, Speech and Signal Processing (ICASSP)(2024)
摘要
As Multimodal Large Language Models (MLLMs) grow in size, adapting them to specialized tasks becomes increasingly challenging due to high computational and memory demands. Indeed, traditional fine-tuning methods are costly, due to the need for extensive, task-specific training. While efficient adaptation methods exist that aim to reduce these costs, in practice they suffer from shallow inter-modal alignment, which severely hurts model effectiveness. To tackle these computational challenges and improve inter-modal alignment, we introduce the MultiWay-Adapter (MWA), a novel framework featuring an ‘Alignment Enhancer’. This enhancer deepens inter-modal alignment, enabling high transferability with minimal tuning effort. Our experiments show that unlike prior efficient tuning approaches, MWA maintains model effectiveness, while reducing training time by up-to 57%. MWA is also lightweight, increasing model size by only 2-3% (in terms of parameters) for state-of-the-art foundation models like BEiT-3 Large. These results demonstrate that MWA provides an efficient and effective adaptation method for MLLMs, significantly broadening their applicability.
更多查看译文
关键词
Multimodal Large Language Models,Image-Text Retrieval,Adapter,Transformers,Transfer Learning
AI 理解论文
溯源树
样例
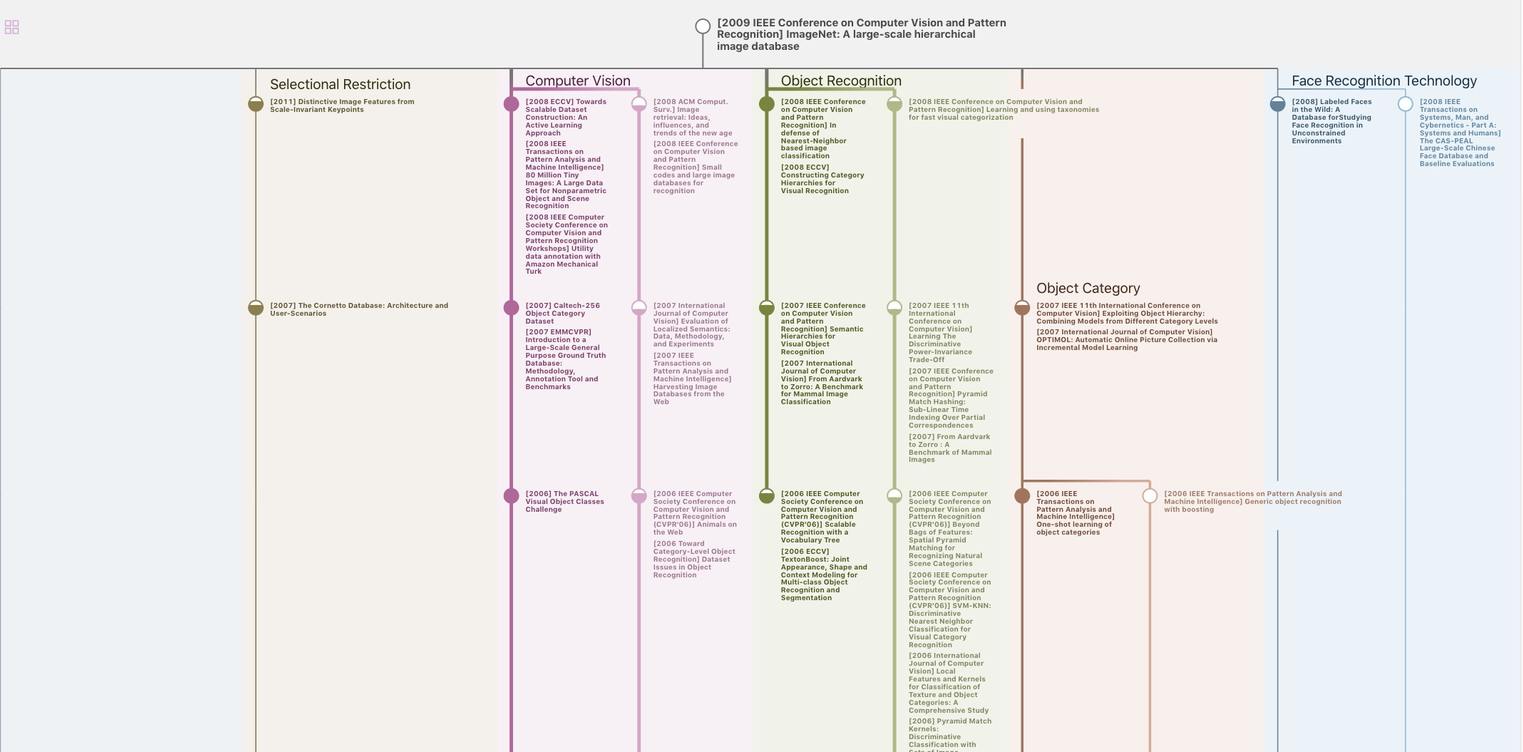
生成溯源树,研究论文发展脉络
Chat Paper
正在生成论文摘要