KC-Prompt: End-To-End Knowledge-Complementary Prompting for Rehearsal-Free Continual Learning.
IEEE International Conference on Acoustics, Speech, and Signal Processing(2024)
Abstract
Continuous learning requires adapting quickly to incoming tasks while avoiding catastrophic forgetting. Typical solutions resort to a rehearsal buffer to replay old data, which is intractable to apply in real-world scenarios with limited memory and inaccessible privacy. Recently, with the emergence of large-scale pre-trained models, prompting methods have rapidly become a popular rehearsal-free alternative to rehearsal-based methods. The core of prmopting is to encode knowledge leveraging a set of parameters, however, knowledge decoupling and complementarity still remain some challenges. To tackle these challenges, this paper presents a KnowledgeComplementary Prompting approach, KC-Prompt, which end-to-end integrates and releases the task-invariant and task-specific knowledge for the ViT backbone. KC-Prompt designs knowledge maintenance and knowledge sharing mechanisms to form complementary prompt generators. In addition, we employ a components weighting method to instantiate prompt generators, making the training process fully differentiable. Sufficient experiments on CIFAR-100 and Split ImageNet-R benchmarks demonstrate the superiority of KC-Prompt in the challenging and realistic class-incremental learning setting.
MoreTranslated text
Key words
continual learning,incremental learning,catastrophic forgetting,stability-plasticity dilemma,prompt
AI Read Science
Must-Reading Tree
Example
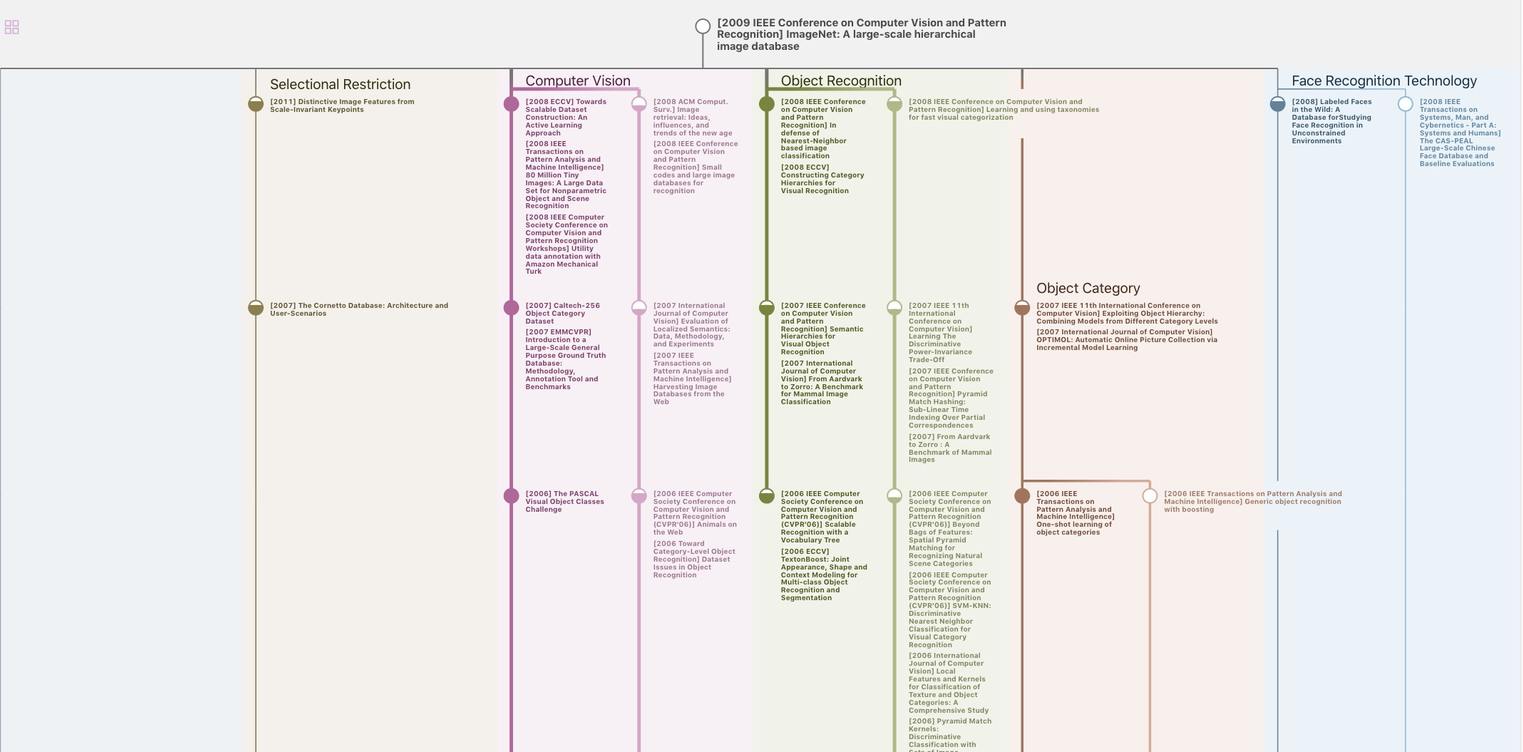
Generate MRT to find the research sequence of this paper
Chat Paper
Summary is being generated by the instructions you defined