The Double-Edged Sword Of Ai Safety: Balancing Anomaly Detection and OOD Generalization Via Model Anchoring.
IEEE International Conference on Acoustics, Speech, and Signal Processing(2024)
Abstract
Safe deployment of AI systems requires models to accurately flag anomalous or semantically unrelated data, while also generalizing to unseen shifts in the data distribution. While both these problems have been extensively studied, there is a risk for undesirable trade-off when exclusively optimizing for one of the objectives. In this paper, we systematically study this trade-off under the lens of model anchoring. Anchoring is a recently proposed training methodology that involves reparameterizing input data into anchor-residual pairs (anchors are drawn from the training data itself), thus establishing a combinatorial relationship with other samples in the training data. We make a surprising finding that the dual objectives of generalization and anomaly detection can be controlled by independently regularizing the model’s dependency on the distribution of anchors and residuals respectively. This enables, for the first time, a finer control of the detection-generalization trade-off without requiring any additional data (e.g., outlier exposure) or computationally intensive modeling strategies (e.g., deep ensembling).
MoreTranslated text
Key words
Anomaly Detection,OOD Generalization,Anchoring,Deep Neural Networks,Augmentations
AI Read Science
Must-Reading Tree
Example
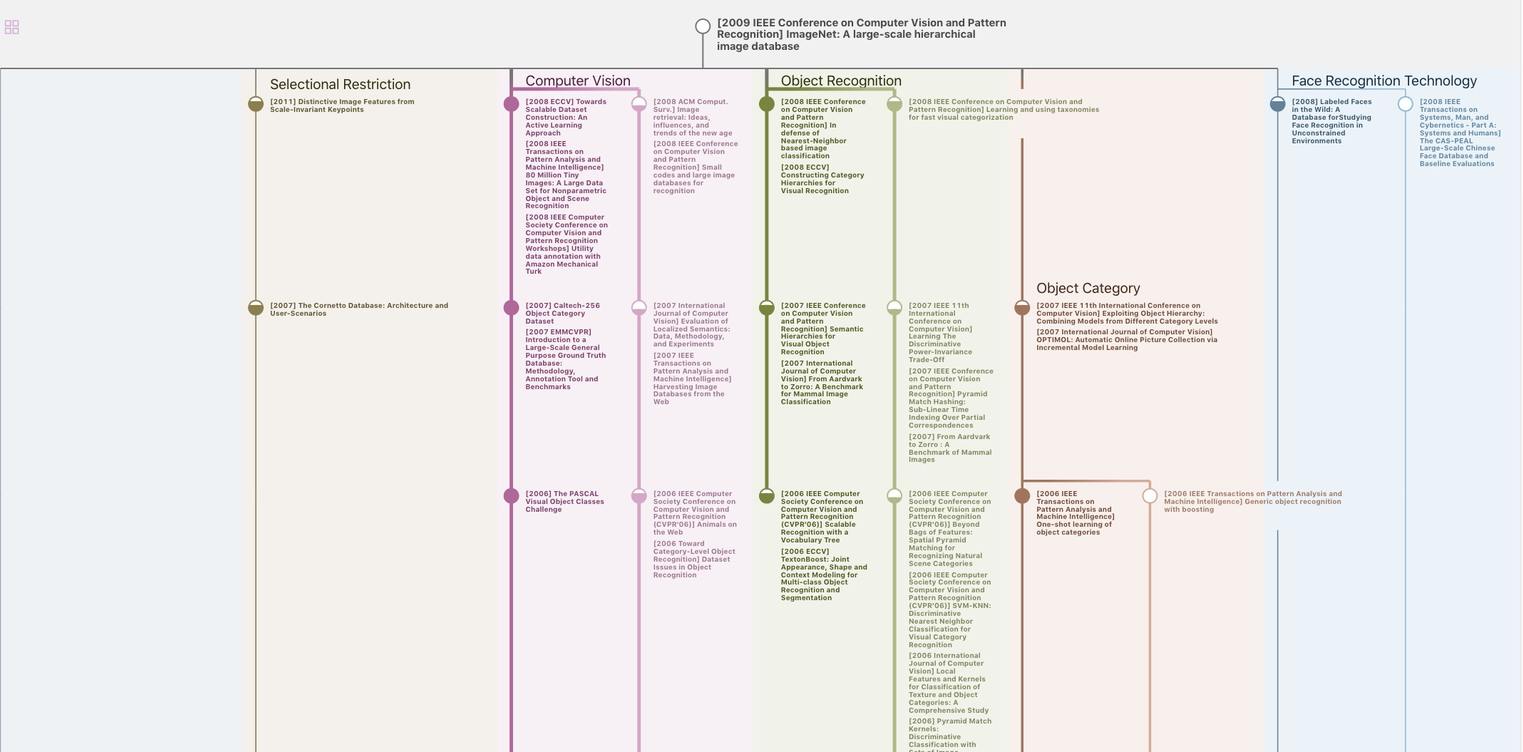
Generate MRT to find the research sequence of this paper
Chat Paper
Summary is being generated by the instructions you defined