Let the Laser Beam Connect the Dots: Forecasting and Narrating Stock Market Volatility
INFORMS JOURNAL ON COMPUTING(2024)
摘要
Forecasting market volatility, especially high-volatility incidents, is a critical issue in financial market research and practice. Business news as an important source of market information is often exploited by artificial intelligence-based volatility forecasting models. Computationally, deep learning architectures, such as recurrent neural networks, on extremely long input sequences remain infeasible because of time complexity and memory limitations. Meanwhile, understanding the inner workings of deep neural networks is challenging because of the largely black box nature of large neural networks. In this work, we address the first challenge by proposing a long- and short-term memory retrieval (LASER) architecture with flexible memory and horizon configurations to forecast market volatility. Then, we tackle the interpretability issue by devising a BEAM algorithm that leverages a large pretrained language model (GPT-2). It generates human-readable narratives verbalizing the evidence leading to the model prediction. Experiments on a Wall Street Journal news data set demonstrate the superior performance of our proposed LASERBEAM pipeline in predicting high-volatility market scenarios and generating high-quality narratives compared with existing methods in the literature.
更多查看译文
关键词
forecasting,memory retrieval,narrative generation,mode interpretability
AI 理解论文
溯源树
样例
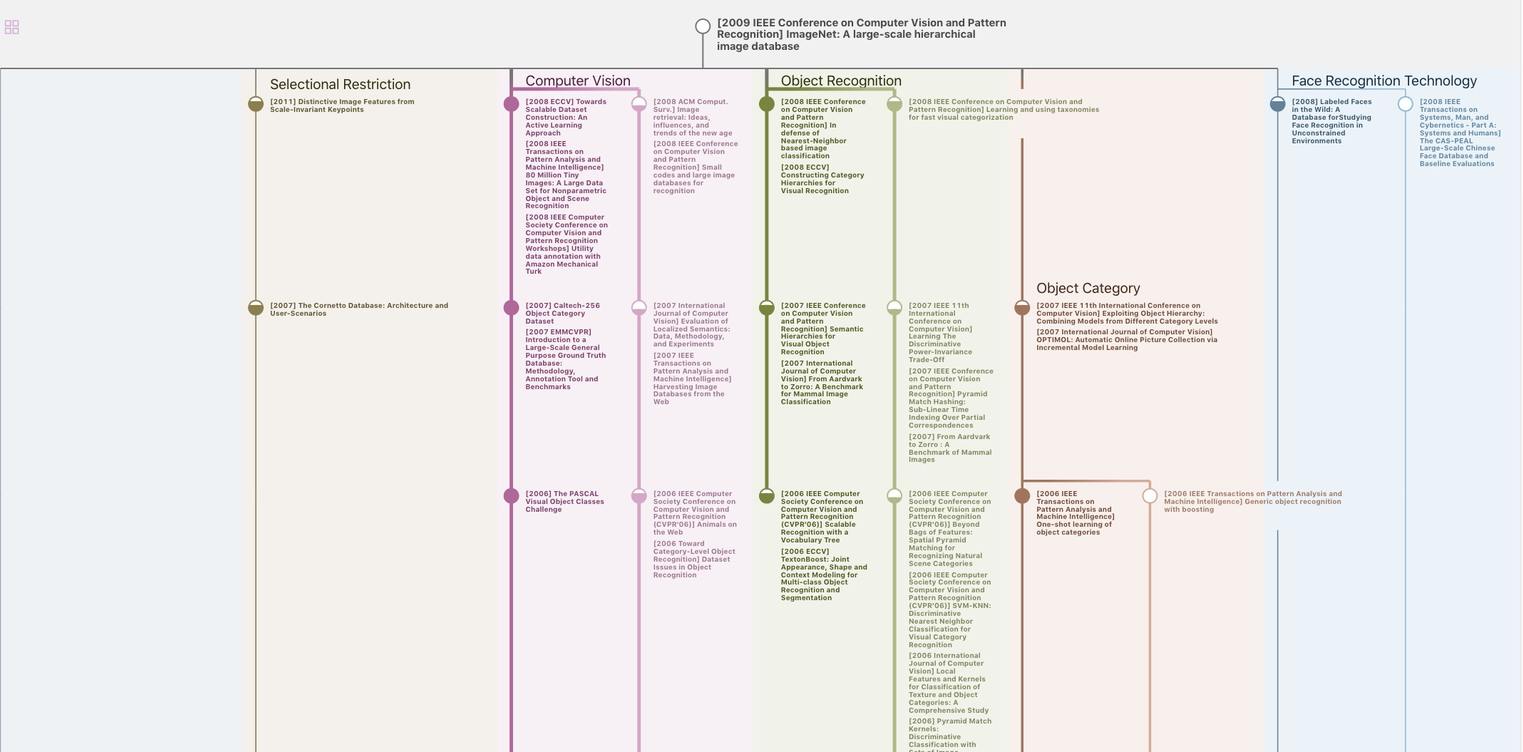
生成溯源树,研究论文发展脉络
Chat Paper
正在生成论文摘要