DMFNet: Dual-Encoder Multistage Feature Fusion Network for Infrared Small Target Detection
IEEE Transactions on Geoscience and Remote Sensing(2024)
摘要
Infrared small target detection (IRSTD) is the challenging task of identifying small targets with low signal-to-noise ratios in complex backgrounds. Traditional methods in the complex background of IRSTD lead to a large number of false alarms and misdetections. Although CNN-based methods have made progress in IRSTD, how to extract more effective information and fully utilize interlayer information remains an unresolved issue. Therefore, this article proposed a dual-encoder multistage feature fusion network (DMFNet). Specifically, we designed a dual-encoder with different inputs to capture more effective small target feature information. We then designed a receptive field expansion attention module (REAM) to incorporate nonlocal contextual information. In the decoding phase, the Triple Cross-layer Fusion Module (TCFM) was developed to exchange the low-level spatial details and the high-level semantic information for preserving more small target information in deeper layers. Finally, by concatenating multiscale features from various layers of the decoder, more discriminative feature maps were generated to clearly describe the infrared small targets. Experimental results on the NUDT-SIRST, NUAA-SIRST, and IRSTD-1k datasets demonstrated that DMFNet outperforms some other state-of-the-art methods, achieving superior detection performance. The codes are available at https://github.com/BJZHOU2000/DMFNet.
更多查看译文
关键词
Feature extraction,Object detection,Task analysis,Deep learning,Semantics,Robustness,Decoding,Decoder-encoder,feature fusion,infrared small target detection (IRSTD),multiscale feature
AI 理解论文
溯源树
样例
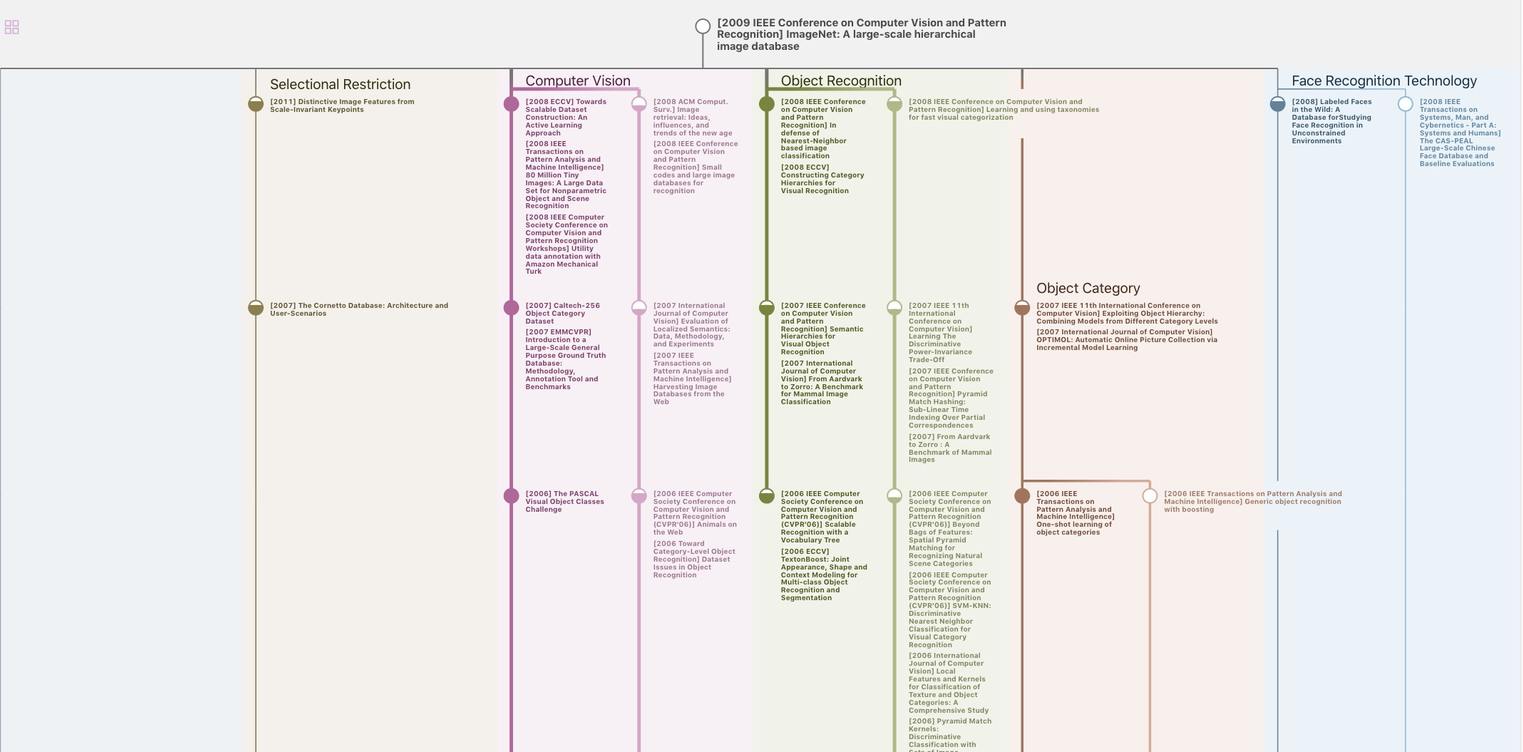
生成溯源树,研究论文发展脉络
Chat Paper
正在生成论文摘要