Enhanced Deep Reinforcement Learning for Parcel Singulation in Non-Stationary Environments.
IEEE International Conference on Acoustics, Speech, and Signal Processing(2024)
Abstract
In the rapidly expanding logistics sector, parcel singulation has emerged as a significant bottleneck. To address this, we propose an automated parcel singulator utilizing a sparse actuator array, which presents an optimal balance between cost and efficiency, albeit requiring a sophisticated control policy. In this study, we frame the parcel singulation issue as a Markov Decision Process with a variable state space dimension, addressed through a deep reinforcement learning (RL) algorithm complemented by a State Space Standardization Module (S3). Distinct from previous RL approaches, our methodology initially considers the non-stationary environment during the problem modeling phase. To counter this challenge, the S3 module standardizes the dynamic input state, thereby stabilizing the RL training process. We validate our method through simulation experiments in complex environments, comparing it with several baseline algorithms. Results indicate that our algorithm excels in parcel singulation tasks, achieving a higher success rate and enhanced efficiency.
MoreTranslated text
Key words
Reinforcement learning,Markov decision process,nonstationary environment,state space standardization,parcel singulation
AI Read Science
Must-Reading Tree
Example
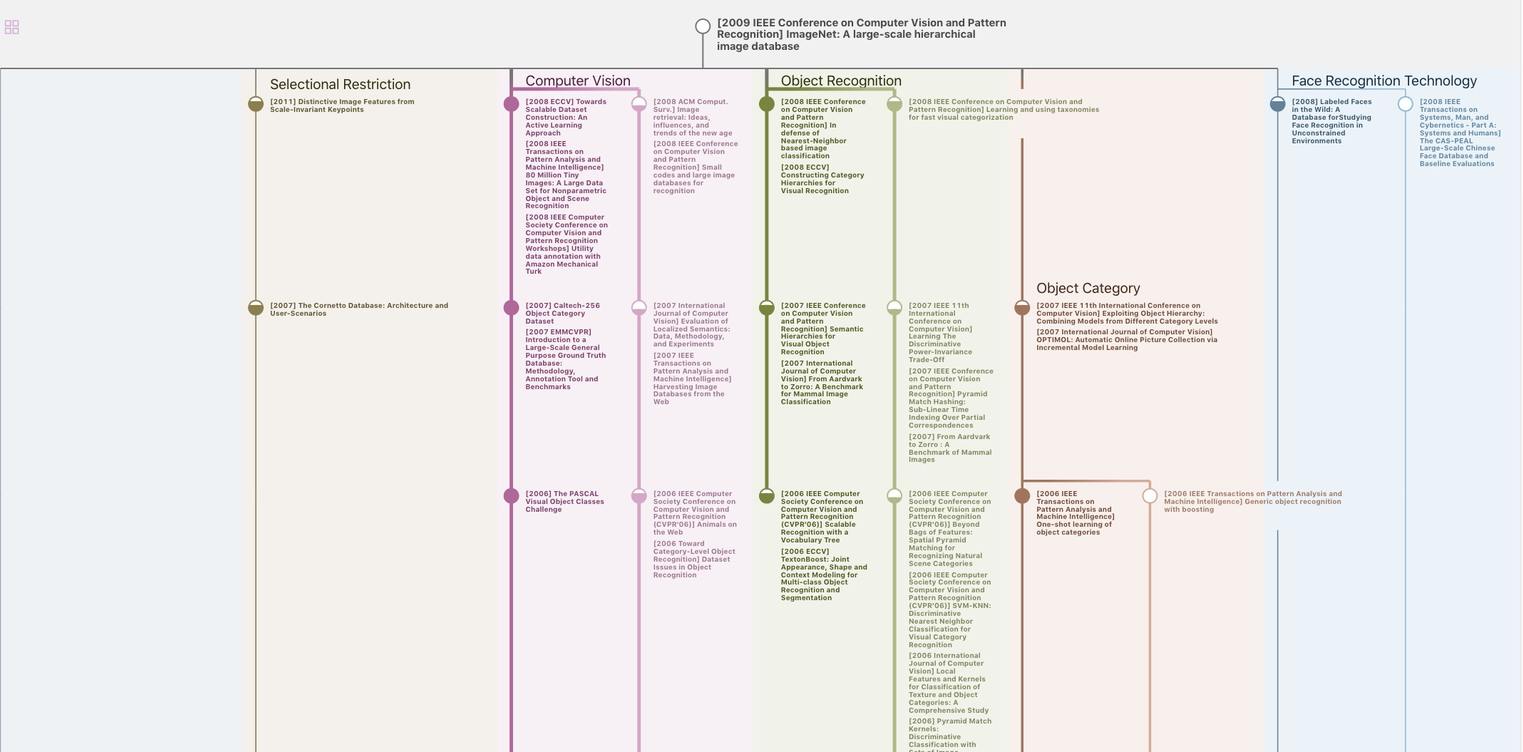
Generate MRT to find the research sequence of this paper
Chat Paper
Summary is being generated by the instructions you defined