MixUp-Based Hard Negative Sample for Self-Supervised Entity Alignment
2023 7th International Conference on Communication and Information Systems (ICCIS)(2023)
摘要
Entity alignment, aiming to discover the entities that refer to the same things in different knowledge graphs (KGs), and is a pivotal technology for the fusion of KGs, which has attracted wide attention in recent years. To apply supervised learning, we need enough labeled data. However, manually labeling data is, However, self-learning, as a new learning method, has achieved remarkable results and attracted increasing attention in representation learning in EA, such as SelfKG. Through neighborhood information aggregation, we can provide high quality negative examples in a targeted manner, speed up convergence, and let the model optimize in the direction. In this paper, we propose a new method named MSEA for negative sampling, which contains Positive Mixing strategy and Multi-head strategy. For the problem that SelfKG lacks information interaction between two KG, we propose Interactive Contrastive Learning Mechanism. Experimental results show that our approach outperforms the previous best self-supervised method by a large margin (over 4% Hits@l almost improvement on average) and performs on par with previous SOTA supervised counterparts, demonstrating the effectiveness of the interactive contrastive learning for self-supervised EA.
更多查看译文
关键词
Knowledge Graph,Entity Alignment,Self Supervised Learning,Negative Sampling. MixUp
AI 理解论文
溯源树
样例
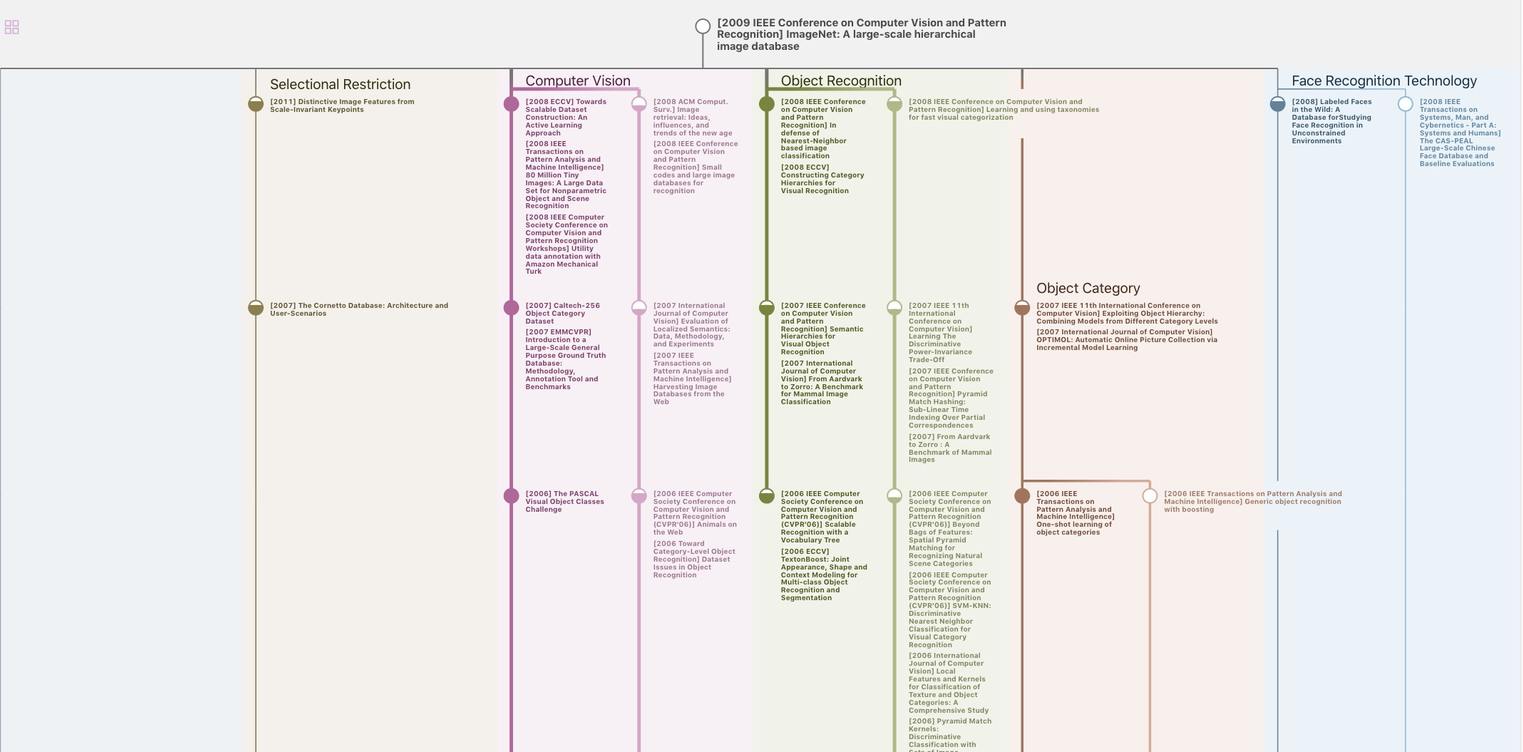
生成溯源树,研究论文发展脉络
Chat Paper
正在生成论文摘要